- The Basics of Multivariate Analysis in Excel
- Understanding Multivariate Analysis
- Excel Functions for Multivariate Analysis
- Exploring Regression Analysis in Excel
- The Essence of Regression Analysis
- Regression Output Interpretation
- Principal Component Analysis (PCA) in Excel
- Introduction to Principal Component Analysis
- Interpreting PCA Results
- Cluster Analysis Using Excel
- Unraveling Patterns with Cluster Analysis
- Interpreting Cluster Analysis Results
- Conclusion
In the dynamic field of statistical analysis, the significance of multivariate analysis cannot be overstated. This methodological approach stands as a cornerstone in unraveling intricate relationships within datasets, offering a profound understanding of how multiple variables interact and influence one another. For students venturing into the expansive landscape of data analytics, the ability to perform multivariate analysis in Excel becomes a pivotal skill set. Excel, a ubiquitous tool in academic and professional settings, serves as an accessible and versatile platform for students to explore and apply the principles of multivariate analysis. Multivariate analysis, at its core, is a statistical technique designed to handle the complexity inherent in datasets containing multiple variables. It goes beyond the limitations of univariate analysis, which focuses on a single variable, by simultaneously examining the relationships and interdependencies among several variables. The synergy among these variables is crucial for gaining a comprehensive understanding of the intricate dynamics within a dataset. Through the lens of multivariate analysis, students can uncover patterns, correlations, and trends that might remain obscured when analyzing variables in isolation. If you need help with your Excel homework, mastering multivariate analysis in Excel is essential for your data analytics tasks, allowing you to unlock deeper insights and make informed decisions based on complex datasets.
Excel, with its user-friendly interface and widespread use, emerges as an ideal environment for students to delve into multivariate analysis. The software provides an array of functions and features that streamline the process, making it accessible even to those in the early stages of their statistical journey. Functions like AVERAGE and STDEV allow for the calculation of means and standard deviations, while more advanced tools like CORREL and COVARIANCE facilitate the exploration of relationships and covariances between variables.
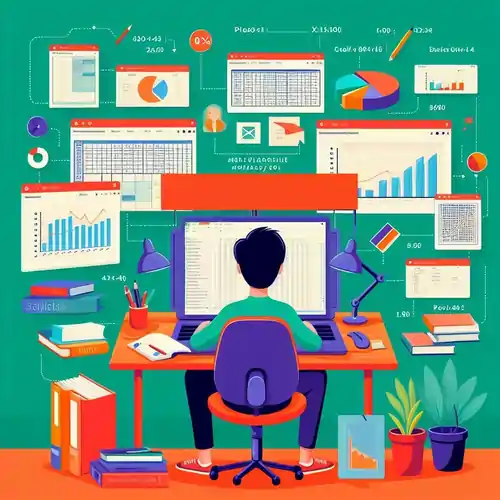
The seamless integration of these functions empowers students to perform complex analyses with relative ease, providing them with a practical and hands-on experience in statistical exploration. As students embark on their journey through the fundamentals of multivariate analysis, they gain a roadmap that guides them through the intricacies of the process. This roadmap becomes invaluable when tackling assignments, as it not only elucidates the theoretical underpinnings of multivariate analysis but also provides practical insights into its application using Excel. By systematically navigating through the steps and concepts, students can build a solid foundation, enhancing their ability to analyze diverse datasets and draw meaningful conclusions.
The Basics of Multivariate Analysis in Excel
Multivariate analysis, a cornerstone of statistical exploration, becomes a formidable ally when harnessed through the capabilities of Microsoft Excel. As students embark on the journey of deciphering complex datasets and unveiling intricate relationships within them, a solid foundation in the basics of multivariate analysis is indispensable. This section will guide you through the fundamental aspects, starting with a conceptual understanding and progressing to the practical implementation using Excel.
Understanding Multivariate Analysis
At its core, multivariate analysis stands in stark contrast to univariate analysis by expanding the scope of investigation. While univariate analysis focuses on a single variable, multivariate analysis juggles multiple variables simultaneously. This broader perspective enables a more nuanced comprehension of complex relationships within datasets, making it an indispensable tool in fields ranging from economics to biology. Imagine you are analyzing a dataset that includes variables such as income, education level, and geographic location. Univariate analysis could provide insights into each variable individually, but it may fall short in revealing the intricate interplay between these factors. This is where multivariate analysis steps in, allowing you to explore how changes in one variable correlate with changes in others.
Excel, being a ubiquitous spreadsheet software, acts as a versatile canvas for conducting multivariate analysis. Its user-friendly interface empowers students to input and manipulate variables effortlessly. By leveraging Excel's capabilities, you can perform a variety of analyses that provide a holistic view of your dataset. Whether you are a novice or an experienced user, Excel's intuitive environment ensures that you can quickly adapt to the intricacies of multivariate analysis. This not only streamlines the analytical process but also enhances your ability to derive meaningful insights from diverse datasets.
Excel Functions for Multivariate Analysis
Excel, with its user-friendly interface and versatile functionality, provides an ideal platform for students to apply multivariate analysis to real-world problems. The journey begins with a mastery of fundamental Excel functions, paving the way for more sophisticated analyses. Let's delve into some key functions that form the bedrock of multivariate analysis in Excel. The journey into multivariate analysis often starts with basic statistical measures. Excel's AVERAGE function calculates the mean of a set of values, providing a central measure around which data points cluster. Complementing this, the STDEV function calculates the standard deviation, offering insights into the spread or dispersion of data. These functions serve as the building blocks for understanding the central tendencies and variabilities within multivariate datasets.
As students progress, they encounter more sophisticated tools in Excel's arsenal. The CORREL function computes the correlation coefficient between two sets of data, revealing the strength and direction of their relationship. On the other hand, the COVARIANCE function provides a quantitative measure of how much two variables change together. These tools are pivotal in deciphering the complex interplay between multiple variables, guiding students towards a nuanced interpretation of relationships within datasets. Familiarity with these functions is akin to acquiring a set of analytical instruments. Armed with the ability to calculate means, standard deviations, correlations, and covariances, students are empowered to unravel the intricacies of multivariate datasets. This foundational knowledge serves as the bedrock upon which more advanced multivariate analyses can be constructed.
Exploring Regression Analysis in Excel
Regression analysis is a fundamental statistical method used to examine the relationship between one dependent variable and one or more independent variables. In Excel, this technique is made accessible to students through user-friendly tools that facilitate the modeling of relationships between variables, enabling predictive analysis and comprehension of the strength of these associations.
The Essence of Regression Analysis
At its core, regression analysis in Excel allows users to explore the connections between variables. It's a powerful tool for modeling how changes in one variable are associated with changes in another, which is invaluable in making predictions. Excel simplifies this process by offering built-in regression tools that support various types of regression: linear, multiple, and logistic.
- Linear Regression: Excel's linear regression tools are particularly useful in establishing a relationship between two variables that appear to have a linear trend. By fitting a line to the data points, students can predict the value of the dependent variable based on changes in the independent variable.
- Multiple Regression: For scenarios involving more than one independent variable, Excel enables students to perform multiple regression analysis effortlessly. This method helps determine how several predictors together influence the dependent variable.
- Logistic Regression: When dealing with categorical outcomes, such as 'yes' or 'no' or 'success' and 'failure,' Excel's logistic regression tools are invaluable. This type of regression assesses the probability of a certain event occurring based on the input variables.
Regression Output Interpretation
Upon completing a regression analysis in Excel, interpreting the output becomes pivotal in drawing meaningful conclusions from the analysis. Excel generates a comprehensive output that includes various components crucial for analysis:
- Coefficients: These values indicate the slopes of the regression line(s) and provide insights into the strength and direction of the relationships between variables. They illustrate how much the dependent variable is expected to change when the independent variable changes by one unit while holding other variables constant.
- P-values: P-values assess the statistical significance of the coefficients. Understanding these values is essential in determining if the relationships observed are likely due to chance or if they are genuinely significant.
- R-squared Values: The R-squared value represents the proportion of the variance in the dependent variable that is explained by the independent variable(s). It ranges from 0 to 1, with higher values indicating a better fit of the model to the data.
Principal Component Analysis (PCA) in Excel
Principal Component Analysis (PCA) stands as a formidable technique in the realm of data analysis, particularly for dealing with high-dimensional datasets. Its primary goal is to reduce the dimensionality of data while retaining as much of the original variability as possible. In the context of Excel, a widely accessible and user-friendly tool, the implementation of PCA is facilitated through the Data Analysis ToolPak, offering students a streamlined process for tackling complex datasets.
Introduction to Principal Component Analysis
At its core, Principal Component Analysis is a mathematical procedure that transforms a set of correlated variables into a set of uncorrelated variables, known as principal components. These components are linear combinations of the original variables and are arranged in descending order of variance. The first principal component captures the maximum variance in the data, with subsequent components representing decreasing amounts of variance. In the context of Excel, the Data Analysis ToolPak simplifies the application of PCA. To access the ToolPak, users need to navigate to the "Data" tab, locate the "Data Analysis" option, and select "Principal Component Analysis."
Once activated, the PCA tool prompts users to input the range of their data, allowing for a seamless transformation of complex datasets into a more manageable form. The essence of PCA lies in its ability to condense information while preserving the most critical aspects of the data. By reducing the number of variables, PCA facilitates a clearer understanding of the underlying structure and relationships within the dataset. This is particularly beneficial when dealing with datasets containing numerous variables, as it becomes challenging to analyze and interpret the information effectively.
Interpreting PCA Results
Upon completing the PCA process in Excel, the next crucial step is to interpret the results effectively. The output typically includes eigenvalues, eigenvectors, and the principal components themselves. Eigenvalues represent the amount of variance captured by each principal component. Larger eigenvalues indicate components that retain more information from the original dataset. Students should pay attention to the proportion of total variance explained by each eigenvalue, aiding in the decision of how many principal components to retain.
Eigenvectors, on the other hand, represent the direction of maximum variance in the dataset. Each eigenvector corresponds to a principal component, and the combination of these vectors forms the principal components. Understanding the relationships between the original variables and the principal components is vital for grasping the meaning of the transformation. Interpreting the principal components involves analyzing the weights assigned to each original variable in the creation of the components. These weights indicate the contribution of each variable to the overall principal component. By examining these weights, students can identify which variables have the most significant impact on specific components, providing insights into the structure of the data.
Cluster Analysis Using Excel
Cluster analysis is a powerful statistical technique that allows you to identify patterns and relationships within datasets by grouping similar data points together. In the context of Excel, this analytical method becomes accessible to students, providing a user-friendly platform to delve into the intricacies of clustering. Let's explore how cluster analysis in Excel can be a valuable tool for unraveling complex data structures.
Unraveling Patterns with Cluster Analysis
At its core, cluster analysis involves the segmentation of a dataset into distinct groups or "clusters" based on the similarity of data points within each group. In Excel, this process is facilitated through the Data Analysis ToolPak, a powerful add-in that extends Excel's capabilities. The concept of clustering revolves around the idea that data points within the same cluster share similarities, while those in different clusters exhibit differences. To initiate cluster analysis in Excel, you first need to organize your data appropriately. Identify the variables that are relevant to your analysis and arrange them in a tabular format. Once your data is structured, navigate to the Data tab, select the Data Analysis option, and choose "Cluster Analysis" from the list of tools available in the ToolPak.
Excel's Cluster Analysis tool prompts you to specify the range of your dataset and select the variables you want to include in the analysis. Additionally, you can define the number of clusters you want the algorithm to identify. Excel employs the k-means clustering algorithm, which iteratively partitions the data into clusters based on similarities until the specified number of clusters is reached. As the algorithm runs, Excel dynamically updates the clusters, allowing you to observe the evolving groupings in real-time. Once the analysis is complete, Excel generates a summary report that includes the cluster assignments for each data point. This report is a valuable resource for understanding the structure of your data and identifying inherent patterns.
Interpreting Cluster Analysis Results
Identifying clusters is only the first step; the real value lies in interpreting the results to extract meaningful insights. Excel simplifies this process by providing visualizations and summaries that aid in comprehending the structure of the clusters. The generated report typically includes visual representations such as scatter plots or dendrogram charts, illustrating the relationships between data points within and across clusters. These visuals serve as powerful tools for grasping the underlying patterns in your data.
Moreover, Excel provides statistical summaries for each cluster, showcasing the average values of variables within each group. This information allows you to discern the characteristics that define each cluster and understand how they differ from one another. Interpreting cluster analysis results is a dynamic process that involves a combination of quantitative analysis and qualitative understanding. Excel equips you with the necessary tools to delve into the intricacies of each cluster, enabling you to draw informed conclusions about the inherent patterns in your dataset.
Conclusion
In conclusion, mastering multivariate analysis in Excel is a valuable skill for students pursuing data analytics or related fields. This guide has provided a comprehensive roadmap, covering the basics of multivariate analysis, regression analysis, principal component analysis, and cluster analysis. By understanding the core concepts and leveraging Excel's powerful tools, you can approach assignments with confidence, unravel complex relationships within datasets, and make informed decisions based on your analyses. As you continue your academic journey, the skills acquired through this roadmap will undoubtedly contribute to your success in the dynamic field of data analysis.