- Understanding the Basics of Data and Research Objectives in Statistical Testing
- Data Types: Categorical and Numerical
- Research Objectives: Navigating Your Statistical Journey
- Unveiling the Role of Research Objectives
- The Purpose-Driven Selection of Statistical Tests
- Navigating through Examples
- The Gateway to Insightful Analysis
- Formulating Research Objectives
- The Hypotheses in Focus
- The Power of Hypotheses in Action
- The Role of Hypotheses in Statistical Testing
- The Dance of Evidence and Inference
- Steps to Choose the Right Statistical Test
- Common Types of Statistical Tests
- 1. Comparing Two Groups:
- 2. Comparing Multiple Groups:
- 3. Relationship Between Two Variables:
- 4. Predictive Analysis:
- 5. Comparing Proportions:
- 6. Paired Data:
- Tools and Software
- Conclusion
In the world of research and data analysis, statistical tests are the backbone of drawing meaningful conclusions from data. Selecting the right statistical test is crucial for obtaining accurate results and making informed decisions based on your data, especially when it's time to take your statistical test. In this blog post, we will guide you through the process of choosing the right statistical test for your assignment.
Understanding the Basics of Data and Research Objectives in Statistical Testing
Statistical testing forms the bedrock of scientific research and data analysis. It empowers us to draw meaningful insights from data, transforming raw information into actionable knowledge. Before delving into the intricate world of statistical tests, it's imperative to establish a firm grasp of some fundamental concepts that lay the groundwork for effective decision-making.
Data Types: Categorical and Numerical
The type of data you're working with serves as a compass guiding you toward the appropriate statistical test. Data is broadly classified into two categories: categorical and numerical.
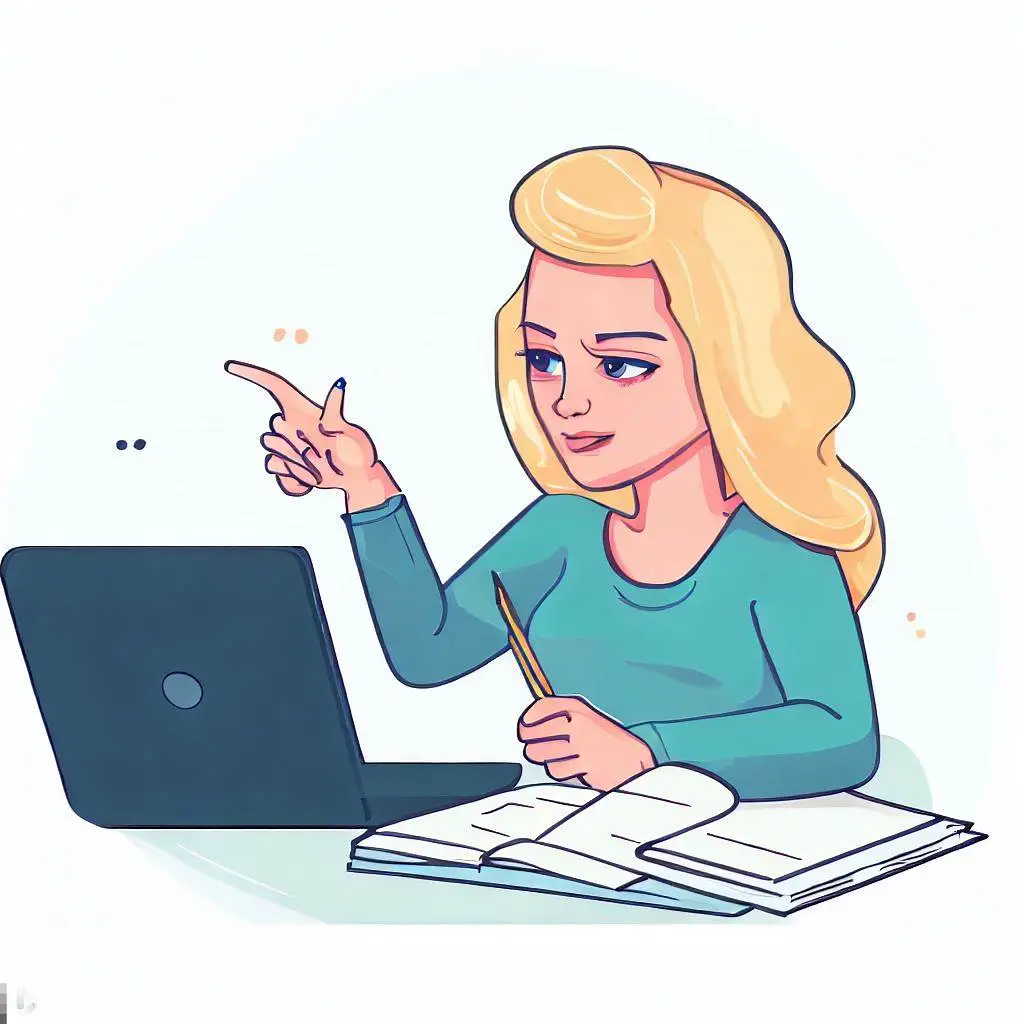
- Categorical Data: This type of data is characterized by its division into distinct categories or groups. However, these categories lack any inherent order or magnitude relationship. Examples of categorical data include gender (male/female), color preferences (red/blue/green), and yes/no responses to survey questions. Categorical data is best described using frequencies and percentages, as the data points do not possess numerical value interpretations.
- Numerical Data: Numerical data, as the name suggests, consists of numerical values. It can be further subdivided into two subtypes:
- Continuous Data: Continuous data is characterized by its ability to take on any numerical value within a specified range. Examples include height, weight, temperature readings, and time measurements. Continuous data can be measured with great precision due to its inherent nature.
- Discrete Data: Discrete data, on the other hand, comprises distinct and specific numerical values. These values are often counted rather than measured. Examples include the number of siblings, the number of cars in a parking lot, and the count of customer complaints. Discrete data is generally reported as whole numbers and is limited to specific, separate values.
Research Objectives: Navigating Your Statistical Journey
Imagine embarking on a journey through a dense forest. As you set out, you need a clear destination in mind to choose the right path and resources. Similarly, in the realm of statistical testing, your research objectives are your guiding stars, illuminating the way to the most appropriate statistical test. Let's delve into the significance of well-defined research objectives and their pivotal role in shaping your statistical voyage.
Unveiling the Role of Research Objectives
Research objectives are the compass that guides your statistical expedition. They offer a panoramic view of your research landscape, helping you decipher the route to take and the tools to employ. Just as a skilled navigator consults maps before setting sail, a researcher crafts clear research objectives before diving into data analysis.
The Purpose-Driven Selection of Statistical Tests
Your research objectives serve as the North Star in the vast cosmos of statistical tests. Each test is tailored to answer specific questions, making the alignment between your objectives and the chosen test paramount. Whether you're comparing means, investigating relationships, or testing differences, the type of question you're aiming to answer will dictate your choice.
Navigating through Examples
Consider a scenario where you're examining the effects of a new fitness program on weight loss. Your research objectives play a pivotal role in selecting the right statistical approach:
- Comparing Means: If your goal is to determine whether the mean weight loss in the group following the new fitness program is significantly different from a control group's mean weight loss, you're embarking on a journey of comparing means. In this case, a two-sample t-test or analysis of variance (ANOVA) might be appropriate.
- Assessing Relationships: On the other hand, if your aim is to explore the connection between the amount of time participants spend exercising and the magnitude of weight loss achieved, you're delving into assessing relationships. This journey might lead you to correlation analysis, where you measure the strength and direction of the linear relationship between two numerical variables.
The Gateway to Insightful Analysis
Research objectives are more than just signposts; they are the gateway to profound insights. By clearly defining what you intend to explore or discover, you enable yourself to extract meaningful conclusions from your data. A vague or poorly defined research objective is akin to setting off on a journey with an unclear destination – you might end up wandering aimlessly, unable to draw valuable conclusions.
Formulating Research Objectives
Crafting research objectives is an art that requires precision and clarity. Start by posing concise questions that encapsulate your research aims. Are you seeking to unveil patterns, determine differences, or unravel relationships? Your objectives should be specific, measurable, achievable, relevant, and time-bound (SMART) – a framework borrowed from project management that's equally applicable to research.
Imagine you're an explorer, embarking on a journey to unravel a hidden treasure. As you set foot on uncharted terrain, you're armed with two assumptions: that the treasure exists (your alternative hypothesis) and that it might not (your null hypothesis). In the realm of statistical analysis, formulating hypotheses is akin to setting the stage for this exploration – it's where your journey begins.
The Hypotheses in Focus
Hypotheses are the pillars upon which the edifice of scientific inquiry stands. They are statements that encapsulate the fundamental assumptions you're testing through your data analysis. These statements provide the direction and purpose for your investigation, shaping every aspect of your study.
In the statistical context, hypotheses come in pairs: the null hypothesis (H0) and the alternative hypothesis (H1 or Ha).
- Null Hypothesis (H0): This hypothesis serves as the baseline assumption. It states that there is no significant effect, relationship, or difference in the variables under investigation. In essence, it embodies the concept that any observed differences or patterns are mere results of random chance.
- Alternative Hypothesis (H1 or Ha): The alternative hypothesis contradicts the null hypothesis. It suggests that there is a specific effect, relationship, or difference that goes beyond random variation. In other words, it posits that something significant is happening in your data.
The Power of Hypotheses in Action
Let's bring this to life with an example. Imagine you're testing a new drug's effectiveness in reducing blood pressure. Your hypotheses would look something like this:
- Null Hypothesis (H0): The new drug has no effect on reducing blood pressure.
- Alternative Hypothesis (H1): The new drug does have a significant effect on reducing blood pressure.
Here, the null hypothesis assumes that any observed changes in blood pressure are due to chance or factors other than the drug. The alternative hypothesis, on the other hand, posits that the drug is indeed influencing blood pressure.
The Role of Hypotheses in Statistical Testing
Hypotheses aren't mere words; they are the backbone of your statistical testing. They lay the groundwork for evaluating your data and drawing conclusions. Your statistical analysis revolves around determining whether your data provides enough evidence to either reject or fail to reject the null hypothesis.
Using our drug example, if your data analysis reveals a significant reduction in blood pressure among the participants who received the drug, you might conclude that there is enough evidence to reject the null hypothesis in favor of the alternative hypothesis. This suggests that the drug does indeed have a notable effect on blood pressure.
The Dance of Evidence and Inference
Statistical testing is like a court trial, where evidence is presented to either convict or acquit the defendant (in this case, the null hypothesis). The evidence takes the form of data, and the verdict determines whether you accept or reject the null hypothesis.
In your journey of statistical exploration, you'll employ various statistical tests to gather this evidence. The results will guide your decision-making and determine whether your data supports or contradicts your initial assumptions.
Steps to Choose the Right Statistical Test
- Identify Your Data Types: The first step is to determine the type of data you have – categorical or numerical. This will narrow down the list of applicable statistical tests.
- Number of Groups or Variables: Determine how many groups or variables you have. For example, are you comparing two groups, multiple groups, or variables against each other?
- Dependent and Independent Variables: Identify your dependent and independent variables. The dependent variable is the outcome you're measuring, while the independent variable is the one you're manipulating or examining for its effect.
- Distribution of Data: Consider the distribution of your data. Is it normally distributed, skewed, or does it follow a different pattern?
- Sample Size: Take note of your sample size. Some tests require larger sample sizes to produce reliable results.
- Assumptions: Understand the assumptions associated with each statistical test. These assumptions might relate to the distribution of data, homogeneity of variances, and more.
- Type of Comparison: Determine whether you're making a one-sample comparison, two-sample comparison, paired comparison, or multiple comparisons.
- Type of Relationship: If you're examining relationships between variables, consider whether it's a correlation, regression, or another type of relationship.
- Nature of Data Comparison: Decide whether you're testing for means, proportions, variances, or other specific attributes of the data.
Common Types of Statistical Tests
Let's explore some common scenarios and the corresponding statistical tests:
1. Comparing Two Groups:
- Independent Samples, Normally Distributed: Use the t-test (Student's t-test or Welch's t-test if variances are unequal).
- Independent Samples, Non-Normally Distributed: Consider the Mann-Whitney U test (non-parametric alternative to the t-test).
2. Comparing Multiple Groups:
- Normally Distributed Data: Employ ANOVA (Analysis of Variance) followed by post-hoc tests for pairwise comparisons.
- Non-Normally Distributed Data: Opt for the Kruskal-Wallis test (non-parametric alternative to ANOVA).
3. Relationship Between Two Variables:
- Correlation: Measure the strength and direction of the linear relationship between two continuous variables using Pearson's correlation coefficient.
- Nonlinear Relationship: Use Spearman's rank correlation for non-parametric correlation between variables.
4. Predictive Analysis:
- Linear Regression: When you want to predict a continuous outcome variable based on one or more predictor variables.
5. Comparing Proportions:
- Two Independent Proportions: Employ the Chi-Square test or Fisher's Exact test for small sample sizes.
6. Paired Data:
- Paired Samples, Normally Distributed: Use a paired t-test.
- Paired Samples, Non-Normally Distributed: Consider a Wilcoxon signed-rank test.
Tools and Software
Several tools and software make the process of selecting a statistical test easier:
- Online Wizards: Websites like GraphPad's QuickCalcs and Laerd Statistics offer step-by-step guides to choosing the right statistical test.
- Statistical Software: Software like R, Python (with libraries like SciPy and StatsModels), and SPSS provide a wide range of statistical tests with detailed documentation.
Conclusion
Choosing the right statistical test for your assignment is a critical step in ensuring the validity and reliability of your results. By understanding your data, research objectives, and the characteristics of different statistical tests, you can confidently make an informed decision. Remember, there's no one-size-fits-all approach, and careful consideration of your data's characteristics will lead you to the most appropriate statistical analysis. Whether you're comparing groups, examining relationships, or making predictions, the right statistical test will illuminate the insights hidden within your data.