- Introduction to Multilevel Modeling
- Hierarchical Structure in Data
- Advantages of Multilevel Modeling
- SPSS as a Tool for Multilevel Modeling
- Getting Started with Multilevel Modeling in SPSS
- Data Preparation and Structure
- Model Specification in SPSS
- Estimation and Model Fit
- Interpreting Multilevel Model Results in SPSS
- Fixed and Random Effects
- Variance Partition Coefficients
- Post Hoc Analyses and Subgroup Effects
- Advanced Topics in Multilevel Modeling with SPSS
- Cross-Level Interactions
- Model Comparison and Selection
- Handling Missing Data and Robustness Checks
- Conclusion
Multilevel modeling (MLM) stands as a formidable statistical approach, offering researchers a robust framework to dissect data characterized by hierarchical structures. Hierarchical structures denote situations where individual observations are not independent but are instead organized into higher-level units or clusters. This organizational hierarchy is pervasive across diverse fields, ranging from educational research, where students are nested within classrooms, to healthcare studies, where patients are nested within hospitals. The conventional statistical methods may falter in capturing the complexities inherent in such hierarchical data, leading to biased and inadequate results. However, MLM emerges as a potent solution, acknowledging and accounting for the intricate relationships within these nested structures. In the realm of applied statistics, MLM holds immense significance, representing a paradigm shift in the analytical landscape. Unlike traditional statistical models that treat all observations as independent entities, MLM recognizes the inherent dependencies within hierarchical data. This nuanced approach is particularly pertinent in scenarios where individual observations are not only influenced by their immediate environment but also by the higher-level units to which they belong. For instance, in educational studies, the performance of students may be influenced not just by individual characteristics but also by the characteristics of their respective classrooms or schools. Failure to account for such hierarchical dependencies can result in oversimplified models that fail to capture the true dynamics of the data. For those seeking help with your SPSS homework, understanding the application of MLM in analyzing hierarchical data is crucial for effectively utilizing SPSS software and conducting rigorous statistical analysis.
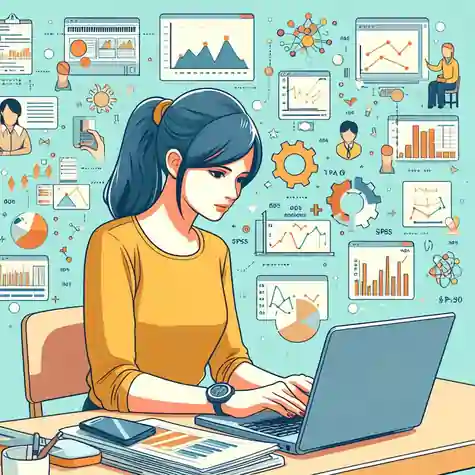
This comprehensive guide aims to demystify the intricacies of multilevel modeling, specifically focusing on its implementation using SPSS (Statistical Package for the Social Sciences). SPSS stands out as a widely used and accessible software tool, making it an ideal platform for students and researchers to delve into the world of multilevel modeling. The guide seeks to empower students by providing not only theoretical insights into MLM but also practical, step-by-step instructions that bridge the gap between theory and application. By doing so, it equips students with the knowledge and skills necessary to navigate the complexities of hierarchical data and extract meaningful insights. The fundamental premise of multilevel modeling lies in acknowledging the dual levels of variation within the data – variation within individual units and variation between higher-level units. Traditional statistical methods often overlook this hierarchical nature, assuming homogeneity across all observations. MLM, on the other hand, introduces the concept of fixed and random effects, recognizing that some factors exert consistent effects across all units (fixed effects), while others contribute to variability at the higher level (random effects). This nuanced modeling allows for a more accurate representation of the underlying data structure, capturing both individual idiosyncrasies and overarching patterns.
Introduction to Multilevel Modeling
Multilevel modeling (MLM), also recognized as hierarchical linear modeling or mixed-effects modeling, stands as a sophisticated statistical method tailored for managing nested data structures. The conventional linear regression techniques often fall short when handling datasets with intricate hierarchies, where observations are grouped within higher-level units. MLM distinguishes itself by acknowledging the existence of multiple levels of variation within the data, proving its efficacy in the analysis of complex datasets.
Hierarchical Structure in Data
At the core of multilevel modeling lies the acknowledgment of hierarchical structures inherent in many datasets. This hierarchy manifests as levels or clusters within the data, wherein individual observations are nested within higher-level units. Consider, for instance, students nested within classrooms or employees within organizations. Traditional statistical methods may inadvertently overlook the dependencies within these clusters, leading to biased results.
Multilevel modeling addresses this limitation by explicitly considering the hierarchical arrangement of data. By recognizing and accounting for the inherent variability at different levels, MLM provides a more accurate representation of the true relationships within the dataset. This is particularly crucial in scenarios where the grouping of observations introduces additional layers of complexity that traditional models fail to capture.
Advantages of Multilevel Modeling
The advantages of multilevel modeling extend beyond its capacity to handle hierarchical data structures. One of its key strengths lies in its ability to account for both within-group and between-group variations. This distinctive feature makes multilevel modeling particularly valuable in various domains, including educational research, clinical studies, and organizational behavior analysis.
In educational research, for example, multilevel modeling allows researchers to explore not only individual student-level effects but also the impact of higher-level factors such as classrooms or schools. Similarly, in clinical studies, where patients are nested within medical practices or hospitals, multilevel modeling can disentangle individual patient outcomes from the effects of the larger medical context.
SPSS as a Tool for Multilevel Modeling
In the realm of statistical analysis, the choice of software is pivotal. SPSS, or the Statistical Package for the Social Sciences, emerges as a popular and versatile tool for researchers and students alike. When it comes to multilevel modeling, SPSS offers a user-friendly interface and robust features that streamline the analytical process.
This guide emphasizes the use of SPSS for conducting multilevel analyses, providing practical tips and step-by-step instructions. Empowering students with the knowledge of utilizing SPSS for multilevel modeling not only facilitates the application of this advanced statistical technique but also enhances their overall analytical skill set. SPSS's capabilities make it an ideal companion for those navigating the complexities of multilevel modeling, ensuring that users can leverage its features to derive meaningful insights from their data.
Getting Started with Multilevel Modeling in SPSS
Getting started with multilevel modeling in SPSS requires a systematic approach, beginning with the crucial steps of data preparation and structure. This section serves as a foundational guide, equipping users with the essential knowledge to set the stage for a successful multilevel analysis.
Data Preparation and Structure
The journey into multilevel modeling starts with meticulous data preparation. A well-organized dataset is the cornerstone of any statistical analysis, and this holds true for multilevel modeling in SPSS. The hierarchical structure inherent in multilevel data necessitates a clear delineation of different levels, such as individuals nested within groups or students within classrooms.
In SPSS, users must structure their data with precision, designating the appropriate variables as level-1 and level-2 units. Level-1 units typically represent individual observations, while level-2 units denote the higher-level clusters to which these observations belong. This classification is vital for accurately capturing the hierarchical relationships within the dataset.
Model Specification in SPSS
Once the data is prepared and structured appropriately, the next step is model specification in SPSS. This involves defining the multilevel model by specifying fixed and random effects and selecting the appropriate covariance structure. SPSS provides a user-friendly interface for this critical phase, offering a range of options to tailor the analysis to the specific research question at hand.
Defining fixed effects involves identifying the predictors that are expected to have a consistent impact across all levels. These are the variables of interest that help uncover general trends or associations within the dataset. On the other hand, random effects capture variations at different levels, acknowledging that not all effects are uniform across all clusters.
Estimation and Model Fit
After specifying the multilevel model, SPSS employs advanced estimation techniques to calculate the model parameters. Understanding the principles of estimation is vital for obtaining reliable results. Additionally, assessing the model fit ensures that your chosen model accurately represents the underlying patterns in the data.
SPSS equips users with various diagnostics and fit indices to evaluate the adequacy of the multilevel model. These indicators help researchers gauge how well the model aligns with the observed data, allowing for informed decisions about its suitability. By comprehensively exploring the estimation process and model fit in SPSS, researchers can ensure the robustness and validity of their multilevel analyses.
Interpreting Multilevel Model Results in SPSS
Interpreting the results of a multilevel model in SPSS is a crucial phase in the analytical process, demanding a careful and nuanced understanding of the output tables and graphs generated by the software. This section will delve into the key components of the multilevel model results and guide you through the interpretation process.
Fixed and Random Effects
The multilevel model output in SPSS presents two fundamental types of effects: fixed and random. Distinguishing between these effects is vital for accurately interpreting the impact of predictors on the outcome variable. Fixed effects capture population-level relationships, representing the average impact of variables across all levels. For example, in an educational context, a fixed effect might signify the average effect of a teaching method across all classrooms.
On the other hand, random effects account for variations at different levels of the hierarchy. In the educational example, random effects would capture the variability in teaching methods' impact between different classrooms. SPSS conveniently provides clear summaries of both fixed and random effects, offering researchers a comprehensive view of the relationships within and between levels. Understanding and appropriately interpreting these effects is essential for drawing accurate conclusions from multilevel analyses.
Variance Partition Coefficients
An integral aspect of multilevel modeling is the partitioning of variance at different levels, and SPSS calculates variance partition coefficients to quantify this distribution. These coefficients reveal the proportion of total variance attributable to each level, providing insights into the hierarchical structure of the data. For instance, in a study examining student performance nested within classrooms, variance partition coefficients elucidate the extent to which performance variability exists within individual students compared to variations between classrooms.
Interpreting these coefficients allows researchers to gauge the relative importance of different levels in explaining the overall variability in the outcome variable. High variance at a specific level indicates that the corresponding level significantly influences the observed variability. Researchers can leverage this information to tailor interventions or focus on specific levels where the greatest impact can be achieved.
Post Hoc Analyses and Subgroup Effects
In some research scenarios, delving deeper into specific subgroup effects or conducting post hoc analyses becomes imperative. SPSS empowers researchers to perform additional tests and comparisons to uncover nuanced patterns within the multilevel data. Post hoc analyses enable exploration beyond the primary relationships examined in the model, shedding light on interactions and variations that may not be immediately apparent.
Researchers utilizing SPSS for post hoc analyses can conduct pairwise comparisons, test specific hypotheses, or explore interactions between variables at different levels. This flexibility is invaluable when investigating complex phenomena with multifaceted relationships. The guide will walk you through the step-by-step process of conducting post hoc analyses in SPSS, ensuring that you can extract meaningful insights and refine your understanding of the intricate patterns and relationships within your multilevel data.
Advanced Topics in Multilevel Modeling with SPSS
As researchers advance in their understanding of multilevel modeling, they inevitably encounter complex scenarios that demand sophisticated techniques. In this section, we'll explore three advanced topics within multilevel modeling using SPSS: Cross-Level Interactions, Model Comparison and Selection, and Handling Missing Data and Robustness Checks.
Cross-Level Interactions
Cross-level interactions add a layer of complexity to multilevel modeling by examining how the relationship between variables varies across different levels of analysis. In SPSS, researchers can delve into testing and interpreting these interactions, gaining deeper insights into the interplay between individual and group-level factors.
Identifying cross-level interactions involves assessing whether the impact of a predictor variable on the outcome variable differs across the levels of another variable. SPSS facilitates this process by allowing users to specify interaction terms in their multilevel models. Through step-by-step guidance, researchers can navigate SPSS to uncover nuanced relationships within their data. Understanding cross-level interactions is invaluable, as it enables researchers to discern whether certain factors exert differential effects on outcomes at individual and group levels.
Model Comparison and Selection
Selecting the most appropriate multilevel model is a critical aspect of the analysis, and SPSS provides tools to aid researchers in this decision-making process. Model comparison involves assessing the fit of different models to the data, and SPSS offers a range of statistical indices and measures to evaluate model performance.
Researchers can employ techniques such as likelihood ratio tests, Akaike Information Criterion (AIC), and Bayesian Information Criterion (BIC) in SPSS to compare models and make informed decisions about model complexity. Through our guide, we will walk you through the step-by-step process of model comparison, equipping you with the skills to choose the model that best captures the underlying patterns in your data. This advanced topic ensures that researchers not only build models that fit their data well but also avoid overfitting by selecting models that generalize to new data.
Handling Missing Data and Robustness Checks
Real-world datasets are often plagued by missing values, and addressing this issue is crucial for the validity of multilevel modeling results. SPSS provides researchers with options for handling missing data, and we will explore strategies such as imputation and sensitivity analyses to maintain the reliability of analyses in the face of incomplete data.
Imputation methods in SPSS, such as mean imputation or multiple imputation, allow researchers to fill in missing values, preserving the integrity of the dataset. Additionally, conducting robustness checks ensures the reliability of multilevel analyses. By systematically testing the sensitivity of results to changes in model specifications or handling of missing data, researchers can enhance the robustness and generalizability of their findings.
Conclusion
In conclusion, mastering multilevel modeling in SPSS opens up a world of possibilities for researchers in various fields. This guide has provided a comprehensive overview, from the basics of hierarchical data structure to advanced techniques for handling complex research questions. Armed with this knowledge, students can confidently approach their assignments, utilizing SPSS to uncover meaningful insights within the intricate layers of their data. As the field of applied statistics continues to evolve, a solid understanding of multilevel modeling will undoubtedly be a valuable asset for aspiring researchers and analysts.