Claim Your Discount Today
Get 10% off on all Statistics homework at statisticshomeworkhelp.com! Whether it’s Probability, Regression Analysis, or Hypothesis Testing, our experts are ready to help you excel. Don’t miss out—grab this offer today! Our dedicated team ensures accurate solutions and timely delivery, boosting your grades and confidence. Hurry, this limited-time discount won’t last forever!
We Accept
- Simple Random Sampling
- Description and Implementation
- Advantages and Limitations (Approx. 200 words)
- Stratified Sampling
- Description and Implementation
- Advantages and Limitations
- Cluster Sampling
- Description and Implementation
- Advantages and Limitations
- Systematic Sampling
- Description and Implementation
- Advantages and Limitations
- Conclusion:
In the dynamic landscape of statistical analysis, SPSS (Statistical Package for the Social Sciences) stands as a cornerstone tool embraced by researchers and students across diverse disciplines. Renowned for its adaptability and robust features, SPSS has evolved into an indispensable asset for handling intricate datasets that characterize modern research endeavors. At the heart of proficient data analysis lies the fundamental concept of sampling, a pivotal process that underpins a myriad of statistical procedures. Sampling, in essence, involves the deliberate selection of a subset of individuals or items from a larger population, meticulously chosen to accurately represent the entirety of the population under scrutiny. Within the expansive toolkit of SPSS, a diverse array of sampling techniques awaits exploration, each meticulously designed to cater to specific research scenarios and objectives. Thus, a comprehensive understanding of these techniques emerges as a cornerstone prerequisite for researchers and students alike, serving as the compass that guides the design of studies and the derivation of meaningful conclusions. As researchers venture into the realm of data analysis, they encounter a plethora of complexities inherent in the datasets they scrutinize. These datasets, often characterized by voluminous dimensions and multifaceted variables, demand meticulous handling to extract meaningful insights effectively. It is within this intricate terrain that SPSS emerges as a stalwart ally, offering a comprehensive suite of tools tailored to navigate the intricacies of modern data analysis. With its user-friendly interface and robust analytical capabilities, SPSS empowers researchers to navigate through complex datasets with ease, unraveling intricate patterns and uncovering hidden relationships that lie dormant within the data. Moreover, SPSS's versatility transcends disciplinary boundaries, catering to the diverse needs of researchers across fields ranging from social sciences to business analytics, thereby cementing its status as a ubiquitous presence in the realm of statistical analysis.
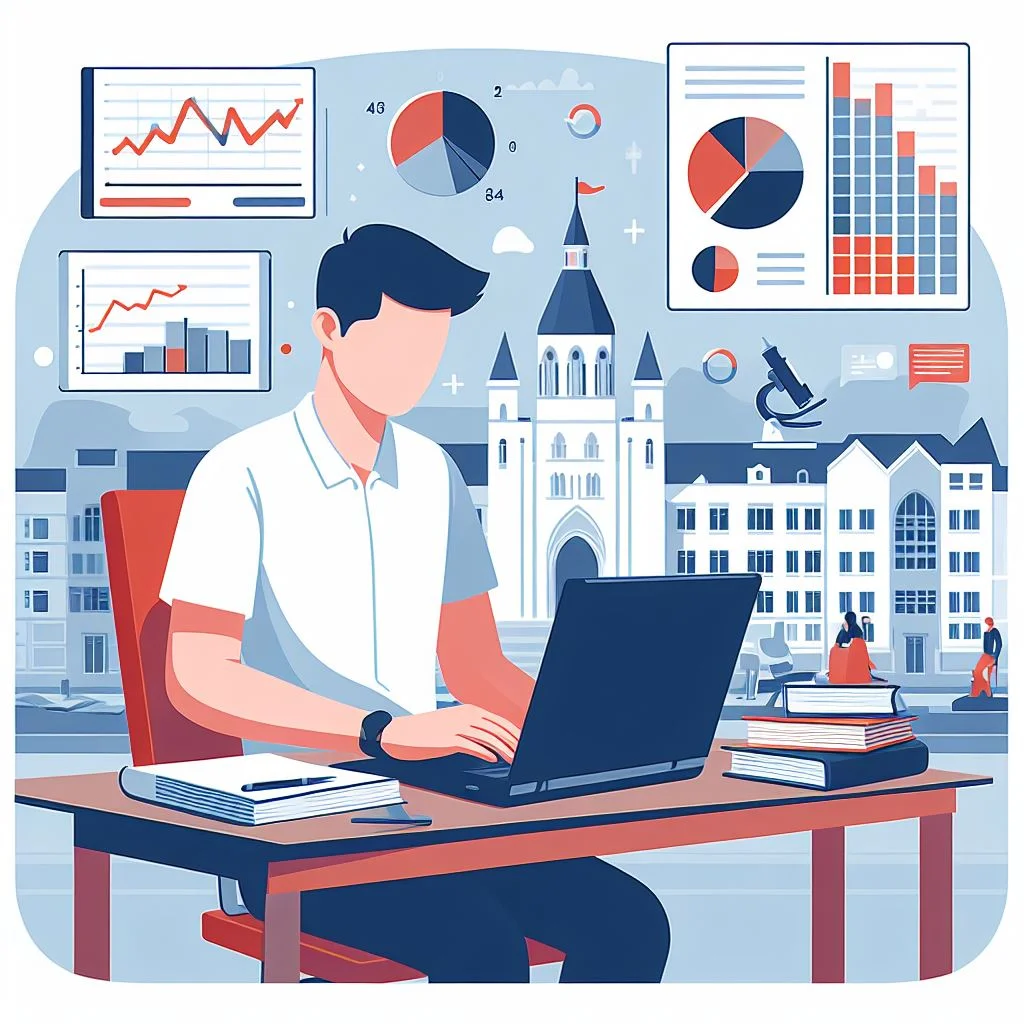
At the core of statistical analysis lies the indispensable concept of sampling, a cornerstone process that forms the bedrock of robust research methodologies. Sampling, in its essence, entails the meticulous selection of a subset of individuals or items from a larger population, meticulously chosen to accurately reflect the characteristics of the broader population. This deliberate curation of samples serves as a conduit through which researchers glean insights into the underlying dynamics of the population under scrutiny, enabling them to draw meaningful inferences and derive actionable insights. However, the efficacy of sampling hinges upon the judicious selection of appropriate sampling techniques, each tailored to cater to specific research objectives and constraints. Within the expansive repertoire of SPSS, a diverse array of sampling techniques awaits exploration, each meticulously designed to cater to a spectrum of research scenarios and objectives. From the foundational simplicity of simple random sampling to the nuanced stratification of stratified sampling, SPSS offers a comprehensive toolkit that empowers researchers to navigate through the intricacies of sampling with precision and efficacy. By equipping research students with the knowledge and proficiency to harness these advanced sampling techniques, SPSS emerges as an invaluable ally in their academic pursuits, empowering them to navigate through the complexities of data analysis with confidence and precision.
Simple Random Sampling
Simple Random Sampling (SRS) is one of the fundamental sampling techniques used in statistics to obtain a representative sample from a population. In essence, it involves selecting individuals from a population in such a way that each member of the population has an equal chance of being chosen for the sample. This ensures that the sample is unbiased and representative of the entire population, making it a cornerstone of statistical inference and hypothesis testing.
Description and Implementation
Implementing Simple Random Sampling (SRS) in SPSS involves several steps to ensure that the sample is selected randomly and without bias. Firstly, each individual in the population is assigned a unique identifier, such as an ID number or code. This unique identifier distinguishes each member of the population and facilitates the random selection process. Next, SPSS provides tools to generate random numbers, which are used to select samples from the population. Researchers can specify the desired sample size, and SPSS will randomly generate numbers corresponding to the unique identifiers of individuals in the population. These random numbers are then used to select the corresponding individuals for inclusion in the sample.
It's essential to ensure that the random number generation process is truly random to maintain the integrity of the sampling procedure. SPSS offers various options for generating random numbers, including using system time or specifying a seed value for the random number generator. Researchers should carefully select the appropriate method based on their specific research needs and ensure that the random numbers generated are truly random and independent of any external factors. Once the random numbers are generated, researchers can use them to select the corresponding individuals from the population dataset in SPSS. This process ensures that each member of the population has an equal chance of being selected for the sample, thereby preserving the unbiased nature of the sample and enabling accurate statistical analysis.
Advantages and Limitations (Approx. 200 words)
Simple Random Sampling (SRS) offers several advantages that make it a popular choice in research studies. Firstly, it is relatively easy to implement, requiring minimal technical expertise and resources. Researchers only need to assign unique identifiers to individuals in the population and use SPSS tools to generate random numbers for sample selection. Additionally, SRS ensures an unbiased sample, making it ideal for homogeneous populations where every member is equally likely to be included in the sample.
This property makes SRS particularly suitable for simple comparative studies or when the population is relatively small and well-defined. However, SRS may not be suitable for heterogeneous populations where certain subgroups are underrepresented in the sample. In such cases, stratified sampling or other more complex sampling techniques may be more appropriate to ensure representative samples from each subgroup.
Stratified Sampling
Stratified Sampling is a method used in statistical research to ensure that the sample represents specific subgroups or strata within a population. This technique involves dividing the population into homogeneous groups based on certain characteristics, such as age, gender, income level, or geographical location. Once the population is divided into these strata, samples are randomly selected from each stratum to form the final sample for analysis.
Description and Implementation
Implementing Stratified Sampling in SPSS involves utilizing the 'Complex Samples' module. This module allows researchers to specify the population strata and then select samples from each stratum. Researchers can define the variables that delineate the strata and indicate the sample size or sampling fraction for each stratum. SPSS then handles the random selection process within each stratum, ensuring that the final sample is representative of the entire population while accounting for the different characteristics present within it.
Stratified Sampling is particularly useful when the population exhibits significant heterogeneity across certain characteristics. By dividing the population into homogeneous groups or strata, researchers can ensure that each subgroup is adequately represented in the sample, thereby improving the accuracy and reliability of the research findings. This technique is commonly employed in surveys and studies where certain demographic or socio-economic factors are known to influence the research outcomes.
Advantages and Limitations
One of the primary advantages of Stratified Sampling is its ability to provide more precise estimates for each subgroup within the population. By ensuring representation from all strata, researchers can make valid inferences about specific subgroups without extrapolating findings from the overall population. This leads to more nuanced insights and enables targeted interventions or policies tailored to different segments of the population.
However, implementing Stratified Sampling in SPSS requires prior knowledge of the population characteristics that define the strata. Researchers must accurately identify relevant variables and determine appropriate stratum boundaries, which may necessitate preliminary research or data analysis. Additionally, the process of defining and sampling from multiple strata can be complex, especially for large or intricate populations, adding to the time and resources required for conducting the study.
Cluster Sampling
Cluster Sampling is a widely used technique in survey research, particularly when the target population is geographically dispersed or difficult to access individually. This method involves dividing the population into clusters or groups based on certain characteristics, such as geographical location, socioeconomic status, or organizational structure. Each cluster represents a subset of the population, and researchers randomly select entire clusters to include in the sample.
Description and Implementation
Implementing Cluster Sampling in SPSS involves several steps. First, researchers need to identify the clusters that will be used for sampling. These clusters should ideally be heterogeneous within themselves but similar to each other in terms of the characteristic being studied. For example, if the research aims to study the academic performance of students in different schools across a region, schools could be considered as clusters. Once the clusters are identified, researchers define them in SPSS using existing variables that delineate the cluster boundaries.
This could be geographical coordinates, school codes, or any other variable that uniquely identifies each cluster. SPSS provides tools and functions to define these clusters within the dataset. After defining the clusters, researchers can utilize SPSS's sampling tools to randomly select clusters from the defined population. This ensures that each cluster has an equal chance of being included in the sample, maintaining the randomness and statistical validity of the sampling process.
Advantages and Limitations
Cluster Sampling offers several advantages, making it a preferred choice in certain research scenarios. One significant advantage is its cost-effectiveness, especially when dealing with large and geographically dispersed populations. Instead of individually sampling every unit in the population, researchers can sample entire clusters, reducing the time and resources required for data collection. Furthermore, Cluster Sampling is convenient when the population is spread out over a wide area or when access to individual units is challenging. By selecting clusters, researchers can streamline the data collection process and overcome logistical barriers associated with reaching scattered populations.
However, Cluster Sampling also has limitations that researchers should consider. One notable limitation is the potential for increased sampling error due to intra-cluster homogeneity. Since clusters are often defined based on certain characteristics, such as geographical proximity or organizational affiliation, individuals within the same cluster may share similar traits or experiences. This intra-cluster homogeneity can lead to underestimation or overestimation of population parameters if not properly accounted for in the analysis. Additionally, Cluster Sampling may not be suitable for populations where clusters vary significantly from each other in terms of the characteristic being studied. In such cases, stratified sampling or other sampling techniques that account for the heterogeneity within the population may be more appropriate.
Systematic Sampling
Systematic Sampling is a method used to select a sample from a larger population in a systematic and predetermined manner. Rather than randomly selecting individuals, this technique involves choosing every nth individual from the population after a random starting point has been established. In SPSS, Systematic Sampling can be implemented efficiently using the software's sorting and selection functions.
Description and Implementation
In Systematic Sampling, the process begins by randomly selecting a starting point within the population. This starting point is crucial as it ensures the sample's randomness. Once the starting point is determined, every nth individual is selected as part of the sample. For instance, if a researcher decides to select every 5th individual from a population of 1000, they would randomly choose a starting point between 1 and 5 and then proceed to select every 5th individual thereafter.
Implementing Systematic Sampling in SPSS involves sorting the data based on a key variable and then selecting cases at regular intervals. SPSS provides tools to sort data based on specific criteria, such as demographics or numerical values. Once the data is sorted, the researcher can easily select cases at the desired intervals using SPSS's selection functions. This systematic approach ensures that the sample is representative of the population while maintaining efficiency in the sampling process.
Advantages and Limitations
Systematic Sampling offers several advantages, making it a popular choice for researchers working with large populations. One of its primary advantages is its ease of implementation. Unlike some other sampling techniques that require complex procedures, Systematic Sampling is straightforward and easy to understand. This simplicity makes it accessible to researchers with varying levels of statistical expertise. Additionally, Systematic Sampling is well-suited for large populations. By selecting every nth individual, researchers can efficiently cover a wide range of individuals within the population without having to examine every single unit. This efficiency saves time and resources, making Systematic Sampling a practical choice for studies involving extensive datasets.
However, Systematic Sampling is not without its limitations. One potential drawback is the introduction of bias if there is a periodic pattern in the data. For example, if the population exhibits a cyclical trend, selecting every nth individual could inadvertently oversample or undersample certain groups within the population. This bias can distort the results of the study and undermine the validity of the findings. Furthermore, Systematic Sampling may not be suitable for populations with unknown or irregular patterns. In such cases, a more flexible sampling technique, such as Simple Random Sampling or Stratified Sampling, may be preferred to ensure a more representative sample.
Conclusion:
Mastering advanced sampling techniques in SPSS is not merely an academic exercise but a fundamental skill that empowers research students to conduct rigorous and reliable analyses. As the cornerstone of statistical analysis, sampling techniques play a pivotal role in ensuring the validity and generalizability of research findings. By delving into the intricacies of advanced sampling methods within SPSS, students equip themselves with a versatile toolkit to navigate the complexities of real-world data and address research questions with precision.
Each advanced sampling technique in SPSS offers unique advantages and considerations, catering to diverse research objectives and data characteristics. For instance, while simple random sampling ensures unbiased representation, it may not be the most efficient choice for heterogeneous populations. On the other hand, stratified sampling allows researchers to account for population stratification, thereby enhancing the accuracy of estimates for each subgroup. Similarly, cluster sampling proves valuable for studies involving geographically dispersed populations, offering cost-effective solutions without compromising statistical integrity.