- Exploratory Data Analysis
- Descriptive Statistics
- Data Visualization Techniques
- Hypothesis Testing in Ecological Research
- Formulating Hypotheses
- Statistical Tests for Ecological Data
- Regression Analysis in Ecological Modeling
- Linear Regression
- Non-linear Regression and Advanced Techniques
- Bayesian Statistics in Ecological Inference
- Basics of Bayesian Inference
- Bayesian Approaches in Ecological Modeling
- Conclusion
Ecological research stands at the intersection of environmental science and statistical analysis, requiring a nuanced understanding of the intricate relationships between various ecological variables. Within this dynamic field, the significance of environmental statistics cannot be overstated. It serves as the bedrock upon which researchers build their interpretations, draw conclusions, and contribute to the broader understanding of ecosystems. As students embark on assignments geared towards navigating data for ecological research, they are thrust into the heart of this intricate interplay between nature and numbers. The complexity of ecological systems necessitates a robust understanding of statistical techniques. It is not merely about crunching numbers but deciphering the language of nature encoded within the data. This is where the journey begins for students – in unraveling the intricacies of environmental statistics. The term "environmental statistics" itself implies a specialization that goes beyond conventional statistical applications. It involves the integration of statistical methods with the unique challenges posed by environmental data, which often spans diverse and interconnected variables. Whether you're seeking assistance with your statistics homework or diving into the realm of environmental statistics, this journey promises to deepen your understanding of statistical analysis in the context of ecological research. The primary objective of this blog is to be a guiding light for students navigating the terrain of environmental statistics within the context of their homework assignments. To navigate this terrain successfully, students must transcend basic statistical knowledge. They need a specialized skill set that allows them to not only manipulate data but to extract meaningful patterns and insights from the complex tapestry of ecological information.
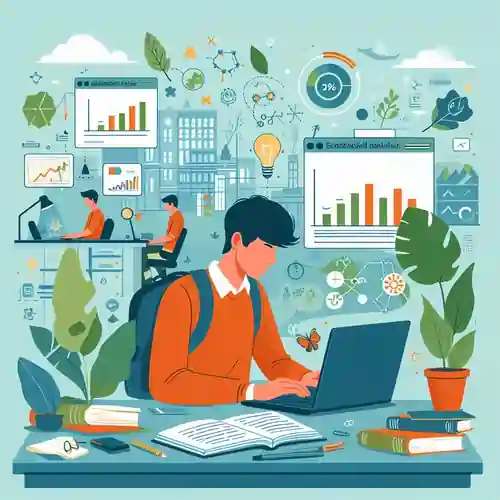
Ecological datasets, by their very nature, are vast and multifaceted. They encapsulate a myriad of environmental variables, each influencing and interacting with the others in intricate ways. The first challenge for students is to grapple with the sheer volume and diversity of data. Environmental statistics acts as a compass, providing the necessary tools to navigate through this sea of information. It equips students with the ability to discern patterns, identify trends, and extract valuable knowledge that contributes to the understanding of ecological systems.
Exploratory Data Analysis
Exploratory Data Analysis (EDA) stands as the cornerstone of ecological research, providing researchers with the initial tools to unravel the complexities inherent in environmental datasets. As students embark on their ecological research assignments, understanding the nuances of EDA becomes paramount for navigating and interpreting vast and intricate data sets.
Descriptive Statistics
Before researchers immerse themselves in the intricacies of ecological datasets, they must first embrace the fundamentals of descriptive statistics. This foundational step involves gaining insights into the main features of a dataset, offering a snapshot that serves as a precursor to deeper analysis. Descriptive statistics encapsulate essential parameters, including central tendency, variability, and distribution. Among these, the mean, median, and standard deviation emerge as indispensable tools in the researcher's arsenal. The mean, or average, provides a measure of central tendency, pinpointing the typical value within the dataset. The median, positioned at the middle of the dataset when arranged in ascending order, offers a robust alternative, especially in the presence of outliers. Standard deviation, on the other hand, gauges the extent of variability within the dataset, elucidating the spread of values around the mean.
In ecological research, where datasets are often characterized by diverse and dynamic variables, these descriptive statistics serve as navigational guides. They pave the way for a nuanced understanding of the data's structure, enabling researchers to discern patterns and trends that may influence ecological phenomena.
Data Visualization Techniques
Armed with a grasp of descriptive statistics, the journey into navigating environmental data advances to the realm of visualization techniques. While descriptive statistics offer numerical insights, visualization brings the data to life, making it more accessible and comprehensible. Visualization not only aids in conveying complex information but also serves as a powerful means for pattern recognition. Ecological data, with its multidimensional nature, often conceals valuable insights within its numerical labyrinth. Visualization tools such as scatter plots, histograms, and box plots become the researcher's brush, painting a vivid picture of the data landscape.
Scatter plots, with their ability to showcase relationships between two variables, become invaluable in identifying correlations. Histograms, on the other hand, provide a visual representation of the data distribution, offering a clear depiction of frequency and density. Box plots reveal the central tendency, variability, and potential outliers, providing a holistic view of the dataset's characteristics. For students grappling with the intricacies of ecological research, mastering these visualization techniques is transformative. It empowers them to not only interpret their data more effectively but also to communicate their findings persuasively. Visualization becomes a bridge between the raw numbers and meaningful ecological narratives, enhancing the overall impact of their research endeavors.
Hypothesis Testing in Ecological Research
Ecological research is a dynamic field that often requires scientists to draw inferences and make decisions based on observed data. Hypothesis testing serves as a fundamental tool in this process, providing a structured framework for researchers to evaluate their findings statistically. As students progress in their ecological research assignments, the significance of hypothesis testing becomes increasingly apparent.
Formulating Hypotheses
At the heart of hypothesis testing lies the formulation of clear and testable hypotheses. Hypotheses act as the cornerstone of statistical analysis, serving as the guiding principles that shape the researcher's approach. When formulating hypotheses, students must articulate a clear statement about the expected relationship or effect within their ecological research. This process demands precision and clarity to ensure that the subsequent statistical tests align with the research questions at hand. In ecological research, hypotheses often revolve around relationships between variables or differences between groups. For example, a hypothesis might assert that an increase in temperature leads to changes in the population dynamics of a particular species. Another hypothesis might propose a difference in biodiversity between two distinct habitats.
These hypotheses set the stage for statistical analyses that will ultimately validate or refute the proposed assertions. Understanding the nuances of hypothesis formulation is crucial for extracting meaningful insights from environmental statistics. A well-crafted hypothesis not only facilitates focused research but also ensures that the subsequent statistical tests are appropriate for addressing the specific research questions posed.
Statistical Tests for Ecological Data
Once hypotheses are formulated, students need to navigate the diverse landscape of statistical tests available for ecological data. Ecological research often involves the analysis of complex datasets with multiple variables, necessitating a thoughtful selection of statistical tests. This stage requires students to delve into the intricacies of different tests and understand their applications in specific research scenarios.
The toolkit for ecological statistical tests is broad and varied. Students may encounter scenarios where t-tests are suitable for comparing means between two groups, or where chi-square tests become instrumental in assessing associations between categorical variables. Beyond these, there are multivariate analyses, regression models, and non-parametric tests, each with its unique strengths and applications. Selecting the right statistical test is pivotal for drawing accurate conclusions from ecological data.
Regression Analysis in Ecological Modeling
Regression analysis stands as a cornerstone in ecological modeling, a powerful tool that empowers researchers to unravel the intricate relationships between variables within complex ecological systems. Among the various forms of regression analysis, linear regression takes a prominent place, frequently serving as the initial step for understanding how changes in one variable correspond to changes in another.
Linear Regression
In ecological research, linear regression proves indispensable due to its simplicity and interpretability. This statistical method is particularly valuable when attempting to establish a linear relationship between two variables, where a change in the independent variable is expected to produce a proportional change in the dependent variable. For instance, researchers might use linear regression to explore the correlation between temperature and the rate of plant growth or the relationship between pollution levels and biodiversity. The process of conducting a linear regression analysis involves fitting a line to the scatterplot of data points, aiming to minimize the difference between the observed values and the values predicted by the regression line. This line is characterized by a slope and an intercept, providing quantitative insights into the strength and direction of the relationship.
Understanding how to interpret these coefficients is crucial for drawing meaningful conclusions from the analysis. Linear regression in ecological modeling allows researchers to make predictions based on observed data, providing a valuable tool for forecasting ecological trends or estimating the impact of environmental changes. Moreover, it aids in identifying outliers and influential data points, contributing to a more nuanced understanding of the underlying ecological processes.
Non-linear Regression and Advanced Techniques
While linear regression is a valuable starting point, ecological systems often exhibit non-linear relationships that demand more sophisticated analyses. Non-linear regression, an advanced technique, steps in to address the complexities that linear models may overlook. In situations where the relationship between variables follows a curve or another non-linear pattern, non-linear regression allows for a more accurate representation of the underlying dynamics. Ecological research frequently encounters scenarios where simple linear relationships fail to capture the full complexity of the system. Non-linear regression models can accommodate exponential, logarithmic, polynomial, or sigmoidal relationships, offering a more flexible approach to modeling ecological data. This flexibility is vital when dealing with ecological variables that do not conform to linear assumptions.
Moreover, advanced techniques such as generalized additive models (GAMs) and machine learning algorithms like random forests or support vector machines extend the researcher's toolkit. These methods excel in capturing intricate relationships and interactions within ecological datasets, providing a more nuanced understanding of ecosystem dynamics. Students exploring regression analysis in ecological modeling will gain not only a theoretical foundation but also practical insights into the application of these techniques. The ability to discern when linear regression suffices and when to employ advanced methods equips researchers with the skills needed to navigate the diverse challenges presented by ecological datasets.
Bayesian Statistics in Ecological Inference
Ecological research, with its inherent complexities and uncertainties, often requires sophisticated statistical methods to extract meaningful insights from data. One such method gaining prominence in recent years is Bayesian statistics. This section delves into the realm of Bayesian statistics, shedding light on its fundamental principles and practical applications in ecological inference.
Basics of Bayesian Inference
In the dynamic landscape of ecological research, Bayesian statistics has emerged as a powerful and adaptive tool, revolutionizing the way researchers make inferences from limited data. At its core, Bayesian inference represents a paradigm shift from classical frequentist statistics, offering a unique approach to handling uncertainty and incorporating prior knowledge into statistical analyses. Bayesian inference begins with the formulation of a prior probability distribution, representing the initial beliefs or knowledge about a parameter before observing any data. This prior is then updated based on observed data using Bayes' theorem, yielding a posterior distribution that reflects the refined understanding of the parameter in light of the new information. This iterative process of updating beliefs makes Bayesian inference particularly suited for scenarios where data are scarce or challenging to obtain.
The advantages of Bayesian inference become evident when dealing with complex ecological systems, where uncertainty is inherent. Unlike frequentist methods that provide point estimates and confidence intervals, Bayesian analyses produce probability distributions, offering a more nuanced understanding of the range of possible parameter values. This probabilistic approach aligns well with the inherent variability and stochasticity observed in ecological processes.
Bayesian Approaches in Ecological Modeling
As students delve into the practical applications of Bayesian statistics in ecological modeling, they will uncover its versatility in addressing a myriad of research questions. One prominent application is in the estimation of population parameters, a task critical to understanding and managing wildlife populations, plant communities, and ecosystem dynamics. In population ecology, Bayesian methods shine when dealing with small sample sizes or incomplete data. Traditional frequentist approaches may struggle in such situations, leading to unreliable estimates. Bayesian modeling, on the other hand, accommodates uncertainty gracefully, yielding credible intervals that convey the plausible range of parameter values. This is particularly advantageous in conservation efforts, where accurate population size estimates are crucial for effective management strategies.
Moreover, Bayesian statistics proves invaluable in predicting ecological outcomes, a task central to understanding how environmental changes may impact ecosystems. Whether forecasting species distributions under climate change scenarios or predicting the effects of habitat alterations, Bayesian modeling allows researchers to account for uncertainties in their predictions. The incorporation of uncertainty in ecological forecasts is essential for developing robust management plans that acknowledge the inherent unpredictability of ecological systems.
Conclusion
In the dynamic realm of ecological research, the mastery of environmental statistics stands as an essential skill for students embarking on assignments that involve navigating intricate datasets. As we reflect on the multifaceted nature of ecological investigations, it becomes evident that a solid grasp of statistical methodologies is the key to unlocking the profound insights concealed within environmental data.
The journey begins with exploratory data analysis (EDA), where students are introduced to the foundational concepts of descriptive statistics. EDA provides a lens through which the intricacies of ecological datasets can be understood. Armed with measures of central tendency, variability, and distribution, students gain the ability to distill complex information into meaningful summaries.