- Unveiling Descriptive Statistics in STATA
- Overview of Descriptive Statistics
- Utilizing Graphical Representation
- Advanced Techniques for Data Summarization
- Beyond the Basics with tabulate
- Harnessing the Power of collapse and egen
- Handling Missing Data Effectively
- Identifying and Managing Missing Values
- Imputation Strategies in STATA
- Conclusion
In the contemporary landscape of data analysis, statistics stands as a dynamic field constantly evolving to meet the demands of an increasingly complex world. As the sheer volume and intricacy of data continue to expand, the need for sophisticated tools has become imperative. These tools serve as gateways to unlocking the wealth of information concealed within datasets, enabling analysts to derive meaningful and actionable insights. In this context, STATA emerges as a beacon of statistical prowess, offering a robust platform that has seamlessly integrated itself into the fabric of academic and research spheres. STATA is not merely a statistical software; it is a comprehensive analytical toolkit that has evolved to cater to the diverse needs of statisticians, researchers, and students. Its versatility lies not only in its ability to handle large datasets but also in its rich repertoire of commands and functions designed to perform intricate analyses. From basic data manipulation to complex econometric modeling, STATA provides a holistic environment for users to explore, analyze, and visualize data effectively.
As we embark on this exploration of advanced statistical techniques in STATA, our focus narrows to two fundamental pillars of statistical analysis: descriptive statistics and data summarization. Descriptive statistics form the bedrock of any analytical endeavor, offering a concise and informative summary of key characteristics within a dataset. STATA's prowess in this domain is epitomized by its 'summarize' command, a versatile tool that extends beyond elementary measures like mean and standard deviation. It encompasses more nuanced statistics such as skewness and kurtosis, affording users a comprehensive understanding of the distributional properties of their data. The significance of descriptive statistics, however, extends beyond numerical summaries. Visualization plays a pivotal role in data interpretation, and STATA's graphical capabilities, which can be vital for assistance with your STATA homework, complement its statistical prowess. Whether through histograms, box plots, or scatter plots, STATA empowers users to unravel intricate patterns and trends that might be obscured in raw numerical outputs. This visual dimension not only aids in grasping the underlying structure of the data but also enhances the communicative power of statistical findings.
Unveiling Descriptive Statistics in STATA
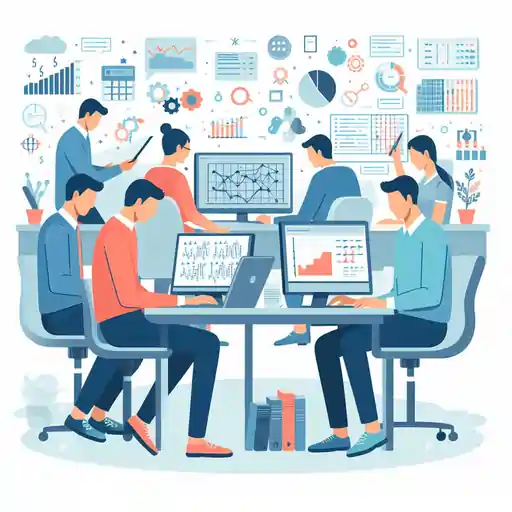
In the vast and intricate landscape of statistical analysis, the foundation of comprehension rests upon the pillars of descriptive statistics. This pivotal branch of statistics serves as a guiding light, illuminating the inherent patterns and characteristics nestled within a dataset. Within this expansive realm, STATA stands out as a beacon of statistical prowess, offering a versatile toolkit that empowers researchers and students alike. At the heart of this statistical arsenal lies the summarize command, a linchpin in the process of unraveling the mysteries concealed within the numerical fabric of data.
Overview of Descriptive Statistics
Descriptive statistics, as the term implies, are concerned with describing and summarizing the main characteristics of a dataset. When embarking on the exploration of a dataset in STATA, the summarize command becomes the go-to instrument for gaining a comprehensive understanding of its fundamental properties. This command transcends the rudimentary reporting of mean and standard deviation; it provides a nuanced perspective by incorporating measures such as skewness and kurtosis. Skewness and kurtosis are statistical measures that extend beyond the basic central tendency and dispersion metrics. Skewness assesses the asymmetry of a distribution, indicating whether the data leans towards one tail more than the other.
On the other hand, kurtosis delves into the shape of the distribution, highlighting whether the dataset has heavier or lighter tails compared to a normal distribution. These measures, offered by the summarize command in STATA, are pivotal for unraveling the distributional characteristics of data. An understanding of skewness and kurtosis is imperative for researchers and students alike. For instance, a positively skewed dataset implies that the majority of values cluster towards the lower end, while a negative skew suggests concentration towards the higher end. Similarly, kurtosis aids in identifying outliers and assessing the overall variability of the dataset. Armed with these insights, users can make informed decisions about the nature of the data distribution and choose appropriate statistical methods for further analysis.
Utilizing Graphical Representation
While descriptive statistics provide a numerical foundation, numbers alone might not unveil the complete narrative. This is where the visual prowess of STATA comes into play. Beyond the numerical outputs of the summarize command, STATA boasts a rich array of graphical tools designed to complement and enhance the understanding of descriptive statistics. From the simplicity of histograms to the intricacies of box plots, these visualizations serve as windows into the underlying patterns and structures of the data.
Histograms offer a visual representation of the distribution, showcasing peaks, troughs, and any evident patterns that might be obscured in raw numerical data. On the other hand, box plots provide a succinct summary of the data's central tendency, dispersion, and potential outliers. In this segment, we will explore not only the mechanics of creating these visualizations but also the art of interpretation. Understanding how to craft compelling graphs in STATA is more than a technical skill; it is a storytelling device. These visual aids empower students to present their findings in a visually appealing and informative manner, transforming complex datasets into narratives that resonate with their audience.
Advanced Techniques for Data Summarization
In the dynamic landscape of statistical analysis, where the pursuit of proficiency is an ongoing journey, students and researchers are increasingly turning to advanced functionalities within statistical software to extract richer insights from their datasets. Among these capabilities, data summarization emerges as a critical dimension, serving as the linchpin for distilling meaningful patterns and trends from complex data structures. In this section, we delve into the advanced techniques offered by STATA, shedding light on two commanding features: 'tabulate' and the synergistic interplay of 'collapse' and 'egen.' These tools, each with its unique strengths, collectively bolster the analytical toolkit available to students, empowering them to navigate the intricacies embedded in their datasets with precision and depth.
Beyond the Basics with tabulate
The tabulate command in STATA emerges as a game-changer, ushering students beyond the analysis of individual variables into the realm of dynamic relationships between variables. This command functions as a catalyst for efficient data exploration by generating frequency tables and cross-tabulations. These tabulations, rather than being mere numerical summaries, serve as windows into the underlying structure of the dataset, unveiling patterns and associations that may be obscured when examining variables in isolation. By facilitating a comprehensive overview of categorical data, the tabulate command provides users with a versatile toolkit for dissecting their datasets. Through its nuanced implementation, students can discern trends, dependencies, and anomalies, laying the groundwork for informed decision-making in their assignments.
From exploring the distribution of variables across different categories to investigating conditional relationships, the tabulate command proves to be an indispensable ally in the pursuit of a deeper understanding of complex datasets. As this section unfolds, users will be guided through the intricacies of the tabulate command, unlocking its potential to be more than just a statistical tool. It becomes a lens through which students can view their data holistically, facilitating the identification of patterns that might otherwise remain elusive. Through hands-on examples and step-by-step instructions, students will gain not only the technical know-how but also the intuition to leverage the tabulate command effectively in their statistical endeavors.
Harnessing the Power of collapse and egen
However, the landscape of data summarization extends beyond the capabilities of individual commands. Recognizing this, STATA introduces the dynamic duo of 'collapse' and 'egen,' offering users a more granular and customizable approach to summary statistics and variable creation. Sometimes, standard measures provided by built-in commands fall short of capturing the nuanced aspects of a dataset. The collapse command in STATA facilitates the aggregation of data, enabling users to compute summary statistics such as totals, means, or variances across specified groups. This proves particularly useful when dealing with large datasets, allowing users to distill information into manageable and insightful summaries.
Moreover, in the toolkit of advanced summarization techniques, 'egen' stands out as a Swiss army knife. This command empowers users to generate new variables based on a myriad of operations, from calculating cumulative sums to creating group-specific averages. Through a journey into the functionalities of 'collapse' and 'egen,' this section aims to demystify the process of creating tailored summary statistics and variables. Users will learn how to navigate these commands to derive information that goes beyond the standard output, unlocking the ability to answer nuanced research questions and overcome the challenges posed by complex assignments. The exploration of 'collapse' and 'egen' serves as a testament to STATA's commitment to providing a flexible and robust environment for statistical analysis, enabling users to elevate their data summarization game and emerge as adept analysts in the field of statistics.
Handling Missing Data Effectively
In the intricate landscape of statistical analysis, the omnipresent challenge of dealing with missing data necessitates adept strategies to ensure the integrity and reliability of study outcomes. STATA, a statistical software revered for its versatility, empowers users with an arsenal of tools specifically designed to navigate the complexities associated with missing data. This section will shed light on the significance of handling missing data effectively and how STATA becomes an invaluable ally in this endeavor. Missing data poses a formidable hurdle in the path of researchers and statisticians, casting shadows on the accuracy and comprehensiveness of their analyses. This challenge is pervasive across diverse fields, ranging from social sciences to healthcare, where the absence of certain observations can significantly impact the validity of study results.
Identifying and Managing Missing Values
The first step in grappling with missing data is to identify its presence within a dataset. STATA simplifies this process through the implementation of the missing command. This command not only pinpoints the location and extent of missing values but also provides essential summary statistics, such as the percentage of missingness across variables. Armed with this information, users can make informed decisions about the most suitable course of action.
Once identified, the management of missing values becomes a critical aspect of data analysis. STATA offers a spectrum of options, allowing users to decide whether to impute missing values or exclude them from analysis. Imputation involves estimating missing values based on observed data, while exclusion involves omitting cases with missing data. The choice between these strategies hinges on the nature of missingness and the potential impact on the study's validity. Through practical examples and demonstrations, users will gain proficiency in navigating this decision-making process, ensuring a judicious approach to handling missing data.
Imputation Strategies in STATA
Imputing missing values is a nuanced task that demands a thoughtful consideration of the dataset's characteristics. STATA rises to the occasion by offering a repertoire of imputation methods, catering to the diverse needs of researchers. The simplest approach involves mean imputation, where missing values are replaced with the mean of observed values for a particular variable. While straightforward, this method might oversimplify the underlying patterns and variability within the data.
For more sophisticated analyses, STATA provides advanced imputation techniques, including multiple imputation. Multiple imputation generates several complete datasets with imputed values, reflecting the uncertainty associated with missing data. This approach not only preserves the variability in the dataset but also produces more accurate standard errors and confidence intervals. By guiding students through the intricacies of each imputation method, this section ensures that they can make informed choices based on the specific nuances of their data, fostering a deeper understanding of the imputation process.
Conclusion
In the intricate tapestry of statistical analysis, the mastery of advanced techniques within the STATA environment emerges as a non-negotiable asset for students engaged in the rigors of assignments and research projects. This comprehensive guide has acted as a compass, steering learners through the diverse terrains of descriptive statistics and data summarization, unraveling the immense potential embedded within STATA's command-driven capabilities.
The significance of mastering advanced statistical techniques cannot be overstated. As students grapple with complex datasets, the proficiency in utilizing STATA commands becomes a linchpin for extracting meaningful insights. The landscape of statistical analysis is not static; it's a dynamic ecosystem where nuanced understanding and application of tools can be the difference between superficial findings and profound discoveries. This guide serves as a beacon, illuminating the path toward analytical excellence.