Experimental Design and Analysis Assignments Using Excel: A Comprehensive Tutorial
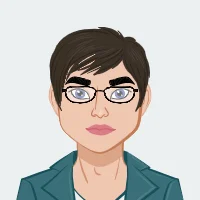
In the dynamic realm of academia, students continually encounter the intricate demands of experimental design and data analysis assignments. These academic endeavors, integral across diverse scientific disciplines, present formidable challenges that necessitate a nuanced grasp of theoretical frameworks coupled with adeptness in practical applications. The synergy between theory and practice is pivotal in unraveling the complexities of these tasks, demanding students to not only comprehend foundational concepts but also wield them adeptly in real-world scenarios. However, amid this academic maze, a beacon of support emerges in the form of Microsoft Excel. Experimental design and data analysis stand as pillars underpinning scientific inquiry, mandating a fusion of methodological finesse and analytical acumen. Students, when confronted with these tasks, often traverse through a labyrinth of variables, controls, hypotheses, and statistical methodologies. These complexities demand a tool that not only simplifies but also amplifies their ability to navigate this intricate landscape. Enter Microsoft Excel, a ubiquitous and versatile software revered for its multifaceted utility in data handling, analysis, and visualization. If you need assistance with your Excel homework, consider Microsoft Excel as a valuable ally in your academic journey, aiding you in effectively tackling the challenges posed by experimental design and data analysis assignments.
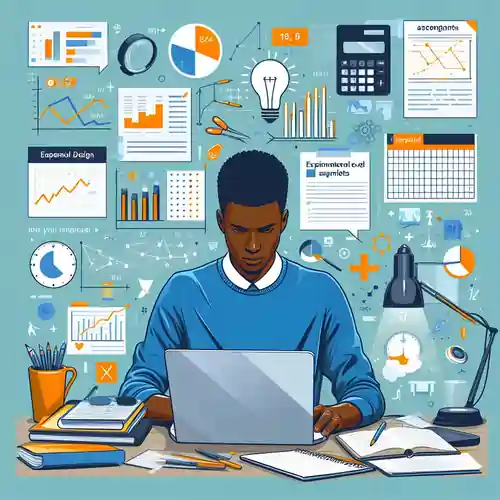
Excel serves as a formidable ally for students embarking on the journey of experimental design and analysis. This tutorial, a compendium of guidance and insights, endeavors to be the guiding light illuminating the path toward mastery in these domains. Its primary objective lies in equipping students with a comprehensive understanding and practical proficiency in leveraging Excel's functionalities to unravel the intricacies of experimental design and analysis. At its core, this tutorial offers a meticulously crafted, step-by-step roadmap that traverses the labyrinthine terrain of experimental design. It unravels the core tenets of constructing robust experiments, elucidating the significance of variables, control groups, randomization, and the orchestration of various experimental factors. By elucidating these fundamental principles, it empowers students to architect experiments that are methodologically sound and scientifically credible.
Understanding Experimental Design
Understanding Experimental Design is paramount for students engaging in scientific research, as it forms the bedrock upon which the entire investigative process rests. It serves as the blueprint, guiding researchers in crafting a robust structure and methodology that ensures the validity and reliability of their studies. Delving into the intricacies of this foundational aspect, we unravel the essential components that make up a well-designed experiment in this comprehensive tutorial.
The Basics of Experimental Design
At its core, experimental design involves the careful orchestration of various elements to uncover meaningful patterns and relationships within the data. The first step in this process is understanding the significance of variables. Variables are the building blocks of any experiment, representing the factors that can change or be manipulated. In this section, we illuminate the distinctions between independent and dependent variables, elucidating how each plays a pivotal role in shaping research outcomes. Control groups emerge as another critical facet of experimental design. These groups act as benchmarks, providing a baseline against which the effects of the independent variable can be measured. We explore the rationale behind incorporating control groups, demonstrating how they contribute to isolating the impact of the manipulated variable and enhancing the internal validity of the study.
Randomization, a seemingly simple yet potent concept, emerges as the third pillar of experimental design. The deliberate introduction of randomness mitigates the risk of bias, ensuring that each participant or sample has an equal chance of being assigned to different experimental conditions. Through vivid examples, we illustrate how randomization safeguards against confounding variables and strengthens the generalizability of research findings.
Excel Tools for Experimental Design
Excel, a ubiquitous tool in the academic and professional realms, emerges as a potent ally in the realm of experimental design. Its arsenal of features equips students with the means to streamline and enhance the efficiency of their experimental designs. From the inception of a study to the final analysis, Excel proves to be an invaluable companion. The journey begins with the creation of random samples—a task that might seem daunting without the aid of technology. Excel, however, simplifies this process through its random number generation functions. We walk students through the steps of generating random samples, emphasizing the importance of this technique in achieving representative and unbiased data.
Moving beyond randomization, Excel's prowess shines in the realm of factorial experiments. This section provides a comprehensive exploration of how students can leverage Excel to design factorial experiments with multiple independent variables. Through practical examples and step-by-step instructions, students gain proficiency in setting up and analyzing complex experimental designs, fostering a deeper understanding of the intricate relationships between variables.
Data Analysis with Excel
Data analysis with Excel represents a powerful and accessible gateway for students venturing into the realms of research and experimental design. Excel, a widely used spreadsheet software, offers a robust platform for organizing, manipulating, and extracting insights from data. This section delves into the multifaceted world of data analysis using Excel, serving as a compass for students navigating the intricacies of this essential skill set. In the initial stages, emphasis is placed on the critical foundations of data entry and cleaning.
Data Entry and Cleaning
Data is the lifeblood of any research study, and its quality directly impacts the reliability of analysis outcomes. The journey into data analysis with Excel begins with the crucial steps of data entry and cleaning. Students embarking on experimental design assignments must understand the nuances of structuring data for meaningful analysis. This involves organizing information into relevant columns and rows, ensuring clarity and consistency in variable labels, and adopting a format that facilitates easy interpretation. A significant aspect of data entry involves dealing with missing values, a common challenge in real-world datasets. Excel provides various tools to address this issue, from simple filtering techniques to advanced imputation methods. This section explores these strategies, guiding students on how to identify missing data, decide on appropriate handling methods, and implement them effectively.
The tutorial highlights Excel functions and formulas that streamline this process, ensuring a clean and complete dataset ready for in-depth analysis. Outliers, while potentially valuable in some contexts, can skew statistical results and compromise the validity of conclusions. The tutorial delves into Excel's capabilities for outlier detection, demonstrating how students can use functions and visualizations to identify and assess the impact of outliers on their data. By mastering data cleaning techniques in Excel, students lay a solid foundation for accurate and reliable analyses, setting the stage for insightful interpretations and meaningful conclusions in their assignments.
Descriptive Statistics in Excel
Once the data is meticulously entered and cleaned, the next step in the data analysis journey involves extracting meaningful insights through descriptive statistics. Excel, with its user-friendly interface and powerful functionalities, becomes an invaluable tool in this phase of the process. This section provides students with a comprehensive guide on leveraging Excel for descriptive statistics, offering a window into the main features of a dataset. Excel's functions empower students to calculate key measures such as the mean, median, and standard deviation with ease. Through clear examples and step-by-step instructions, the tutorial ensures that students not only perform these calculations but also understand the interpretation and implications of each statistic.
Illustrative visuals, generated using Excel's charting capabilities, enhance the learning experience, making abstract statistical concepts more tangible. Furthermore, the tutorial explores Excel's capabilities in handling categorical data, guiding students on creating frequency distributions, pie charts, and bar graphs. By visually representing data, students can communicate findings more effectively and gain a deeper understanding of patterns and trends within their datasets. Excel's versatility in generating various types of charts allows students to choose the most suitable visualization method for their specific data, enhancing the clarity and impact of their presentations.
Advanced Data Analysis Techniques
In the realm of experimental design and analysis, mastering advanced data analysis techniques is essential for extracting meaningful insights from data sets. Going beyond the surface-level understanding offered by descriptive statistics, students delve into the realm of inferential statistics, a powerful tool for making predictions and drawing broader conclusions about populations based on sample data.
Inferential Statistics with Excel
Inferential statistics form the cornerstone of sophisticated data analysis, allowing students to move beyond the mere description of data and into the realm of hypothesis testing and population inference. Excel, a widely accessible and user-friendly platform, becomes the canvas for students to paint statistical narratives. This section serves as a guide through the labyrinth of inferential statistics, demystifying concepts and providing practical insights. One of the fundamental tools explored in this section is the t-test, a statistical method used to compare the means of two groups. Whether assessing the effectiveness of a new drug or examining the impact of a teaching method, t-tests provide a robust framework for hypothesis testing.
Step-by-step tutorials within Excel showcase how students can effortlessly execute t-tests, interpret results, and make informed conclusions about the significance of observed differences. Analysis of Variance (ANOVA) takes center stage as a more advanced technique, allowing students to compare means across multiple groups simultaneously. Excel's ANOVA functions empower students to explore the nuances of experimental designs with multiple factors, offering a comprehensive understanding of the variability within and between groups. Real-world examples guide students through the intricacies of ANOVA, enhancing their ability to select the right statistical tool for diverse research scenarios.
Visualizing Data with Excel Charts
While mastering inferential statistics is pivotal, the ability to effectively communicate findings is equally crucial. This subsection introduces students to Excel's robust charting capabilities, enabling them to transform raw data into visually compelling representations. Excel's charting functionalities extend beyond basic bar graphs and scatter plots. This tutorial provides an in-depth exploration of various chart types, elucidating the nuances of when to use each. Whether aiming to showcase distribution patterns, highlight trends over time, or compare different categories, students gain insights into the art of selecting the most appropriate chart to convey their message accurately.
Creating visually appealing charts is not just about aesthetics; it's about ensuring that the message is clear and impactful. This section delves into the finer details of chart customization within Excel, guiding students on aspects such as color selection, labeling, and axis scaling. By incorporating these design principles, students elevate the visual appeal of their charts, making them more accessible and compelling for their target audience.
Troubleshooting and Best Practices
In the realm of experimental design and data analysis using Excel, the journey is fraught with challenges, from elusive errors to the necessity of adopting best practices. This section, dedicated to troubleshooting and best practices, serves as a beacon for students navigating the intricacies of spreadsheet-driven exploration.
Common Errors and How to Avoid Them
Even the most proficient Excel users, seasoned researchers, and budding scholars alike, find themselves grappling with errors in the labyrinth of spreadsheet calculations. In this section, we meticulously dissect the common errors that can befuddle students during experimental design and data analysis assignments, unraveling the intricacies of Excel's potential pitfalls. One prevalent stumbling block is the mismanagement of data types. Excel, in its eagerness to assist, might misinterpret numerical data as text, leading to calculation errors. This tutorial sheds light on this issue, providing step-by-step solutions to convert data types accurately. Additionally, we delve into the realm of formula errors, elucidating the nuances of circular references, syntax missteps, and the dreaded #DIV/0! error.
By understanding the anatomy of these errors, students can preemptively address them, creating a robust foundation for their analytical endeavors. Furthermore, we explore the potential pitfalls of improper referencing and cell addressing. This often-overlooked aspect can wreak havoc on data analysis, causing inaccuracies that may go unnoticed. The tutorial guides students on how to navigate through relative and absolute references, ensuring that their formulas dynamically adapt to changes in data size or structure.
Best Practices for Efficient Analysis
To transcend mediocrity and truly excel in experimental design and data analysis assignments, students must embrace a set of best practices that lay the groundwork for efficiency and accuracy. This section transcends the conventional narrative, offering not just a checklist but a comprehensive guide to cultivating a research mindset and optimizing the analytical workflow.
The tutorial begins by emphasizing the significance of data organization. Students are guided through the art of structuring data in a logical and intuitive manner, ensuring that the dataset is not only comprehensible to others but also conducive to efficient analysis. Practical tips on naming conventions, creating headers, and utilizing Excel tables empower students to transform raw data into a well-organized foundation for their analytical journey.
Conclusion
Mastering the art of experimental design and analysis using Microsoft Excel is a crucial skill that holds immense value for students aiming to forge careers in the realms of science, research, or academia. This proficiency serves as a gateway to unlocking the full potential of their academic journey and future professional endeavors. The significance of this expertise lies not only in its immediate application to assignments but in its enduring impact on the way students approach and contribute to their respective fields.
The tutorial presented herein acts as a comprehensive roadmap, meticulously designed to usher students through the intricate landscape of experimental design, data analysis, and the nuanced process of troubleshooting common issues. The journey begins with a foundational understanding of experimental design principles, emphasizing the pivotal role of variables, control groups, and the importance of randomization.