- The Basics of Non-Parametric Tests
- Understanding Non-Parametric Tests
- Advantages and Limitations of Non-Parametric Tests
- Performing Non-Parametric Tests in Excel
- Data Preparation
- Conducting Mann-Whitney U Test in Excel
- Applications of Non-Parametric Tests
- Medical Research Case Study
- Social Sciences Application
- Conclusion
Statistical analysis stands as an indispensable cornerstone in the realm of research across diverse fields, serving as the linchpin for drawing insightful conclusions from complex datasets. It acts as the compass that guides researchers through the intricacies of their findings, providing a systematic and objective framework to interpret the information at hand. Amidst the plethora of statistical methods, parametric tests have long been the conventional choice, relying on assumptions such as normality and homogeneity of variances. However, when these assumptions are untenable due to the nature of the data or the specific circumstances of a study, non-parametric tests emerge as a formidable alternative. Non-parametric tests, in essence, offer a robust and flexible approach to statistical analysis. Unlike their parametric counterparts, they make minimal assumptions about the underlying distribution of the data, rendering them particularly useful in scenarios where these assumptions cannot be met. This becomes particularly relevant in real-world research, where datasets often deviate from the idealized normal distribution. Non-parametric tests, therefore, provide a more versatile toolkit, accommodating a broader range of data types, including ordinal and nominal data, which might be prevalent in fields such as social sciences and healthcare. For students embarking on the challenging journey into the world of statistics, the mastery of non-parametric tests becomes a crucial skill set. These tests not only expand the analytical horizon but also offer a safety net when confronted with data that doesn't conform to the stringent assumptions of parametric tests. Whether you need help with your Excel homework or are looking to enhance your understanding of statistical analysis, grasping the nuances of non-parametric tests can significantly enrich your analytical toolkit.
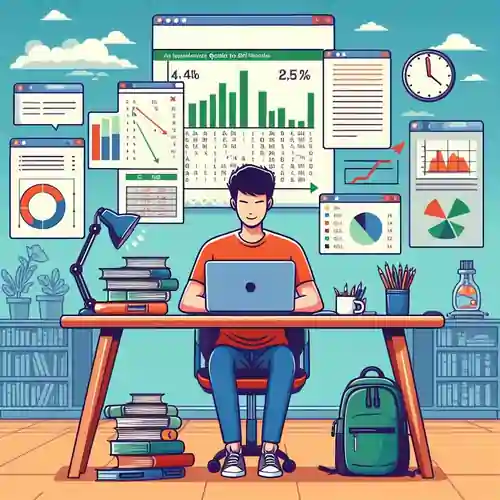
Nevertheless, navigating the intricacies of non-parametric tests can be daunting, especially for those in the early stages of their statistical education. It is this challenge that the current introductory guide aims to address, acting as a beacon to demystify the complexities associated with non-parametric tests. The focus of this guide extends beyond mere theoretical understanding, aiming to provide practical insights into the application of non-parametric tests. Microsoft Excel, a ubiquitous and accessible tool, is chosen as the vehicle for this journey. Excel, with its familiar interface and extensive functionalities, serves as an excellent platform for students to bridge the gap between theory and application. By demonstrating how non-parametric tests can be executed in Excel, the guide empowers students to apply their statistical knowledge to real-world scenarios effectively. The decision to employ Microsoft Excel as the chosen tool is intentional, recognizing its widespread availability and ease of use. This makes the guide not only informative but also practical for students who may have varying levels of statistical and technical expertise. The step-by-step instructions provided in the guide offer a scaffolded approach, ensuring that students can follow along and implement the techniques without feeling overwhelmed.
The Basics of Non-Parametric Tests
Non-parametric tests form a critical category of statistical procedures renowned for their flexibility and broad applicability. In contrast to their parametric counterparts, non-parametric tests refrain from imposing stringent assumptions on the underlying population distribution from which the data is derived. This characteristic liberates these tests, making them suitable for a diverse array of data types and scenarios. Unlike parametric tests that hinge on assumptions of normality and homogeneity of variances, non-parametric tests provide a robust alternative, ensuring reliable results even when such assumptions are not met. In this section, we embark on a journey to unravel the fundamental concepts of non-parametric tests, shedding light on their utility and significance in statistical analysis.
Understanding Non-Parametric Tests
Non-parametric tests emerge as indispensable tools in statistical analysis, particularly when confronted with specific data characteristics or research scenarios. One of the primary domains where non-parametric tests shine is in the analysis of ordinal or nominal data, where the traditional assumptions of parametric tests may not hold. Furthermore, non-parametric tests exhibit a remarkable capability to handle outliers gracefully, making them resilient in the face of data anomalies. Their utility extends to situations involving small sample sizes, where the robustness of non-parametric tests becomes particularly advantageous.
Within the realm of non-parametric tests, there exists a rich assortment of statistical tools, each tailored to address distinct research questions. Among the prominent examples are the Mann-Whitney U test, Wilcoxon signed-rank test, and Kruskal-Wallis test. These tests cater to different study designs and data structures, providing researchers with a versatile toolkit for conducting statistical inference. As we delve into each of these tests, we not only unravel their mathematical underpinnings but also illuminate their specific use cases and the nuanced interpretation of results. This exploration aims to empower students with a nuanced understanding of non-parametric tests, enabling them to discern when and why these tests are the optimal choice for their analytical endeavors.
Advantages and Limitations of Non-Parametric Tests
While the advantages of non-parametric tests are evident, a comprehensive understanding requires acknowledgment of both their strengths and limitations. One of the notable advantages is the remarkable flexibility these tests offer, especially in the face of non-normally distributed data. The resilience to outliers further enhances their robustness in real-world scenarios where data anomalies are not uncommon.
However, it is essential to recognize that non-parametric tests may come with a trade-off. Despite their flexibility, they might exhibit less statistical power compared to parametric tests under certain conditions. This limitation implies that non-parametric tests might be less sensitive in detecting subtle differences or patterns in the data, particularly when sample sizes are large. Therefore, a careful consideration of the specific characteristics of the dataset and the research question is imperative when deciding between parametric and non-parametric tests.
Performing Non-Parametric Tests in Excel
In the realm of statistical analysis, Microsoft Excel emerges as a formidable ally, offering a user-friendly interface that facilitates a wide array of analyses, including non-parametric tests. This section aims to unravel the potential of Excel as a robust tool for statistical exploration, guiding students through the intricate process of performing non-parametric tests with a focus on step-by-step procedures and critical considerations.
Data Preparation
Before embarking on the journey of non-parametric tests, meticulous data preparation stands as the foundational step. Recognizing the significance of organized and formatted data, this subsection illuminates the crucial role played by Excel's versatile functions in sorting, filtering, and managing datasets. These functions not only enhance the accessibility of data but also lay the groundwork for accurate and reliable statistical analyses.
Excel's user-friendly features empower students to organize datasets with ease, ensuring that variables are correctly labeled and values are appropriately formatted. The significance of this preparatory phase cannot be overstated, as it mitigates the risk of errors and ensures that subsequent analyses are based on a solid data foundation. By delving into the intricacies of data preparation, students are equipped with the skills needed to navigate the complexities of real-world datasets.
Conducting Mann-Whitney U Test in Excel
As a quintessential non-parametric test for comparing two independent samples, the Mann-Whitney U test holds a prominent place in statistical analyses. This subsection serves as a comprehensive guide, offering students a detailed walkthrough on how to execute the Mann-Whitney U test within the Excel environment. The journey begins with an exploration of the underlying principles of the Mann-Whitney U test, providing students with a solid theoretical foundation. Moving seamlessly from theory to practice, the guide then delves into the practical aspects of executing the test in Excel. Clear and concise instructions, accompanied by illustrative examples, ensure that students not only grasp the mechanics of the test but also gain a nuanced understanding of its application.
Crucially, the guide elucidates the specific Excel formulas required for the Mann-Whitney U test, demystifying the numerical intricacies involved. Through practical examples, students learn to input their data into Excel, perform the test, and interpret the results effectively. The emphasis on interpretation ensures that students not only navigate the technical aspects of the test but also comprehend the meaningful insights it can yield.
Applications of Non-Parametric Tests
Non-parametric tests are invaluable tools in statistical analysis, finding applications in diverse research scenarios across various fields. This section delves into real-world examples, shedding light on how non-parametric tests play a pivotal role in different contexts, underlining their versatility and relevance.
Medical Research Case Study
Medical research is a domain where non-parametric tests emerge as indispensable instruments, particularly when dealing with patient data that does not conform to a normal distribution. In this subsection, we will delve into a compelling case study, illustrating the critical role non-parametric tests played in the analysis of clinical trial data. The significance lies not just in the application of these tests but also in emphasizing the paramount importance of choosing the right statistical approach in the realm of medical research. Clinical trials, being the cornerstone of evidence-based medicine, generate vast amounts of data. However, the distribution of this data can often be skewed, influenced by various factors such as individual patient variability and the complexity of medical conditions. Traditional parametric tests, which assume a normal distribution, may prove inadequate in such scenarios. Enter non-parametric tests, which offer a robust alternative.
Consider a clinical trial assessing the efficacy of a new drug. Patient responses to the treatment may not conform to a normal distribution due to the variability in individual reactions. Employing non-parametric tests, such as the Wilcoxon signed-rank test for paired samples or the Mann-Whitney U test for independent samples, allows researchers to analyze the data without the constraints imposed by parametric assumptions. Through the lens of this case study, students gain a practical understanding of how non-parametric tests contribute to sound statistical analysis in medical research. The takeaway extends beyond the specific tests used—it underscores the necessity of aligning statistical methods with the unique characteristics of the data at hand.
Social Sciences Application
In the social sciences, where researchers often grapple with qualitative data that may not adhere to parametric assumptions, non-parametric tests emerge as a vital analytical tool. This subsection illuminates a research scenario in the social sciences where non-parametric tests were instrumental in extracting meaningful insights from survey responses, providing valuable perspectives into human behavior. Social science research frequently involves exploring the complexities of human behavior, attitudes, and opinions through surveys. The nature of this data can be inherently qualitative, categorical, or ordinal, deviating from the assumptions of normality required for parametric tests. Non-parametric tests, with their flexibility and ability to handle such data, become an indispensable ally in the social scientist's toolkit.
Consider a study examining public perceptions of a controversial social issue. Survey responses, being subjective and diverse, may not exhibit a normal distribution. Applying non-parametric tests, such as the Chi-square test for independence or the Kruskal-Wallis test for comparing more than two groups, enables researchers to draw meaningful conclusions without imposing unrealistic assumptions on the data. This case study not only highlights the practical utility of non-parametric tests in social science research but also underscores the broader lesson—adapting statistical methodologies to the unique characteristics of the data enhances the reliability and validity of the findings.
Conclusion
In the culmination of this comprehensive guide, the overarching objective has been to furnish statistics students with the essential knowledge and skills requisite for comprehending, executing, and deciphering non-parametric tests utilizing the ubiquitous Microsoft Excel. This endeavor is not merely about acquiring theoretical proficiency but is geared towards the practical application of statistical tools, thereby enabling students to augment their analytical prowess and confront an expansive spectrum of research inquiries. The utility of non-parametric tests extends far beyond the confines of statistical classrooms, proving to be invaluable assets in the dynamic realm of research and data analysis. Adeptness in these tests transcends the theoretical boundaries of statistical knowledge, empowering students to navigate the intricacies of real-world datasets with confidence. Whether immersed in the intricacies of medical data, scrutinizing the nuances of social science surveys, or exploring the multifaceted nature of any dataset, the versatility of non-parametric tests positions them as indispensable components of a burgeoning statistician's toolkit.
In a continuously evolving landscape of research methodologies and analytical techniques, the significance of cultivating a solid foundation in non-parametric tests cannot be overstated. This foundation serves as a catalyst, propelling students towards making informed decisions and deriving reliable conclusions from their research findings. The skills acquired in this guide transcend the conventional boundaries of statistical knowledge, fostering an environment where students evolve into adept researchers capable of addressing the challenges posed by real-world datasets.