- Misinterpretation or Misinterpretation of The Problem Statement:
- Inadequate Data Cleaning:
- Neglecting Data Exploration:
- Incorrect Data Analysis Techniques Selection:
- Failure to Validate and Test Models:
- Overlooking Data Visualization's Importance:
- Inadequate Result Interpretation and Reporting:
- Conclusion:
In today's increasingly data-driven world, it's critical for students to develop strong data analysis skills. Understanding the complexities and subtleties of data analysis is critical in many fields, including business, healthcare, technology, and academia. Nonetheless, many students make several common mistakes while working on data analysis assignments, often without realizing it. In this blog, we will look in depth at those errors, their consequences, and, most importantly, how to avoid them. Data analysis is critical in many academic disciplines and professional fields. It entails systematically examining, interpreting, and organizing raw data in order to derive meaningful insights and make informed decisions. Data analysis is a skill that is required for success whether you are a student studying statistics, a researcher conducting experiments, or a business professional analyzing market trends. Navigating the world of data analysis, on the other hand, can be a daunting task, especially when it comes to completing data analysis homework assignments. To ensure accurate results and meaningful interpretations, the process requires a careful and meticulous approach. Unfortunately, many students and professionals make common errors that impede their progress and lead to incorrect conclusions.
We will look at the most common mistakes to avoid when doing data analysis homework in this comprehensive guide. Understanding these pitfalls and learning how to avoid them will equip you to handle data analysis tasks effectively and produce accurate results.
We will look at the critical errors that students frequently make, such as misinterpreting data and using inappropriate statistical methods, as well as failing to validate assumptions and mishandling missing data. By highlighting these errors and providing practical solutions, we hope to provide you with the knowledge and strategies you need to confidently tackle data analysis assignments.
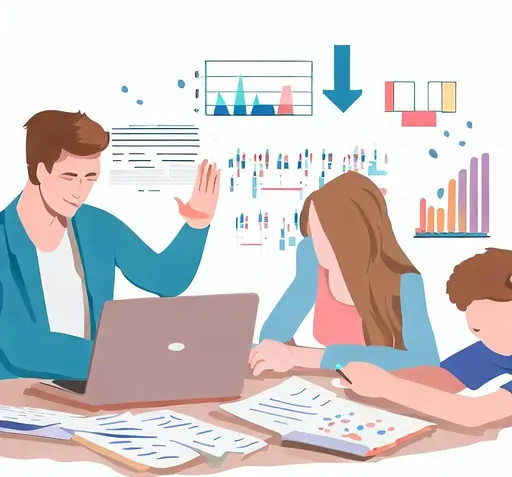
Whether you're new to data analysis or looking to improve your skills, this blog will be a valuable resource in avoiding common pitfalls and maximizing your success in data analysis homework. So, let's delve into the complexities of data analysis and uncover the blunders you should avoid if you want to excel in this field.
Misinterpretation or Misinterpretation of The Problem Statement:
The first and most serious mistake made when doing data analysis homework is misinterpreting or misinterpreting the problem statement. The first step in any data analysis task is to accurately understand what the problem is asking and what it hopes to accomplish. Misinterpretation at this stage can result in flawed analysis and incorrect results because you may be working with incorrect data or employing ineffective analytical methods.
To avoid this problem, read and comprehend the problem statement thoroughly. Divide it into smaller sections and clarify any ambiguous or complex terms or phrases. Make sure you understand the purpose of the analysis, the questions that must be answered, the data you are given, and the data analysis techniques that should be used. This comprehension is the foundation of your entire analytical process. A solid foundation can help to ensure a smooth workflow and accurate results, whereas a shaky one can derail your entire analysis.
Inadequate Data Cleaning:
Another common error is insufficient data cleaning. Inconsistencies, inaccuracies, missing values, and outliers are common in raw data. These can introduce bias, skew your results, or render your conclusions meaningless if they are not addressed prior to the analysis. However, many students overlook the significance of this step, either due to ignorance or the mistaken belief that it will not have a significant impact on the outcome.
To mitigate this, it is critical to devote significant time to data cleaning. First, identify and correct any data errors, such as misspellings or incorrect entries. Then, handle missing values appropriately - you may need to fill them using an appropriate method or, in some cases, remove the corresponding data points. Finally, identify and treat any outliers that may have distorted your analysis. The key is to remember that data cleaning is not an afterthought, but rather an essential part of the data analysis process.
Neglecting Data Exploration:
Many students proceed directly to advanced analysis without first performing exploratory data analysis (EDA). EDA is a critical component of data analysis because it allows you to gain an understanding of the underlying structure and characteristics of your data. If you skip this step, you risk missing important patterns, trends, and relationships in your data, resulting in a surface-level analysis that doesn't fully leverage the insights your data can provide.
Begin your data analysis with EDA. This includes descriptive statistics like mean, median, mode, variance, and range, which give you an idea of the central tendency and dispersion of your data. It also includes graphical representations such as histograms, box plots, and scatter plots, which show the distribution of your data and the relationships between variables. Furthermore, EDA can assist you in identifying potential issues with your data, such as skewness, kurtosis, or multicollinearity, allowing you to address them before moving forward with further analysis.
Incorrect Data Analysis Techniques Selection:
One of the most damaging errors you can make is selecting the wrong data analysis techniques. With so many statistical tests, models, and algorithms to choose from, it's easy to become overwhelmed and make the wrong decision. An incorrect technique can produce misleading results and interpretations, leading to incorrect conclusions.
To avoid this, you must have a thorough understanding of various data analysis techniques, as well as their assumptions and applicability. If you're going to use regression analysis, chi-square tests, t-tests, ANOVA, or machine learning algorithms, make sure you understand the principles behind them and when they should be used. If you have any doubts about a method, consult textbooks, scholarly articles, online resources, or even your instructors or peers. Also, keep in mind that using the most complicated method isn't always the best option. Simplier models are frequently more interpretable, robust, and less prone to overfitting.
Failure to Validate and Test Models:
Another common error is to fail to validate and test your models. After creating a model, it is critical to assess its performance and dependability. Without proper validation, your model may overfit the training data, performing well on it but poorly on new, unknown data. The model's utility and predictive power suffer as a result of its lack of generalizability.
Always divide your data into a training set and a testing set to ensure the robustness of your model. Create your model with the training set, then test it on the testing set to see how it performs. For classification models, metrics such as accuracy, precision, recall, F1 score, and area under the ROC curve can be used, while regression models can use R-squared, adjusted R-squared, root mean squared error, and mean absolute error. If your model is underperforming, consider changing its parameters or trying a different model entirely.
Overlooking Data Visualization's Importance:
Many students underestimate the importance of data visualization in data analysis. Using well-designed charts and graphs to present your findings can improve understanding and make your conclusions more compelling. Many students, however, either ignore this aspect entirely or produce subpar visualizations that fail to effectively communicate their results.
Avoid making this error by including appropriate data visualizations in your assignments. This includes, among other things, bar charts, pie charts, line graphs, scatter plots, and heatmaps. Choose wisely because each type of visualization serves a specific purpose and is appropriate for specific types of data. Furthermore, make sure your visualizations are clear and easy to understand, with properly labeled axes, legends, and titles. Remember that your goal is to communicate your findings in the most direct and impactful way possible.
Inadequate Result Interpretation and Reporting:
The final error to avoid is poor interpretation and reporting of results. Data analysis and results are only half the battle; the other half involves correctly interpreting these results and effectively communicating them to your audience. Many students falter at this stage, offering superficial interpretations or failing to articulate their findings thoroughly.
To avoid this trap, always interpret your findings in light of your problem statement and research question. Discuss the significance of your findings, how they answer your research question, and the implications they have. Be sure to mention any limitations or potential sources of bias. Furthermore, organize your report logically and coherently, and write in clear, concise language that your audience will understand. Remember that the goal of your assignment is not just to analyze data, but to communicate the results and their significance to your audience.
Conclusion:
Data analysis is a multifaceted process that necessitates close attention and comprehension at each stage. By avoiding these common errors, you can improve the quality of your data analysis assignments and build a strong analytical skill set that will serve you well in your academic and professional endeavors. Remember that each assignment is an opportunity to learn, so embrace your mistakes and learn from them.