Claim Your Discount Today
Get 10% off on all Statistics Homework at statisticshomeworkhelp.com! This Spring Semester, use code SHHR10OFF to save on assignments like Probability, Regression Analysis, and Hypothesis Testing. Our experts provide accurate solutions with timely delivery to help you excel. Don’t miss out—this limited-time offer won’t last forever. Claim your discount today!
We Accept
- Types of Missing Data in SPSS
- Missing Completely at Random (MCAR)
- Missing at Random (MAR)
- Strategies for Handling Missing Data in SPSS
- Imputation Techniques
- Advanced Techniques for Missing Data
- Best Practices for Dealing with Missing Data
- Data Collection Strategies
- Transparent Reporting and Documentation
- Conclusion
Missing data represents a ubiquitous challenge in the field of statistical analysis, posing a significant hurdle to researchers and students striving for reliable and robust results. The impact of missing data on statistical analyses cannot be overstated, as it has the potential to compromise the validity and reliability of study findings. This phenomenon can introduce bias and diminish the precision of results, thereby increasing the likelihood of drawing inaccurate or misleading conclusions. Addressing missing data is not merely a technicality but a critical aspect of the research process that demands careful consideration and strategic intervention. In the landscape of statistical software, SPSS (Statistical Package for the Social Sciences) emerges as a prominent tool extensively employed by students and researchers. Its popularity can be attributed to its user-friendly interface, versatility, and a comprehensive array of analytical features tailored to social science research. If you need assistance with your SPSS homework, understanding how to address missing data in SPSS is crucial for ensuring the validity and reliability of your statistical analyses.
However, despite its robust capabilities, SPSS users often encounter the challenge of missing data, necessitating a nuanced understanding of strategies to navigate this issue effectively. To embark on a journey of unraveling effective strategies for handling missing data in SPSS, it is imperative to recognize the profound implications of missing data on the broader research process. At the heart of this issue lies the potential distortion of results, creating a ripple effect that extends from the initial data analysis to the interpretation and conclusions drawn from the study. The presence of missing data introduces a layer of uncertainty, and the manner in which it is addressed directly influences the integrity of the entire research endeavor. The primary consequence of missing data lies in its ability to introduce bias into statistical analyses. Bias occurs when the missing data is not random but systematically related to certain characteristics of the study, leading to a skewed representation of the true population. This bias can manifest in various forms, such as underestimating or overestimating relationships between variables, thereby distorting the overall research findings. Consequently, the reliability of the results becomes questionable, and any subsequent conclusions drawn may not accurately reflect the underlying reality. Moreover, missing data has a detrimental impact on the precision of statistical results. The reduction in precision arises from the diminished sample size resulting from the exclusion of cases with missing data. A smaller sample size inherently yields less statistical power, making it challenging to detect true effects and increasing the risk of Type II errors – the failure to reject a false null hypothesis. This compromised precision not only hampers the internal validity of the study but also limits the generalizability of findings to the broader population.
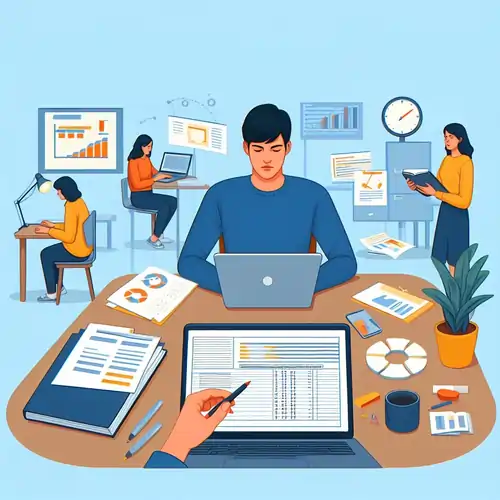
Types of Missing Data in SPSS
In the realm of statistical analysis, understanding the nature of missing data is crucial for implementing effective strategies in tools like SPSS. Two common types of missing data are Missing Completely at Random (MCAR) and Missing at Random (MAR). In this section, we will delve into the characteristics of each type and explore the techniques SPSS provides for handling them.
Missing Completely at Random (MCAR)
In scenarios characterized by Missing Completely at Random (MCAR), the probability of data being missing is unrelated to both observed and unobserved variables within the dataset. This essentially means that the missing values are a product of random chance and are not systematically related to any specific variable. In an ideal world, all instances of missing data would be MCAR, as it suggests that the missing values represent a random and unbiased subset of the overall data. Handling MCAR in SPSS involves employing various techniques, each with its own set of advantages and limitations. One common approach is Listwise Deletion, which involves excluding cases with missing values from the analysis entirely. While this method is straightforward, it may lead to a significant reduction in sample size, potentially impacting the statistical power of the analysis.
Another option is Pairwise Deletion, which uses all available data for each analysis. This means that cases with missing values are included in analyses where they have complete data, maximizing the use of available information. However, this method can lead to varying sample sizes across different analyses, potentially complicating the interpretation of results. A more sophisticated approach to handling MCAR is through Multiple Imputation. This technique generates multiple datasets, each with imputed values for missing data. The analyses are then conducted on each imputed dataset, and the results are combined to provide a more accurate and robust estimate. Multiple Imputation acknowledges the uncertainty associated with missing data and offers a more nuanced understanding of the potential impact on the analysis.
Missing at Random (MAR)
In contrast to MCAR, situations involving Missing at Random (MAR) imply that the probability of missing data is related to observed variables but not to unobserved variables. This means that the missingness can be explained by the values of other variables in the dataset. For example, if participants with higher income are less likely to provide certain information, the missing data is considered to be at random with respect to the unobserved variables. SPSS equips researchers with various imputation methods to address MAR effectively. Mean Imputation involves replacing missing values with the mean of the observed values for that variable. While this method is simple, it assumes that the missing data is missing completely at random within each category of the observed variables.
Regression Imputation is another method provided by SPSS for MAR scenarios. It involves predicting the missing values based on the relationships observed in the rest of the data. This approach is more sophisticated than mean imputation but assumes a linear relationship between variables. For complex datasets, researchers might consider Propensity Score Imputation, a technique in which missing values are imputed based on the probability of their occurrence given observed variables. This method helps balance observed variables between cases with and without missing data, offering a more nuanced and accurate imputation.
Strategies for Handling Missing Data in SPSS
Handling missing data is a critical aspect of statistical analysis, and SPSS provides a suite of strategies to address this challenge. Among these strategies, imputation stands out as a fundamental approach, involving the replacement of missing values with estimated values based on observed data. In this section, we will delve into the imputation techniques offered by SPSS and explore both conventional and advanced methods, shedding light on their merits, drawbacks, and the underlying assumptions that students need to comprehend for informed decision-making in their assignments.
Imputation Techniques
Imputation is a commonly employed strategy to handle missing data in SPSS. It encompasses several techniques, each with its unique characteristics and considerations. One straightforward method is mean imputation, where missing values are replaced with the mean of the observed values for that variable. This method is simple and easy to implement, making it a quick solution for datasets with sporadic missing entries. However, it comes with a caveat—mean imputation assumes that the missing values are missing completely at random (MCAR), which may not always be the case in real-world scenarios. Another imputation technique is median imputation, which replaces missing values with the median of the observed values. Median imputation is less sensitive to extreme values than mean imputation, making it a robust choice when dealing with skewed distributions. However, similar to mean imputation, it assumes MCAR, and its effectiveness can be compromised if the data distribution is significantly skewed.
Regression imputation is a more sophisticated technique that involves predicting missing values based on the relationship with other variables in the dataset. SPSS allows users to perform regression imputation, leveraging the information from observed variables to estimate missing values accurately. This method is particularly useful when the missingness is related to other observed variables, assuming a linear relationship between variables. Nevertheless, like all imputation techniques, regression imputation rests on the assumption of the data being MCAR or missing at random (MAR), requiring students to carefully evaluate the appropriateness of this method for their specific dataset.
Advanced Techniques for Missing Data
While conventional imputation methods are valuable, SPSS extends its capabilities with advanced techniques, offering students a more nuanced approach to handling missing data. One such advanced technique is multiple imputation, a powerful strategy that generates multiple datasets, each with different imputed values. This approach recognizes the uncertainty associated with missing data and produces more accurate standard errors and confidence intervals. Multiple imputation involves creating multiple copies of the dataset, imputing missing values in each copy, and then analyzing each imputed dataset separately. The results are combined to provide a more robust and comprehensive analysis, accounting for the variability introduced by the imputation process.
However, it's important to note that multiple imputation requires a deeper understanding of statistical concepts and assumptions. Students can benefit significantly from exploring the intricacies of multiple imputation, especially when dealing with complex datasets or when conventional imputation methods may not be suitable. Understanding the underlying statistical principles empowers students to make informed decisions about which imputation technique aligns with their data characteristics and research objectives.
Best Practices for Dealing with Missing Data
Missing data is an inevitable aspect of statistical analysis, and addressing it effectively requires a proactive approach starting from the initial stages of data collection. In this section, we will delve into best practices for dealing with missing data, emphasizing the importance of robust data collection strategies and transparent reporting in the context of SPSS.
Data Collection Strategies
Preventing missing data begins at the inception of a research project, with a focus on robust data collection strategies. Students engaging in statistical analyses using SPSS must be cognizant of potential sources of missing data and employ measures to minimize its occurrence.
Effective Communication with Participants
Communication with participants is another critical element in mitigating missing data. Clear and concise instructions enhance participant understanding and encourage accurate responses. Establishing a connection with participants, explaining the importance of their responses, and ensuring confidentiality fosters a collaborative environment that minimizes the likelihood of incomplete or inaccurate data.
Diligent Data Entry Procedures
Once data is collected, diligent data entry procedures become paramount. Errors in data entry can introduce missing values or inaccuracies, compromising the quality of the dataset. Implementing double-entry verification, where data is entered independently by two individuals, and employing validation rules to check for outliers and inconsistencies can significantly reduce the risk of missing data due to data entry errors.
Transparent Reporting and Documentation
Transparency in reporting and documentation is equally essential when dealing with missing data. SPSS users must diligently document the methods employed for handling missing data, providing a clear trail for others to follow and assess the impact of missing data on the results.
Documenting Handling Methods
Students should explicitly document whether they chose listwise deletion, imputation, or any other technique to address missing data in their SPSS analyses. This documentation serves multiple purposes – it allows for the replication of the analysis, enables others to understand the rationale behind the chosen method, and facilitates the identification of potential biases introduced by the handling strategy.
Stating Limitations and Assumptions
Transparent reporting extends to stating the limitations and assumptions associated with the chosen handling method. Acknowledging the inherent uncertainties and potential biases helps in contextualizing the results for a more nuanced interpretation. Whether the missing data is assumed to be missing completely at random (MCAR) or missing at random (MAR), clearly stating these assumptions contributes to the overall transparency of the analysis.
Conclusion
In Conclusion, the realm of statistical analysis using SPSS, the task of addressing missing data is not merely a technical challenge but a nuanced interplay of theoretical comprehension and practical application. As students venture into this aspect of data manipulation, they must recognize that a one-size-fits-all approach does not exist. Instead, a thoughtful consideration of the nature of missing data within their datasets is paramount for effective handling.
The process of choosing appropriate strategies involves a delicate balance between theory and application. Whether opting for simple imputation methods or delving into the complexities of advanced techniques such as multiple imputation, students must be cognizant of the specific advantages and limitations associated with each approach. Simple imputation methods, like mean or median imputation, may provide quick solutions but at the cost of potentially oversimplifying the reality of the data. On the other hand, advanced techniques such as multiple imputation, while offering a more nuanced and comprehensive solution, demand a deeper understanding of statistical concepts.