Claim Your Discount Today
Get 10% off on all Statistics homework at statisticshomeworkhelp.com! Whether it’s Probability, Regression Analysis, or Hypothesis Testing, our experts are ready to help you excel. Don’t miss out—grab this offer today! Our dedicated team ensures accurate solutions and timely delivery, boosting your grades and confidence. Hurry, this limited-time discount won’t last forever!
We Accept
- Understanding the Basics of Hypothesis Testing
- Defining Hypotheses
- Types of Tests
- Example Scenario
- Calculating the Test Statistic
- Formula for the T-Test Statistic
- Standard Error
- Determining the P-Value
- Calculating the P-Value
- Example Calculation
- Drawing Conclusions
- Significance Level ((\alpha))
- Decision Rule
- Example Conclusion
- Practical Examples of Hypothesis Testing
- Left-Tailed Test Example
- Right-Tailed Test Example
- Two-Tailed Test Example
- Tips for Conducting Hypothesis Testing
- Ensure
- Verify Assumptions
- Utilize Software Tools
- Interpret Results Carefully
- Report Results Transparently
- Common Pitfalls in Hypothesis Testing
- Misinterpreting P-Values
- Ignoring Assumptions
- Overemphasizing Statistical Significance
- Conclusion
Hypothesis testing is a fundamental statistical technique used to make inferences about populations based on sample data. This blog will guide you through the process of hypothesis testing, helping you understand and apply the concepts to solve similar assignments efficiently. By following this structured approach, you'll be able to solve your hypothesis testing homework problem with confidence.
Understanding the Basics of Hypothesis Testing
Hypothesis testing involves making a decision about the validity of a hypothesis based on sample data. It comprises four key steps: defining hypotheses, calculating the test statistic, determining the p-value, and drawing conclusions. Let's explore each of these steps in detail.
Defining Hypotheses
The first step in hypothesis testing is to define the null and alternative hypotheses. These hypotheses represent the statements we want to test.
Null Hypothesis (H0)
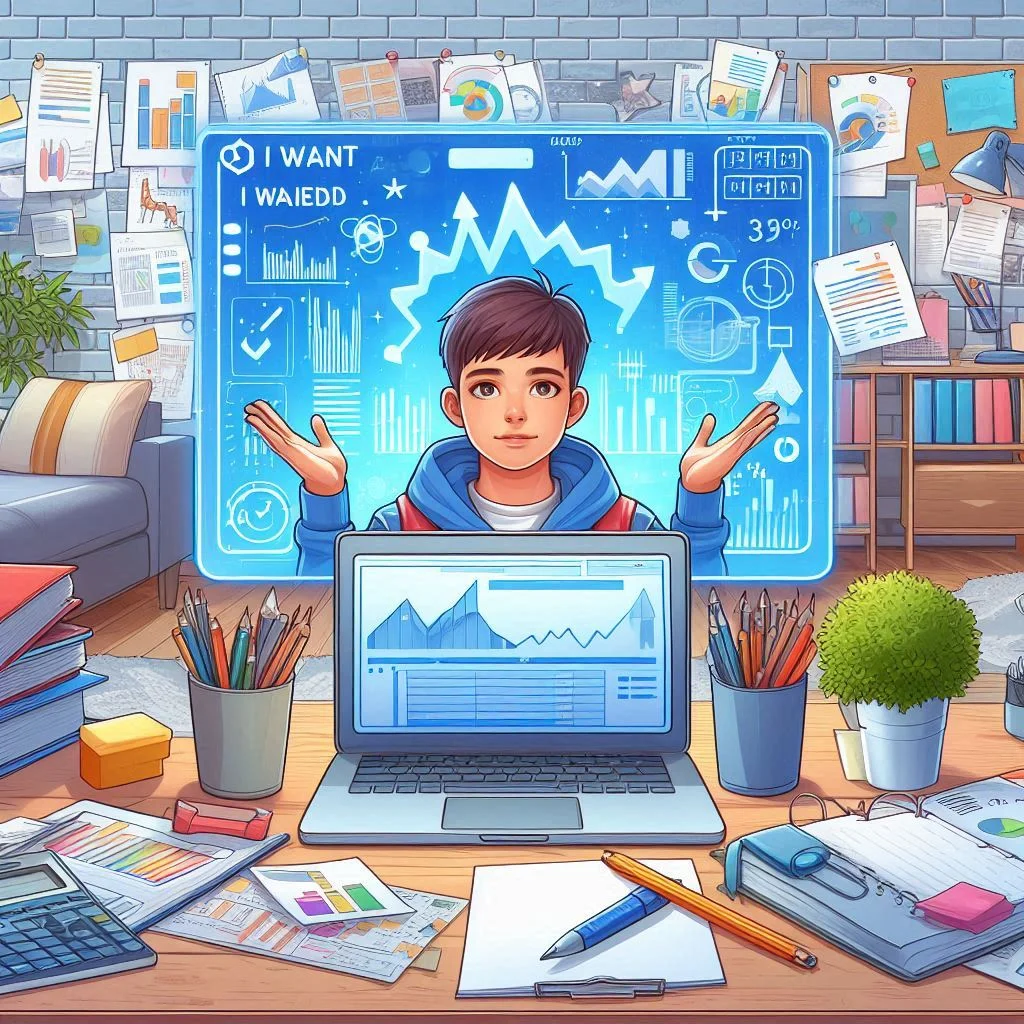
The null hypothesis (H0) is a statement that there is no effect or difference. It serves as the default assumption that we aim to test against.
Alternative Hypothesis (Ha or H1)
The alternative hypothesis (Ha or H1) is a statement that indicates the presence of an effect or difference. It represents what we want to prove.
Types of Tests
Depending on the direction of the hypothesis, we have three types of tests: left-tailed, right-tailed, and two-tailed tests.
Left-Tailed Test
A left-tailed test is used when we want to determine if the population mean is less than a specified value.
Right-Tailed Test
A right-tailed test is used when we want to determine if the population mean is greater than a specified value.
Two-Tailed Test
A two-tailed test is used when we want to determine if the population mean is different from a specified value, either higher or lower.
Example Scenario
Consider a scenario where we want to test if the average vehicle price from a sample is less than $27,000. We would set up our hypotheses as follows:
- Null Hypothesis (H0): μ = 27,000
- Alternative Hypothesis (Ha):μ < 27,000
Calculating the Test Statistic
Once the hypotheses are defined, the next step is to calculate the test statistic. The test statistic helps us determine the likelihood of observing the sample data under the null hypothesis.
Formula for the T-Test Statistic
The t-test statistic is calculated using the formula:
[ t = \frac{\bar{X} - \mu}{S / \sqrt{n}} ]
Where:
- (\bar{X}) is the sample mean
- (S) is the sample standard deviation
- (n) is the sample size
- (\mu) is the population mean defined in the null hypothesis
Standard Error
The denominator of the t-test formula, (S / \sqrt{n}), is known as the standard error (SE). It measures the variability of the sample mean.
Example Calculation
Let's calculate the test statistic for our vehicle price example. Given:
- Sample mean ((\bar{X})) = 25,650
- Sample standard deviation (S) = 3,488
- Sample size (n) = 10
- Population mean ((\mu)) = 27,000
First, we calculate the standard error (SE):
[ SE = \frac{S}{\sqrt{n}} = \frac{3488}{\sqrt{10}} \approx 1103 ]
Next, we calculate the test statistic (t):
[ t = \frac{25650 - 27000}{1103} \approx -1.2238 ]
Determining the P-Value
The p-value is a critical component of hypothesis testing. It indicates the probability of obtaining a test statistic as extreme as the one observed, assuming the null hypothesis is true.
Calculating the P-Value
The method to calculate the p-value depends on the type of test (left-tailed, right-tailed, or two-tailed) and the direction of the alternative hypothesis.
Left-Tailed Test
For a left-tailed test, the p-value is calculated using the T.DIST() function in Excel.
Right-Tailed Test
For a right-tailed test, the p-value is calculated using the T.DIST.RT() function in Excel.
Two-Tailed Test
For a two-tailed test, the p-value is calculated using the T.DIST.2T() function in Excel. When the test statistic is negative, use the absolute value function (ABS()) to remove the negative sign before calculating the p-value.
Example Calculation
For our vehicle price example with a left-tailed test, we calculate the p-value using the T.DIST() function in Excel:
[ \text{p-value} = T.DIST(-1.2238, 9, TRUE) \approx 0.1261 ]
Drawing Conclusions
The final step in hypothesis testing is to draw a conclusion based on the p-value and a pre-determined significance level ((\alpha)).
Significance Level ((\alpha))
The significance level ((\alpha)) is the threshold for deciding whether to reject the null hypothesis. Common values for (\alpha) are 0.05, 0.01, 0.10, and 0.005.
Decision Rule
- If the p-value is less than (\alpha), we reject the null hypothesis.
- If the p-value is greater than (\alpha), we fail to reject the null hypothesis.
Example Conclusion
For our vehicle price example with (\alpha = 0.05):
- p-value = 0.1261
- (\alpha) = 0.05
Since 0.1261 > 0.05, we fail to reject the null hypothesis. There is not enough evidence to suggest that the average vehicle price is less than $27,000.
Practical Examples of Hypothesis Testing
To further illustrate hypothesis testing, let's explore three different scenarios: left-tailed test, right-tailed test, and two-tailed test.
Left-Tailed Test Example
In this example, we test if the average vehicle price is less than $27,000.
Step-by-Step Process
Define Hypotheses:
- Null Hypothesis (H0): μ = 27,000
- Alternative Hypothesis (Ha): μ < 27,000
Calculate Test Statistic:
- Sample mean ((\bar{X})) = 25,650
- Sample standard deviation (S) = 3,488
- Sample size (n) = 10
- Population mean ((\mu)) = 27,000
- Standard error (SE) = 1103
- Test statistic (t) = -1.2238
Determine P-Value:
- p-value = 0.1261
Draw Conclusion:
- (\alpha) = 0.05
- Since 0.1261 > 0.05, fail to reject the null hypothesis.
- Conclusion: There is not enough evidence to suggest that the average vehicle price is less than $27,000.
Right-Tailed Test Example
In this example, we test if the average vehicle price is greater than $23,500.
Step-by-Step Process
Define Hypotheses:
- Null Hypothesis (H0): μ = 23,500
- Alternative Hypothesis (Ha):μ > 23,500
Calculate Test Statistic:
- Sample mean ((\bar{X})) = 25,650
- Sample standard deviation (S) = 3,488
- Sample size (n) = 10
- Population mean ((\mu)) = 23,500
- Standard error (SE) = 1103
- Test statistic (t) = 1.9490
Determine P-Value:
- p-value = 0.0416
Draw Conclusion:
- (\alpha) = 0.05
- Since 0.0416 < 0.05, reject the null hypothesis.
- Conclusion:There is enough evidence to suggest that the average vehicle price is greater than $23,500.
Two-Tailed Test Example
In this example, we test if the average vehicle price is different from $23,500.
Step-by-Step Process
Define Hypotheses:
- Null Hypothesis (H0): μ = 23,500
- Alternative Hypothesis (Ha):μ ≠ 23,500
Calculate Test Statistic:
- Sample mean ((\bar{X})) = 25,650
- Sample standard deviation (S) = 3,488
- Sample size (n) = 10
- Population mean ((\mu)) = 23,500
- Standard error (SE) = 1103
- Test statistic (t) = 1.9490
Determine P-Value:
- p-value = 0.0831
Draw Conclusion:
- (\alpha) = 0.05
- Since 0.0831 > 0.05, fail to reject the null hypothesis.
- Conclusion: There is not enough evidence to suggest that the average vehicle price is different from $23,500.
Tips for Conducting Hypothesis Testing
Successfully conducting hypothesis testing involves several critical steps. Here are some tips to help you perform hypothesis testing effectively.
Ensure
Proper Data Collection
Accurate and reliable data collection is crucial for hypothesis testing. Ensure that your sample is representative of the population and collected using appropriate methods.
Random Sampling
Use random sampling techniques to avoid bias and ensure that your sample accurately represents the population.
Sample Size
Ensure that your sample size is large enough to provide reliable results. Larger sample sizes reduce the margin of error and increase the power of the test.
Verify Assumptions
Hypothesis tests often rely on certain assumptions about the data. Verify these assumptions before proceeding with the test.
Normality
Many hypothesis tests, including the t-test, assume that the data follows a normal distribution. Use graphical methods (e.g., histograms, Q-Q plots) or statistical tests (e.g., Shapiro-Wilk test) to check for normality.
Independence
Ensure that the observations in your sample are independent of each other. Independence is a key assumption for most hypothesis tests.
Utilize Software Tools
Software tools like Excel, R, and SPSS can simplify the calculations involved in hypothesis testing and reduce the risk of errors.
Excel
Excel provides several functions for hypothesis testing, such as T.DIST(), T.DIST.RT(), and T.DIST.2T(). Use these functions to calculate p-values and make decisions based on your test statistics.
R
R is a powerful statistical software that offers various packages for hypothesis testing. Use functions like t.test() to perform t-tests and obtain p-values and confidence intervals.
Interpret Results Carefully
Proper interpretation of the results is crucial for drawing accurate conclusions from hypothesis testing.
Statistical Significance
A statistically significant result (p-value < (\alpha)) indicates that there is strong evidence against the null hypothesis. However, it does not imply practical significance. Consider the context and the practical implications of the results.
Type I and Type II Errors
Be aware of the potential for Type I and Type II errors. A Type I error occurs when the null hypothesis is incorrectly rejected, while a Type II error occurs when the null hypothesis is not rejected despite being false. The significance level ((\alpha)) affects the probability of Type I errors, while the sample size and effect size influence the probability of Type II errors.
Report Results Transparently
When reporting the results of hypothesis testing, include all relevant information to ensure transparency and reproducibility.
Detailed Description
Provide a detailed description of the hypotheses, test statistic, p-value, significance level, and the conclusion. This information helps others understand and evaluate your analysis.
Confidence Intervals
Include confidence intervals for the estimated parameters. Confidence intervals provide a range of plausible values for the population parameter and offer additional context for interpreting the results.
Common Pitfalls in Hypothesis Testing
Hypothesis testing is a powerful tool, but it is essential to be aware of common pitfalls to avoid incorrect conclusions.
Misinterpreting P-Values
P-values indicate the probability of obtaining a test statistic as extreme as the one observed, assuming the null hypothesis is true. A small p-value suggests strong evidence against the null hypothesis, but it does not provide a measure of the effect size or practical significance.
P-Value Misconceptions
Avoid common misconceptions about p-values, such as believing that a p-value of 0.05 means there is a 5% chance that the null hypothesis is true. P-values do not measure the probability that the null hypothesis is true or false.
Ignoring Assumptions
Ignoring the assumptions underlying hypothesis tests can lead to incorrect conclusions. Always verify the assumptions before proceeding with the test.
Assumption Violations
If the assumptions are violated, consider using alternative tests that do not rely on those assumptions. For example, if the data is not normally distributed, use non-parametric tests like the Wilcoxon rank-sum test or the Mann-Whitney U test.
Overemphasizing Statistical Significance
Statistical significance does not imply practical significance. A result can be statistically significant but have a negligible practical effect. Always consider the context and practical implications of the results.
Effect Size
Report and interpret effect sizes alongside p-values. Effect sizes provide a measure of the magnitude of the observed effect and offer valuable context for interpreting the results.
Conclusion
Hypothesis testing is a critical tool in statistics for making inferences about populations based on sample data. By understanding the steps involved—defining hypotheses, calculating the test statistic, determining the p-value, and drawing conclusions—you can approach hypothesis testing with confidence.
Ensure proper data collection, verify assumptions, utilize software tools, interpret results carefully, and report findings transparently to enhance the reliability and validity of your hypothesis tests. By avoiding common pitfalls and considering both statistical and practical significance, you'll be well-equipped to tackle statistics homework and research projects effectively.