Claim Your Discount Today
Get 10% off on all Statistics homework at statisticshomeworkhelp.com! Whether it’s Probability, Regression Analysis, or Hypothesis Testing, our experts are ready to help you excel. Don’t miss out—grab this offer today! Our dedicated team ensures accurate solutions and timely delivery, boosting your grades and confidence. Hurry, this limited-time discount won’t last forever!
We Accept
- Step 1: Understanding the Assignment
- Step 2: Data Preparation and Exploration
- Step 3: Conducting Basic Statistical Analysis
- Step 4: Advanced Statistical Analysis
- Step 5: Data Visualization and Interpretation
- Step 6: Critical Evaluation and Reflection
- Conclusion:
Quantitative data analysis is an essential skill for students in fields like social sciences, economics, business, and health sciences. Whether you're dealing with experiments, surveys, or observational studies, understanding how to properly analyze quantitative data is crucial for drawing meaningful conclusions from your research. This guide will provide you with a structured approach to tackling assignments involving quantitative data analysis, using common statistical methods that are applicable to various scenarios. If you find yourself needing additional help with data analysis homework, following these steps will enable you to confidently navigate similar assignments and develop a strong foundation in data analysis.
Step 1: Understanding the Assignment
Before diving into the analysis, it's important to thoroughly understand the assignment. Read the questions carefully and identify the key tasks you're being asked to perform. Common tasks in quantitative data analysis assignments include:
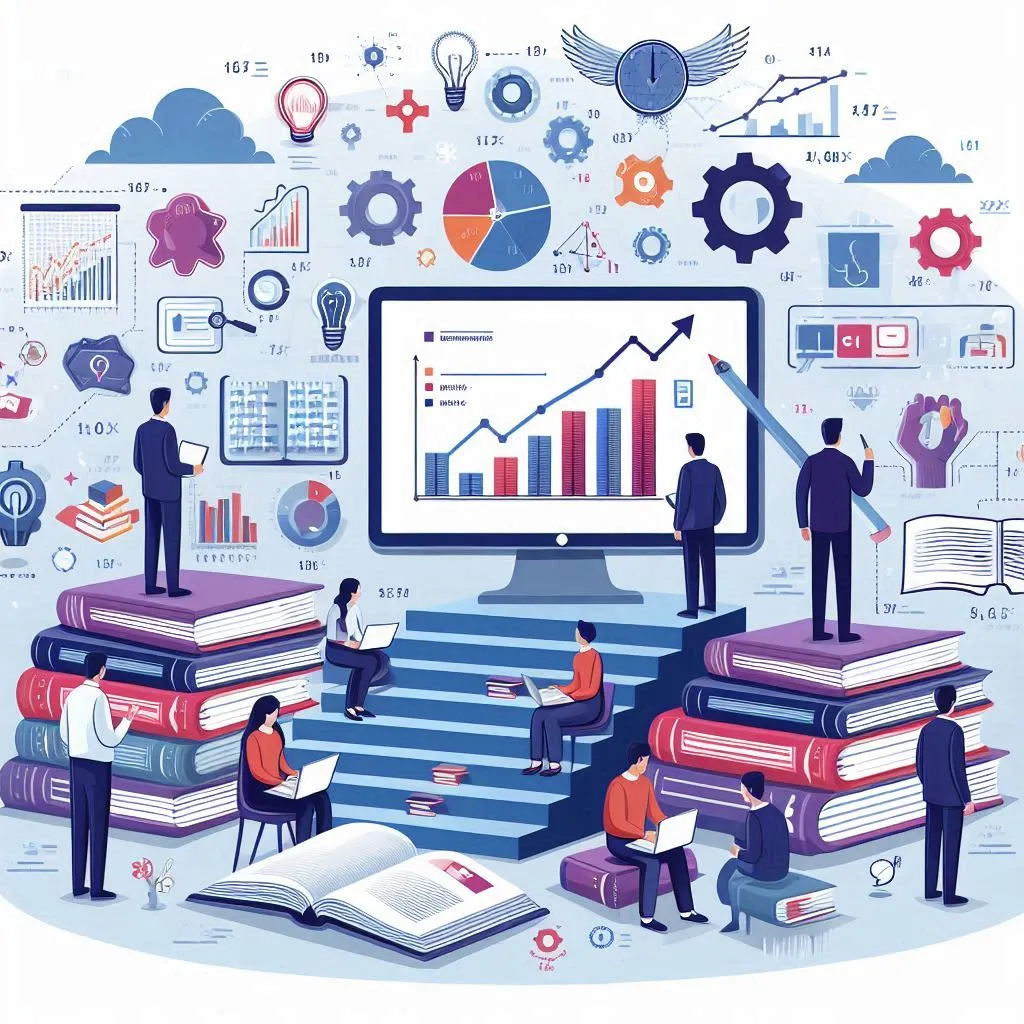
- Calculating averages or means: Understanding the central tendency of your data.
- Interpreting results: Drawing conclusions from your statistical calculations.
- Conducting hypothesis tests: Assessing whether your results are statistically significant.
- Building regression models: Examining relationships between variables.
- Visualizing data: Using graphs to represent your findings.
Make sure you know what each question is asking for, as this will guide your analysis and help you focus on the relevant statistical methods.
Step 2: Data Preparation and Exploration
Data preparation is a critical step in any quantitative analysis. Start by loading your dataset into a statistical software program such as R, Python, or SPSS. Once your data is loaded, it's time to explore the data and clean it if necessary. Here are some key steps:
- Check for Missing Data: Missing data can skew your results. Determine whether you need to impute missing values, exclude them, or use statistical methods that can handle missing data.
- Examine Descriptive Statistics: Calculate basic descriptive statistics (mean, median, standard deviation, etc.) to get an overview of your data. This will help you understand the distribution of your variables and identify any outliers.
- Visualize the Data: Create visualizations such as histograms, box plots, and scatter plots to better understand the distribution and relationships within your data. Visualizations can reveal patterns that may not be immediately apparent from descriptive statistics alone.
Step 3: Conducting Basic Statistical Analysis
Once you have a good understanding of your data, you can start performing basic statistical analyses. These analyses often include calculating means, standard errors, and confidence intervals. Let’s explore some of these methods in detail:
- Calculating the Mean: The mean (average) is a measure of central tendency that is commonly used to summarize data. In an assignment, you might be asked to calculate and interpret the mean to understand the general behavior of a dataset, such as the average effect of a treatment.
- Example: If you're examining the effect of an educational program on student scores, calculating the mean post-treatment score can give you an idea of the program's overall effectiveness.
- Example: To evaluate the reliability of the mean score you've calculated, you might calculate the standard error and then determine the 95% confidence interval. This will tell you with 95% confidence that the true mean lies within a certain range.
- Example: If you're comparing the average scores of two groups of students, you can use a t-test to determine if the difference in means is statistically significant at a 95% confidence level.
Step 4: Advanced Statistical Analysis
In more complex assignments, you may be required to perform advanced analyses such as regression modeling or difference-in-differences estimation. These methods allow you to analyze relationships between variables and assess causal effects.
- Linear Regression: Regression analysis is used to model the relationship between a dependent variable and one or more independent variables. Simple linear regression involves one independent variable, while multiple regression involves two or more.
- Example: If you're examining how student grade level and a treatment program affect reading scores, you might build two models: one that only includes the treatment and another that controls for grade level. Comparing these models can help you understand how much of the variation in scores is explained by the treatment alone versus when controlling for grade.
- Example: To assess the impact of an educational program, you could use DiD to compare reading scores before and after the program, while accounting for differences between treated and control groups.
- Example: You might find that the effect of a treatment on student scores varies by grade level. Including an interaction term between treatment and grade level in your model can help you assess this relationship.
Step 5: Data Visualization and Interpretation
Once you've completed your statistical analyses, it's time to interpret your results and present them clearly. This often involves creating tables, graphs, and writing concise summaries of your findings.
- Tables and Graphs:Tables are useful for presenting detailed regression results, while graphs can effectively illustrate key findings, such as trends over time or differences between groups.
- Example: You might use a scatter plot to compare student scores at the beginning and end of the year, with separate colors for treated and control groups. This can visually demonstrate the effect of the treatment.
- Example: If your analysis shows that the treatment has a statistically significant effect on student scores, you should also consider the size of this effect and its implications for educational policy.
- Example: You might write a paragraph summarizing your findings on the effect of educational television on student reading scores, explaining that the program led to a modest but statistically significant improvement in scores.
Step 6: Critical Evaluation and Reflection
A crucial part of any quantitative analysis is critically evaluating your approach and results. This includes considering potential limitations and alternative explanations for your findings.
- Assessing Model Fit: Evaluate how well your models explain the variation in your data. This can involve looking at R-squared values in regression models or comparing predicted values with actual data.
- Example: If your model explains a low percentage of the variance in student scores, you might need to consider additional variables or alternative modeling approaches.
- Example: In your analysis of the effect of a treatment program, consider whether other factors (e.g., differences in teacher quality) might be driving the observed effect, rather than the treatment itself.
- Example: If you had access to more detailed data on student backgrounds, you could control for these factors in your analysis to better isolate the effect of the treatment.
Conclusion:
Quantitative data analysis is a powerful tool for understanding complex relationships in data and making informed decisions based on evidence. By following a structured approach to your assignments, you can develop the skills needed to confidently analyze data and draw meaningful conclusions.
Whether you’re calculating averages, conducting hypothesis tests, or building regression models, the key is to approach each task methodically and thoughtfully. Remember to always critically evaluate your results and consider the broader implications of your findings. With practice and persistence, you’ll become proficient in quantitative analysis and be well-prepared to tackle any statistics homework in the future.