- Linear and Nonlinear Regression Analysis
- Mastering Linear Regression
- Unleashing Nonlinear Regression
- Survival Analysis for Time-to-Event Data
- Understanding the Basics
- Advanced Survival Techniques
- Structural Equation Modeling (SEM) for Complex Relationships
- Building the Foundation
- Handling Measurement Error and Latent Variables
- Bayesian Analysis with Stata
- Embracing Bayesian Paradigm
- Advanced Bayesian Techniques
- Conclusion:
Embarking on the academic journey is a thrilling yet challenging experience for students, often marked by encounters with formidable statistical assignments. The academic landscape is evolving, demanding a deeper understanding of statistical methodologies to navigate the complexities of modern datasets. From deciphering intricate data structures to implementing sophisticated models, students find themselves at the crossroads of theory and application, facing the need to master statistical techniques to succeed in their coursework. Moreover, the implementation of advanced statistical models adds another layer of complexity. Theoretical knowledge needs to be translated into practical application, necessitating proficiency in statistical software that can handle intricate analyses. Herein lies the crux of the matter: how can students bridge the gap between theoretical understanding and practical implementation?
Enter Stata, a robust statistical software that stands as a beacon of support for students navigating the statistical terrain. Stata is not merely a tool; it is an ally in the academic journey, especially when it comes to conquering the challenges posed by advanced statistical modeling. The term "advanced modeling techniques" encompasses a spectrum of methodologies that go beyond the basics of statistical analysis. Linear regression, the cornerstone of many statistical analyses, evolves into a more intricate exploration of relationships in the form of nonlinear regression. Survival analysis becomes crucial when dealing with time-to-event data, a common occurrence in fields like medicine and social sciences. Structural Equation Modeling (SEM) allows for the examination of complex relationships between variables, uncovering hidden patterns and dependencies. Bayesian analysis introduces a paradigm shift, offering a probabilistic approach to statistical inference. Stata has emerged as a go-to tool for statisticians, researchers, and students alike due to its versatility and user-friendly interface. To truly harness its potential, understanding advanced statistical modeling is key. Let's delve into four advanced techniques that can elevate your homework game. Solve your Stata homework with confidence by mastering these advanced statistical modeling techniques.
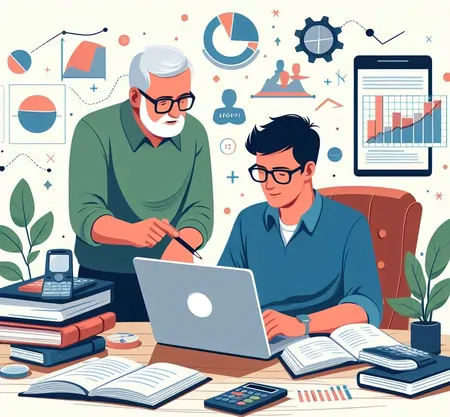
Linear and Nonlinear Regression Analysis
Linear regression serves as the cornerstone of statistical modeling, offering a robust framework for understanding relationships between variables. Stata, a powerful statistical software, simplifies the process of linear regression, making it as accessible as typing a command. However, delving into the intricacies of statistical modeling requires finesse, especially when extending beyond simple linear relationships.
Mastering Linear Regression
Linear regression, the bedrock of statistical analysis, is a fundamental technique within Stata. Initiating a simple linear regression is a matter of executing the 'regress' command, providing immediate insights into the relationship between dependent and independent variables. Yet, the real power of linear regression in Stata unfolds when you move beyond the basics. To master linear regression, begin by importing your dataset into Stata, ensuring that your variables are appropriately defined. The 'regress' command opens the gateway to exploring the nuances of multiple regression, allowing you to incorporate multiple predictors into your model. This step is crucial when dealing with real-world data where relationships are seldom simplistic.
Stata's versatility shines as you delve deeper into the analysis. Experiment with options such as robust standard errors, which account for potential heteroskedasticity and outliers, enhancing the reliability of your results. The inclusion of heteroskedasticity tests further refines your model, ensuring that it stands up to scrutiny.
Moreover, Stata's graphical capabilities add a visual dimension to your analysis. Leverage diagnostic plots to identify outliers and influential data points. These graphical aids not only enhance the interpretability of your results but also provide a deeper understanding of the underlying patterns in your data.
Unleashing Nonlinear Regression
Despite the power of linear regression, real-world phenomena often exhibit complexities that linear models cannot capture. This is where nonlinear regression steps in as a valuable tool. Stata's 'nl' command empowers analysts to break free from the constraints of linearity and model intricate relationships, such as exponential growth, logistic curves, or custom functions.
As you embark on nonlinear regression in Stata, meticulous attention to detail is crucial. Interpretation of parameters takes center stage, requiring a nuanced understanding of how each parameter influences the model. Additionally, assessing goodness-of-fit measures becomes imperative to ensure that your nonlinear model aligns with the underlying patterns in your data.
Stata's output, comprehensive and detailed, becomes your guide in this journey. The wealth of information provided allows for a thorough evaluation of model adequacy, enabling you to make informed decisions about the suitability of your nonlinear model for the given dataset. In essence, mastering both linear and nonlinear regression in Stata equips you with a powerful toolkit to unravel complex relationships in diverse datasets, providing a solid foundation for tackling advanced statistical assignments.
Survival Analysis for Time-to-Event Data
Survival Analysis, a cornerstone in statistical modeling, plays a pivotal role when examining time-to-event data, a prevalent scenario in fields such as medical research and social sciences. The essence of survival analysis lies in its ability to analyze the time until an event of interest occurs, making it particularly suitable for understanding durations until events like death, disease recurrence, or any other critical incident. In the realm of statistical software, Stata emerges as a powerful ally, offering a suite of commands specifically tailored for survival analysis.
Understanding the Basics
Survival analysis begins with recognizing its applicability in situations where traditional statistical methods fall short. Time-to-event data inherently involves the measurement of durations until a specific event unfolds. This type of data is prevalent in diverse fields, including medical studies tracking patient outcomes, sociology examining event occurrences, and epidemiology assessing the onset of diseases.
In Stata, the ‘st’ commands provide a gateway to a robust set of tools for survival analysis. The initial steps involve importing your time-to-event data into Stata and defining essential components such as survival time and event indicators. Stata's syntax simplifies the process, enabling users to focus on the interpretation of hazard ratios and the creation of visually compelling Kaplan-Meier curves. The simplicity of Stata's commands facilitates a smooth transition for users who are new to survival analysis, providing a solid foundation for delving into more advanced techniques.
Advanced Survival Techniques
As you progress in your understanding of survival analysis, Stata offers advanced capabilities that elevate your modeling strategies. One such advancement involves incorporating time-varying covariates, allowing for the examination of how variables change over the course of the study, influencing the survival outcome. Additionally, Stata facilitates stratified analyses, enabling you to explore variations in survival patterns across different subgroups. Competing risks models further enrich the analytical toolkit by considering multiple potential events that may preclude the occurrence of the primary event of interest. Stata's user-friendly syntax remains a constant, ensuring that even as you venture into more complex survival models, the software remains accessible and efficient. This adaptability is particularly advantageous when dealing with intricate datasets and nuanced survival scenarios.
In essence, Stata's prowess in survival analysis extends beyond the basics, providing researchers and students with the means to explore, interpret, and model time-to-event data comprehensively. Whether it's the fundamental Kaplan-Meier curves or the intricate considerations of advanced survival techniques, Stata empowers users to navigate the complexities of survival analysis with confidence and precision.
Structural Equation Modeling (SEM) for Complex Relationships
Structural Equation Modeling (SEM) is a powerful analytical tool that goes beyond traditional statistical methods, allowing researchers to disentangle intricate relationships between variables. In the realm of complex relationships, SEM becomes indispensable, and Stata's dedicated ‘sem’ command positions itself as a versatile and user-friendly tool for implementing this advanced technique.
Building the Foundation
At its core, Structural Equation Modeling involves creating a comprehensive framework to represent relationships among latent constructs, observed variables, and directional paths. Stata simplifies this process with its ‘sem’ command, providing a straightforward syntax to specify the elements of your SEM. To embark on this journey, start by conceptualizing your model—the latent variables and the connections between them. Once your theoretical foundation is solid, translate it into Stata code. The ‘sem’ command allows you to define latent constructs, link them with observed variables, and establish the directional paths, creating a visual representation of your model.
As you proceed, Stata's SEM capabilities empower you to refine your model iteratively. This involves paying close attention to fit indices, which assess how well your model fits the observed data, and modification indices, which suggest potential improvements. Stata's user-friendly interface ensures that researchers can easily navigate and interpret these indices, enabling them to enhance the accuracy and explanatory power of their SEM.
Handling Measurement Error and Latent Variables
However, the real-world complexity of data often introduces challenges, such as measurement error and latent variables, that necessitate additional considerations in SEM. Stata comes to the rescue with its ‘factor’ command, which facilitates the creation and integration of latent constructs into your model. This allows you to capture underlying, unobservable factors that contribute to the observed variability in your data. Moreover, Stata's advanced options, including the ‘mim’ command, gracefully handle missing data—an inevitable aspect of real-world datasets. This ensures that your SEM is robust even in the face of incomplete information.
One significant hurdle in SEM is dealing with measurement error. Stata provides tools to navigate this challenge by allowing researchers to incorporate error covariances into their models. This not only acknowledges the imprecision in measurement but also enhances the accuracy of the overall SEM. To further refine your model and address potential issues introduced by measurement error and latent variables, Stata offers diagnostic tools. These tools empower researchers to assess the goodness of fit, identify areas for improvement, and make informed decisions to enhance the overall quality of their SEM.
Bayesian Analysis with Stata
Bayesian Analysis stands as a revolutionary paradigm in statistical modeling, challenging the traditional approach of relying solely on point estimates. Stata, a versatile statistical software, takes a giant leap forward in facilitating Bayesian methods seamlessly through its dedicated suite of commands.
Embracing Bayesian Paradigm
Embracing the Bayesian paradigm marks a departure from the deterministic world of point estimates. Bayesian Analysis introduces a probabilistic framework, emphasizing the entire probability distribution of parameters rather than a single fixed value. This shift brings a wealth of advantages, allowing researchers to incorporate prior knowledge, update beliefs with new data, and quantify uncertainty comprehensively. In the realm of Stata, this transition is made remarkably accessible. The bayes commands within Stata's arsenal streamline the process of delving into Bayesian methods. Researchers can effortlessly specify their prior beliefs about parameters, initiate chains of iterations to explore the posterior distribution, and extract credible intervals—all within the familiar Stata environment.
Stata's bayes commands provide a user-friendly interface for those venturing into Bayesian Analysis for the first time. The syntax is intuitive, making it easy to grasp the fundamental concepts of Bayesian modeling. As researchers input their prior beliefs, define likelihood functions, and set up the structure of their Bayesian models, Stata's real-time feedback and output visualization become invaluable aids in the learning process.
Advanced Bayesian Techniques
Moving beyond the basics, Stata's integration of advanced Bayesian techniques elevates the software to a sophisticated tool for seasoned statisticians. Hierarchical models, a cornerstone of complex statistical structures, can be effortlessly implemented using Stata's bayes commands. This allows researchers to model variability at multiple levels, providing a nuanced understanding of the data generation process. Bayesian model averaging, another advanced technique, enables the consideration of multiple models and their associated parameters simultaneously. Stata's implementation of this approach ensures a robust analysis that accounts for model uncertainty, a crucial aspect often overlooked in traditional statistical approaches.
Convergence diagnostics, a critical step in Bayesian Analysis, become a seamless part of the modeling process in Stata. Researchers can monitor the stability and convergence of their Bayesian chains, ensuring the reliability of their results. Stata's integration of these diagnostics simplifies the often complex task of assessing whether the Markov chains have reached a stationary distribution, a key aspect of Bayesian modeling.
Conclusion:
In conclusion, venturing into the multifaceted realm of statistical modeling becomes an enlightening journey with Stata as a steadfast companion. The software's versatility shines through as it seamlessly accommodates a spectrum of advanced techniques, ranging from the foundational linear and nonlinear regression to the nuanced realms of survival analysis, structural equation modeling, and Bayesian analysis. Stata's prowess extends beyond being a mere statistical tool; it transforms into a formidable ally, offering a rich tapestry of features that cater to the diverse needs of researchers and students.
Mastering these advanced techniques within the Stata environment is not just about acing homework assignments; it is a gateway to cultivating a robust and adaptable skill set. As you delve into the intricacies of modeling, you not only decipher complex datasets but also gain a profound understanding of the underlying statistical principles. This skill set transcends the academic realm, laying a solid foundation for a successful professional journey.