- The Basics of Bootstrapping in SPSS
- How to Conduct Basic Bootstrapping in SPSS
- Interpreting Results and Advantages of Basic Bootstrapping
- Advanced Applications of Bootstrapping in SPSS
- Bootstrapping Regression Analysis in SPSS
- Bootstrapping for Mediation and Moderation Analyses
- Addressing Challenges and Considerations in Bootstrapping
- Dealing with Skewed Distributions and Outliers
- Choosing the Right Number of Bootstrap Samples
- Conclusion
Statistical analysis serves as a cornerstone in the realm of data exploration, allowing researchers and analysts to derive meaningful insights from complex datasets. Yet, the efficacy of such analyses is intrinsically tied to the assumptions inherent in traditional statistical methods. These assumptions, ranging from normality to homoscedasticity, can pose significant challenges, especially when working with real-world data that may deviate from idealized conditions. Amidst the constraints imposed by these assumptions, bootstrapping emerges as a potent resampling technique, offering a resilient alternative to conventional statistical approaches. It becomes particularly invaluable in scenarios where meeting the stringent assumptions of traditional methods becomes impractical or unattainable. In the context of this blog, we embark on an exploration of the world of bootstrapping methods within the framework of the Statistical Package for the Social Sciences (SPSS), unraveling the ways in which students can harness this technique to elevate the accuracy and reliability of their statistical analyses.
Bootstrapping, in essence, is a resampling method characterized by the repeated drawing of samples with replacement from the observed data.This departure from the conventional approach of assuming a fixed underlying distribution allows for a more flexible and robust analysis. This flexibility proves to be a key asset when confronted with challenges such as small sample sizes or datasets that do not conform to the normal distribution, which is a common occurrence in real-world research. Within the confines of SPSS, the implementation of bootstrapping is remarkably accessible, providing students with a user-friendly platform to enhance their statistical endeavors, including solving their SPSS homework. The ease with which bootstrapping can be executed in SPSS makes it a practical choice for those navigating the complexities of statistical analyses. By opting for bootstrapping, students can obtain estimates of parameters that are more reliable, contributing to a higher level of confidence in the inferences drawn from their analyses.
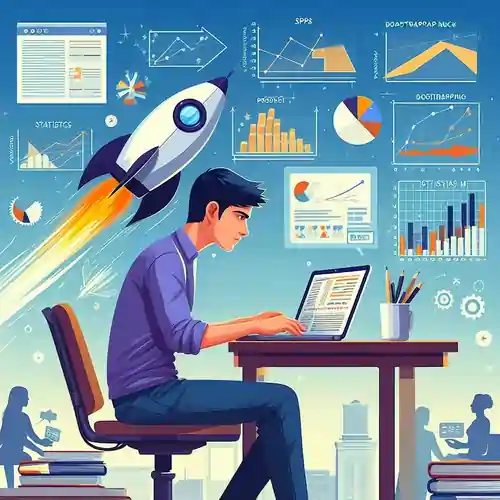
The Basics of Bootstrapping in SPSS
Bootstrapping stands as a versatile and indispensable statistical technique, empowering researchers to extract more reliable estimates from their datasets. Its application within the realm of SPSS opens a gateway to enhanced accuracy and robust statistical inferences. To comprehend the intricacies of this method, let's delve into a detailed breakdown of the essential steps involved in implementing basic bootstrapping. At the core of bootstrapping lies the idea of resampling with replacement. This process is particularly valuable when faced with challenges such as small sample sizes or data distributions that deviate from the normal. Here's a detailed breakdown of the essential steps:
How to Conduct Basic Bootstrapping in SPSS
The first step in basic bootstrapping is Data Preparation. Load your dataset into SPSS, ensuring that variables of interest are properly defined. This initial stage sets the foundation for accurate and meaningful analysis. Once your data is loaded, proceed to the Selecting Analysis phase. Navigate to the Analyze menu, choose the Descriptive Statistics option, and then select Bootstrapping. This step initiates the process of resampling, which is the core principle of bootstrapping. With the analysis tool in place, move on to Variable Configuration. Specify the variables for which you intend to conduct bootstrapping. Whether you're interested in computing confidence intervals for means, medians, or other statistics, this step allows you to tailor the analysis to your specific research questions.
After defining your variables, proceed to Setting Parameters. Configure the number of bootstrap samples and the desired confidence level. A common and reasonable choice is often 1,000 bootstrap samples with a 95% confidence interval. These parameters influence the precision and reliability of your results. With all settings in place, it's time to Run the Analysis. Execute the process, and SPSS will generate bootstrap samples from your dataset. For each sample, the software computes the desired statistic, whether it's a mean, median, or any other parameter of interest. This step forms the heart of bootstrapping, as it involves the creation of multiple datasets through resampling.
Interpreting Results and Advantages of Basic Bootstrapping
Once the analysis is complete, the next critical step is Interpreting Results. Examine the output to gain insights into the distribution of the statistic of interest. Bootstrapping results typically include bias-corrected and accelerated (BCa) confidence intervals, providing a more accurate estimation of the parameter. The advantages of basic bootstrapping are manifold. Firstly, it leads to Increased Precision in Parameter Estimates. By generating multiple samples and calculating the statistic of interest for each, bootstrapping reduces the impact of outliers and variations inherent in small sample sizes, resulting in more precise estimates.
Secondly, basic bootstrapping exhibits Enhanced Robustness to Violations of Normality. Traditional statistical methods often assume normality, but real-world data may not always conform to this assumption. Bootstrapping, being distribution-free, doesn't rely on such assumptions, making it a robust choice for non-normally distributed data. Furthermore, basic bootstrapping provides the Ability to Derive Confidence Intervals for Almost Any Statistic. This flexibility is particularly advantageous when dealing with diverse research questions and a wide range of statistical measures. Whether estimating medians, proportions, or other parameters, bootstrapping offers a versatile approach for deriving accurate confidence intervals.
Advanced Applications of Bootstrapping in SPSS
Bootstrapping, a resampling technique, unfolds as a versatile statistical tool, stretching far beyond its fundamental role in basic parameter estimation. Its applications extend into more complex realms, providing robust solutions to challenges inherent in traditional statistical methods. One such area where bootstrapping showcases its power is in the domain of regression analysis, particularly within the context of SPSS. Traditional regression analysis relies on stringent assumptions, two of which include homoscedasticity and normally distributed residuals. However, real-world data often deviate from these assumptions, presenting a significant challenge to the reliability of traditional regression results.
Bootstrapping Regression Analysis in SPSS
Regression analysis is a fundamental statistical tool used to explore relationships between variables, but its reliability hinges on the fulfillment of stringent assumptions, notably homoscedasticity and normal distribution of residuals. In the real world, however, data rarely conform to these ideal conditions, presenting a challenge for researchers. Enter bootstrapping regression in SPSS, a powerful and flexible technique that serves as a remedy when traditional assumptions are violated. To embark on bootstrapping for regression analysis in SPSS, the procedure closely mirrors that of basic bootstrapping. Users initiate the process by navigating to the Regression menu, where they can find the Bootstrapping option. The subsequent steps align with the basic bootstrapping approach: selecting variables of interest, configuring the number of bootstrap samples, and defining the desired confidence level. This user-friendly process makes it accessible to students and researchers seeking to elevate their analyses beyond the constraints of traditional regression methods.
The distinctive strength of bootstrapping in regression lies in its capacity to generate a distribution of coefficients, ushering in a more accurate estimation of standard errors and confidence intervals. Unlike traditional regression, which assumes a fixed set of coefficients, bootstrapping introduces variability through resampling with replacement from the original dataset. This variability is crucial, especially when confronting issues such as non-normality or heteroscedasticity in residuals. The heart of bootstrapping's effectiveness lies in its adaptability to the intricacies of real-world data. Traditional regression assumes that residuals exhibit constant variance and follow a normal distribution. However, in practice, this assumption is often untenable.
Bootstrapping for Mediation and Moderation Analyses
Moving beyond basic parameter estimation, bootstrapping in SPSS plays a crucial role in the complex realms of mediation and moderation analyses. These advanced techniques explore relationships between variables, examining indirect effects and moderation effects, respectively. For mediation and moderation analyses, SPSS provides a specialized tool through the PROCESS macro developed by Andrew F. Hayes. This macro streamlines the process of conducting mediation and moderation analyses, integrating bootstrapping to enhance the accuracy and reliability of results.
In the context of mediation analysis, bootstrapping assists in estimating indirect effects. Traditional methods may struggle with the assumptions required for accurate mediation testing, but bootstrapping provides a non-parametric approach, allowing students to obtain confidence intervals for the indirect effects without relying on strict assumptions. Moreover, when it comes to moderation analysis, bootstrapping aids in testing the significance of moderation effects. By resampling the data and computing the moderation effects in each bootstrap sample, students gain a distribution of effects, enabling more robust statistical inference.
Addressing Challenges and Considerations in Bootstrapping
Bootstrapping stands as a formidable resampling technique, wielding significant influence in the realm of statistical analysis. Its emergence marked a paradigm shift, providing researchers, particularly students, with a tool to address the limitations and assumptions associated with traditional statistical methods. As a non-parametric method, bootstrapping operates by resampling the observed data with replacement, generating numerous bootstrap samples to estimate the variability of a statistic or parameter. This technique's widespread adoption stems from its ability to deliver advantages that extend beyond the constraints of conventional approaches. Nevertheless, its efficacy is not without its caveats, necessitating a nuanced understanding of the challenges and considerations inherent in its application.
Dealing with Skewed Distributions and Outliers
One of the significant strengths of bootstrapping lies in its resilience to skewed distributions and outliers. However, it's essential to recognize that extreme values can still exert an influence on the outcomes. Skewed distributions, characterized by a lack of symmetry, and outliers, data points significantly different from the rest, can distort the resampled estimates. In such situations, students should adopt proactive strategies to mitigate the impact of skewed distributions and outliers. One approach is to consider transforming variables before conducting bootstrapping. Techniques like logarithmic or square root transformations can help normalize the data, making it more amenable to the assumptions of bootstrapping.
Alternatively, students can explore alternative resampling methods designed to be more robust in the presence of skewed data. Methods like the wild bootstrap or bias-corrected and accelerated (BCa) bootstrap may be more suitable when dealing with non-normally distributed data. By addressing these challenges head-on, students can ensure that bootstrapping remains a reliable tool even in the face of skewed distributions and outliers.
Choosing the Right Number of Bootstrap Samples
The number of bootstrap samples chosen is a critical factor influencing the accuracy and precision of results derived from bootstrapping. While the instinct may be to believe that an abundance of samples always leads to superior outcomes, the reality demands a nuanced understanding. Striking a delicate balance is essential for students navigating the terrain of selecting the optimal number of bootstrap samples. The fundamental principle is that an increase in the number of bootstrap samples generally correlates with improved accuracy in parameter estimates and heightened reliability in the results obtained. However, this advantage is not without its drawbacks, as it introduces a trade-off involving computational resources and time constraints. The relationship between the number of bootstrap samples and the precision of results is pivotal. As the number of samples rises, the estimates derived from the resampling process tend to converge towards the true population parameters. This convergence, in turn, leads to narrower confidence intervals and a more refined understanding of the underlying statistical properties. It is, therefore, logical to conclude that a higher number of bootstrap samples contributes positively to the robustness of statistical inferences.
Yet, this seemingly straightforward principle encounters practical challenges, especially in the realm of large datasets and complex analyses. As the number of bootstrap samples escalates, so does the demand on computational resources and time. The impracticality of an excessive number of bootstrap samples becomes apparent in scenarios where the computational burden surpasses the available resources, rendering the analysis unfeasible. For students engaging in statistical analyses, a careful consideration of the trade-off between precision and efficiency is imperative when deciding on the number of bootstrap samples. The complexity of the dataset under investigation and the computational power at the students' disposal should be the guiding factors in making this decision. Larger datasets and intricate analyses may necessitate a compromise, advocating for a balance that ensures the practicality of the analysis without sacrificing statistical precision.
Conclusion
In the dynamic landscape of statistical analysis, where precision and reliability are paramount, bootstrapping emerges as an invaluable tool within the realm of SPSS. This resampling method not only caters to the intricacies of small sample sizes but also stands as a robust alternative when data distributions deviate from the assumptions inherent in traditional statistical approaches. Its significance is particularly pronounced for students navigating the challenges of statistical assignments, providing them with a means to fortify the accuracy of their analyses.
Whether students are engaged in basic parameter estimation or grappling with the intricacies of complex mediation analyses, bootstrapping in SPSS offers a versatile and reliable methodology. At its core, this technique involves the generation of innumerable bootstrap samples through random sampling with replacement from the observed data. It is these resampled datasets that serve as the foundation for obtaining more robust estimates of parameters and constructing confidence intervals. The flexibility inherent in bootstrapping aligns seamlessly with the diverse needs of researchers across various fields, making it a preferred choice for those seeking enhanced statistical validity.