Claim Your Discount Today
Get 10% off on all Statistics Homework at statisticshomeworkhelp.com! This Spring Semester, use code SHHR10OFF to save on assignments like Probability, Regression Analysis, and Hypothesis Testing. Our experts provide accurate solutions with timely delivery to help you excel. Don’t miss out—this limited-time offer won’t last forever. Claim your discount today!
We Accept
- Complete Case Analysis
- Pairwise Deletion
- Pros and Cons
- Imputation Methods
- Mean/Median Imputation
- Multiple Imputation
- Model-Based Methods
- Regression Imputation
- Bayesian Methods
- Conclusion:
Statistics homework poses a myriad of challenges to students, and among the most formidable obstacles is the presence of missing data. The causes of missing data are diverse, ranging from inadvertent data entry errors to survey participants opting not to respond. Sometimes, missing data is inherent to the data collection process itself, adding an extra layer of complexity to statistical analyses. Navigating through these challenges and effectively addressing missing data is pivotal for obtaining accurate, reliable, and meaningful results in statistical analysis. Dealing with missing data requires a nuanced approach, and this comprehensive guide aims to equip students with a repertoire of strategies to overcome this common hurdle. By understanding and implementing these strategies, students can bolster the integrity and validity of their statistical findings. One of the primary reasons for missing data in statistics homework is data entry errors. Students, while transcribing data from one source to another, may inadvertently omit certain values or input incorrect information. Recognizing this source of missing data is the first step in devising strategies to address it. To mitigate data entry errors, students should employ double-checking mechanisms during the data entry process. This involves carefully reviewing the entered data for any discrepancies and cross-referencing it with the original source. Software tools with built-in validation checks can also be utilized to minimize the occurrence of these errors, ensuring that the data entered is accurate and complete. Non-response from survey participants is another common source of missing data, especially in survey-based research. Individuals may choose not to answer certain questions for various reasons, leading to gaps in the dataset. In such cases, understanding the reasons behind non-response is crucial. If the non-response is random, it might not significantly impact the validity of the analysis.
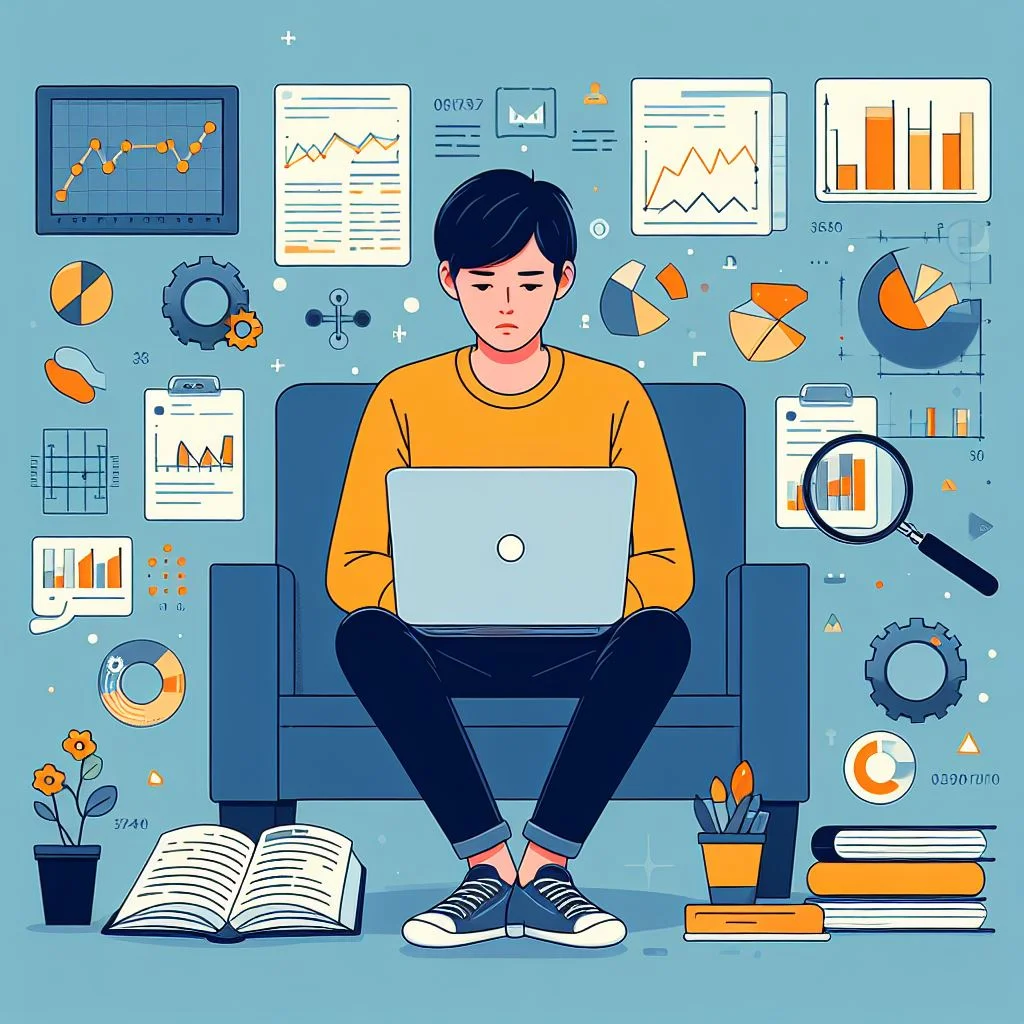
However, if there is a pattern to the non-response, it could introduce bias into the results. To address this, researchers can employ imputation techniques, wherein missing values are replaced with estimated values based on the patterns observed in the rest of the dataset. Imputation helps maintain the sample size and ensures that the analysis is not unduly influenced by the missing data. The nature of the data collection process itself can contribute to missing data. For example, in longitudinal studies where data is collected over an extended period, participants may drop out, leading to missing observations. Recognizing the mechanisms behind missing data in longitudinal studies is essential for employing appropriate strategies. Techniques such as multiple imputation, where missing values are imputed multiple times to account for uncertainty, can be particularly useful in such scenarios. Additionally, sensitivity analyses can be conducted to assess the robustness of the findings under different assumptions about the missing data. In the pursuit of handling missing data effectively, students should also consider the Missing Completely at Random (MCAR), Missing at Random (MAR), and Missing Not at Random (MNAR) frameworks. MCAR implies that the likelihood of data being missing is unrelated to both observed and unobserved data. MAR suggests that the probability of missing data is related to observed variables but not to the unobserved ones. MNAR, on the other hand, indicates that the missing data is related to the unobserved variables, leading to potential bias. Understanding these frameworks helps in choosing appropriate methods for handling missing data based on the underlying mechanisms.
Complete Case Analysis
Also known as listwise deletion, complete case analysis is a straightforward method where any observation with missing values is entirely excluded from the analysis. This simplicity is both a strength and a weakness. On the positive side, implementing complete case analysis is easy, making it a practical choice for researchers. However, the ease of application comes at a cost.
The primary advantage of complete case analysis lies in its simplicity. Researchers can quickly implement this strategy without complex computational procedures. However, the main drawback is the potential for biased results, especially when missing data are not randomly distributed. If the missing values are related to the outcome of interest, the exclusion of cases with missing data may introduce systematic errors, compromising the validity of the analysis.
Pairwise Deletion
Pairwise deletion, in contrast, involves including all available data for each specific analysis, effectively ignoring missing values on a case-by-case basis. This approach maximizes the use of available data, presenting a potential advantage over complete case analysis. However, like its counterpart, pairwise deletion has its own set of pros and cons. The strength of pairwise deletion lies in its ability to make the most of the available information without completely excluding cases with missing data. This method is particularly useful when dealing with datasets where missing values occur sporadically and are unrelated to the variables of interest.
Researchers can conduct analyses on a case-by-case basis, incorporating all available information. However, the major drawback of pairwise deletion is its vulnerability to biased estimates and standard errors if the missing data are not missing completely at random (MCAR). If the pattern of missingness is related to the variables under investigation, the results may be distorted, leading to inaccurate conclusions. In situations where the missing data are not MCAR, researchers should exercise caution when employing pairwise deletion to avoid compromising the integrity of their analyses.
Pros and Cons
Both complete case analysis and pairwise deletion offer simplicity in handling missing data, but they come with inherent risks that researchers must carefully weigh. The decision between these methods should be guided by the nature of the dataset and the characteristics of the missing data. The advantage of complete case analysis lies in its simplicity of implementation, but researchers must be mindful of potential biases and reduced statistical power.
On the other hand, pairwise deletion maximizes available data but requires caution, as it may lead to biased estimates if the missing data are not missing completely at random. Ultimately, researchers must assess the trade-offs between simplicity and potential bias, considering the specific context of their study. As the statistical landscape continues to evolve, exploring alternative methods for handling missing data, such as imputation techniques, may offer a more balanced approach, striking a compromise between simplicity and accuracy in statistical analyses.
Imputation Methods
In the realm of statistical analysis, missing data can pose a significant challenge, potentially compromising the integrity and power of research findings. Imputation methods emerge as crucial tools in addressing this challenge, offering a means to estimate missing values based on observed data. This not only preserves sample size but also maximizes statistical power, ensuring a more robust analysis. Among the plethora of imputation techniques available, two noteworthy methods are Mean/Median Imputation and Multiple Imputation, each carrying its unique set of strengths and limitations.
Mean/Median Imputation
Mean or median imputation stands out as one of the simplest methods for handling missing data. This technique involves replacing missing values with either the mean or median of the observed data for the respective variable. While its simplicity makes it an attractive choice, mean/median imputation has notable limitations that researchers must consider. The primary advantage of mean/median imputation lies in its ease of implementation. Calculating the mean or median of the observed data for a specific variable is a straightforward process, making it accessible even to those with limited statistical expertise.
However, the simplicity of this method comes at a cost, particularly in terms of its inability to account for variability in the data. One significant drawback of mean/median imputation is its susceptibility to bias, especially when the missing data are non-random. By replacing missing values with a single value (mean or median), this approach assumes a uniformity that may not reflect the true nature of the underlying data distribution. As a result, estimates derived from mean/median imputation can be skewed, leading to inaccurate and potentially misleading results.
Multiple Imputation
In contrast to the simplicity of mean/median imputation, multiple imputation represents a more sophisticated and robust approach to handling missing data. This method goes beyond providing a single imputed value and instead generates multiple plausible values for each missing data point. These imputed values are derived based on the observed data and an assumed model for the missing data mechanism. The strength of multiple imputation lies in its ability to account for the inherent uncertainty associated with imputed values. Rather than relying on a singular imputation, multiple plausible values are created, reflecting the range of possible outcomes given the available information. Subsequently, these imputed values undergo separate analyses, and the results are amalgamated using appropriate statistical techniques.
This multifaceted approach to imputation not only acknowledges the complexity of missing data scenarios but also provides a more nuanced understanding of the potential variability in the results. Researchers leveraging multiple imputation can derive more accurate estimates and make informed inferences, even in the presence of missing data. While multiple imputation requires a more intricate implementation process compared to mean/median imputation, its benefits far outweigh the complexity. Researchers seeking a comprehensive and reliable solution for handling missing data in their analyses often turn to multiple imputation as a preferred method due to its ability to address the limitations associated with simpler imputation techniques.
Model-Based Methods
In the dynamic landscape of statistical analysis and data interpretation, addressing missing values is a critical aspect that influences the accuracy and reliability of results. Model-based methods stand out as a versatile and effective approach to handle missing data, employing statistical models to predict and impute values that are absent in the observed dataset. This article delves into two significant facets of model-based methods: Regression Imputation and Bayesian Methods.
Regression Imputation
Regression imputation is a widely used technique in handling missing data, particularly when there is a need to predict values based on the relationship between variables. The core idea behind regression imputation is to utilize a regression model to estimate the missing values by considering the relationships observed in the available data. In this approach, a regression model is built using the variables that are complete or have minimal missingness. The model then predicts the missing values based on the observed values of other variables. This prediction is made under the assumption of a linear relationship between the variables, implying that the missing values are estimated as a function of the observed data.
While regression imputation offers a straightforward way to handle missing data, it comes with certain assumptions and limitations. One of the key assumptions is the linearity of the relationship between variables. If the relationship is non-linear, or if the data includes categorical variables, the performance of regression imputation may be compromised. In such cases, more sophisticated imputation techniques, such as multiple imputation or Bayesian methods, may be more appropriate. Despite its limitations, regression imputation can produce accurate imputations under certain conditions. It is particularly useful when the missingness mechanism is related to the observed variables used in the regression model. Additionally, regression imputation is computationally efficient and easy to implement, making it a popular choice in practice.
Bayesian Methods
Bayesian methods offer a flexible and powerful framework for handling missing data by incorporating uncertainty in the imputed values. These methods are based on Bayesian statistical principles, which involve updating prior beliefs about the data distribution based on observed data. In the context of missing data imputation, Bayesian methods leverage the observed data and prior knowledge about the data distribution to estimate the missing values. Unlike traditional imputation techniques that provide a single imputed value, Bayesian methods generate a distribution of plausible values for each missing data point.
By considering uncertainty in the imputed values, Bayesian methods offer several advantages over deterministic imputation techniques. They provide a more comprehensive representation of the uncertainty inherent in the imputation process, allowing researchers to make more informed decisions about the analysis results. Moreover, Bayesian methods allow for the incorporation of prior information, which can improve the accuracy of imputations, especially in situations with limited observed data. This feature makes Bayesian methods particularly valuable in settings where external information or expert knowledge is available.
Conclusion:
Handling missing data is a critical aspect of statistical analysis because it directly impacts the accuracy and reliability of study findings. When data are missing, it creates gaps in the dataset, potentially skewing statistical estimates and leading to biased conclusions. Therefore, understanding how to effectively handle missing data is essential for ensuring the integrity of statistical analyses. The choice of an appropriate strategy for handling missing data depends on several factors, each of which plays a crucial role in determining the most suitable approach. One of the primary considerations is the nature of the missing data itself.
Missing data can occur for various reasons, such as data entry errors, participant non-response, or systematic issues in the data collection process. The pattern and mechanism of missingness can significantly influence the choice of handling strategy. For instance, if the missing data are missing completely at random (MCAR), simpler methods like complete case analysis or mean imputation may be appropriate. However, if the missing data exhibit a non-random pattern, such as missingness related to certain demographic characteristics or specific survey questions, more sophisticated techniques like multiple imputation or model-based methods may be necessary to avoid biased results.