- Understanding Hypothesis Testing
- Strategy 1: Clearly Define Your Hypotheses
- Strategy 2: Choose the Right Test for Your Data
- Strategy 3: Ensure Proper Sample Size
- Strategy 4: Check Assumptions
- Strategy 5: Interpret Results in Context
- Strategy 6: Avoid Common Pitfalls
- Practical Example
- 1. Formulate Hypotheses:
- Conclusion
Hypothesis testing is a fundamental aspect of statistics, crucial for drawing meaningful conclusions from data. Whether you’re a student tackling your statistics homework or a researcher analyzing experimental results, understanding the process of hypothesis testing can significantly enhance your analytical skills. This guide will provide you with effective strategies for conducting hypothesis tests, ensuring you approach your statistical problems with confidence and precision.
Understanding Hypothesis Testing
Before diving into strategies, let's briefly recap what hypothesis testing involves. Hypothesis testing is a statistical method used to make inferences about a population parameter based on a sample. The process typically involves the following steps:
- Formulate the Hypotheses: Establish the null hypothesis ((H_0)) and the alternative hypothesis ((H_a)).
- Select the Significance Level ((\alpha)): Choose the probability of rejecting the null hypothesis when it is true.
- Choose the Appropriate Test: Depending on the data and the hypotheses, select the appropriate statistical test.
- Calculate the Test Statistic: Use the sample data to calculate the test statistic.
- Determine the p-value or Critical Value: Compare the test statistic to the critical value or use the p-value to decide whether to reject the null hypothesis.
- Draw Conclusions: Based on the comparison, decide whether to reject or fail to reject the null hypothesis.
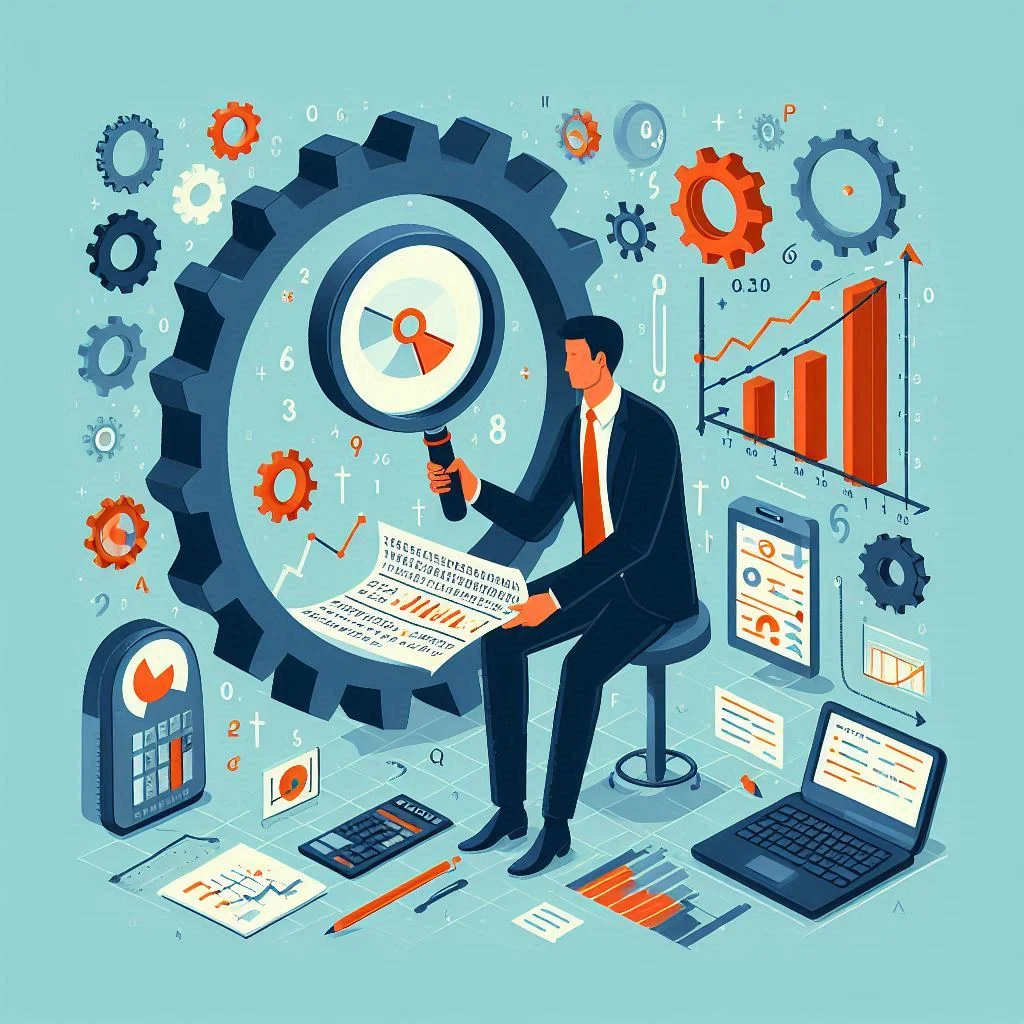
Strategy 1: Clearly Define Your Hypotheses
The first step in hypothesis testing is to clearly define your null and alternative hypotheses. The null hypothesis ((H_0)) typically represents the status quo or a statement of no effect, while the alternative hypothesis ((H_a)) represents the effect or difference you aim to detect. For instance, if you are testing whether a new drug is more effective than the existing one, (H_0) could be "the new drug has no effect" and (H_a) could be "the new drug is more effective."
Strategy 2: Choose the Right Test for Your Data
Selecting the appropriate statistical test is crucial. The choice depends on several factors, including the type of data, sample size, and whether the population variance is known. Common tests include:
- Z-test: Used when the population variance is known and the sample size is large.
- T-test: Used when the population variance is unknown and the sample size is small.
- Chi-square test: Used for categorical data to assess how likely it is that an observed distribution is due to chance.
- ANOVA (Analysis of Variance): Used to compare the means of three or more samples.
Strategy 3: Ensure Proper Sample Size
A proper sample size is essential for the reliability of your hypothesis test. Too small a sample size may lead to inconclusive results, while too large a sample size may make even trivial differences appear significant. Use power analysis to determine the appropriate sample size for your study, ensuring that it is neither too small nor unnecessarily large.
Strategy 4: Check Assumptions
Every statistical test comes with its assumptions. For instance, the t-test assumes that the data are normally distributed and that the samples have equal variances. Always check these assumptions before proceeding with the test. If the assumptions are violated, consider using a different test or transforming your data.
Strategy 5: Interpret Results in Context
Interpreting the results of a hypothesis test goes beyond just looking at the p-value. Consider the practical significance of your findings. A statistically significant result does not always imply a practically important effect. Additionally, consider the confidence interval, which provides a range of values within which the true population parameter is likely to lie.
Strategy 6: Avoid Common Pitfalls
Be aware of common pitfalls in hypothesis testing:
- P-hacking: Avoid manipulating your data or performing multiple tests just to achieve significant results.
- Ignoring Effect Size: Always report the effect size to provide a sense of the magnitude of the observed effect.
- Overreliance on P-values: A p-value is not the probability that the null hypothesis is true. It merely indicates the strength of the evidence against the null hypothesis.
Practical Example
Let’s consider a practical example to illustrate these strategies. Suppose we want to test whether a new teaching method improves student performance compared to the traditional method.
1. Formulate Hypotheses:
(H_0): The new teaching method has no effect on student performance.
(H_a): The new teaching method improves student performance.
- Select Significance Level: Choose (\alpha = 0.05).
- Choose the Test: If we have a small sample size and do not know the population variance, a t-test is appropriate.
- Calculate the Test Statistic: Use sample data to calculate the t-value.
- Determine p-value or Critical Value: Compare the calculated t-value to the critical value from the t-distribution table.
- Draw Conclusions: If the p-value is less than 0.05, we reject (H_0), concluding that the new teaching method improves student performance.
Conclusion
Hypothesis testing is a powerful tool for making inferences about populations based on sample data. By clearly defining hypotheses, choosing the right test, ensuring proper sample size, checking assumptions, interpreting results in context, and avoiding common pitfalls, you can effectively conduct hypothesis tests and draw meaningful conclusions from your statistical analyses. Whether you’re working on homework assignments or conducting research, these strategies will enhance your ability to perform accurate and reliable hypothesis tests.