- Experimental Design and Sample Size Determination
- Importance of Experimental Design:
- Sample Size Determination:
- Data Collection and Management
- Data Collection Methods:
- Data Management and Cleaning:
- Statistical Analysis and Interpretation
- Statistical Analysis Techniques:
- Interpretation of Results:
- Presentation and Communication of Findings
- Effective Data Visualization:
- Clear and Accessible Reporting:
- Conclusion:
Biostatistics, a branch of statistics specifically focused on biological data and phenomena, serves as an indispensable tool in modern research across diverse fields within biology and related disciplines. Its significance lies in its ability to provide quantitative methods for analyzing complex biological processes, allowing researchers to extract meaningful insights from data and make informed decisions. In this section, we will delve deeper into the multifaceted role of Biostatistics in various aspects of research projects and its pivotal contributions to advancing knowledge in the biological sciences. Firstly, Biostatistics plays a critical role in experimental design, where it aids researchers in planning and executing studies that are both scientifically rigorous and ethically sound. By employing statistical methods, researchers can design experiments that effectively test hypotheses while minimizing biases and confounding variables. This ensures the validity and reliability of the results obtained, laying a solid foundation for drawing meaningful conclusions from the data collected. Moreover, BioStatistics facilitates sample size determination, ensuring that studies are adequately powered to detect significant effects or differences. By calculating the optimal sample size, researchers can maximize the efficiency of their studies and enhance the statistical power, thereby increasing the likelihood of obtaining reliable results. Understanding these principles and methodologies can significantly enhance your ability to analyze biological data and contribute to scientific research in the field and helps to master your Biostatistics homework.
Secondly, Biostatistics is indispensable in the realm of data collection and management, where it ensures the accuracy, integrity, and reliability of biological data. Researchers rely on various data collection methods, such as surveys, experiments, observational studies, and clinical trials, to gather information relevant to their research questions. Biostatisticians play a crucial role in designing data collection protocols and implementing quality control measures to minimize errors and maintain data quality throughout the research process.
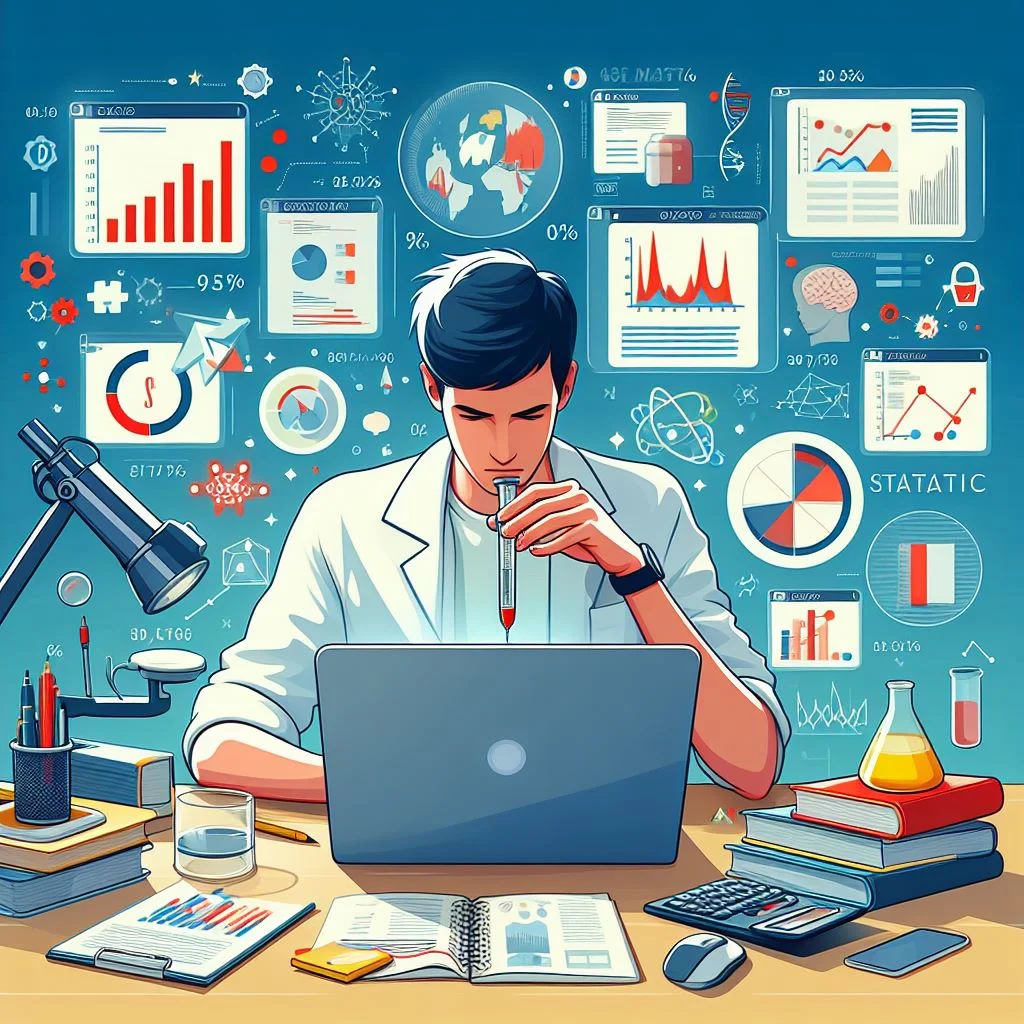
Thirdly, Biostatistics encompasses a wide array of statistical analysis techniques that enable researchers to extract meaningful insights from biological data. From descriptive statistics that summarize and describe the characteristics of data to hypothesis testing that assesses the significance of relationships or differences, Biostatisticians employ a diverse range of methods tailored to the specific research question and study design. Regression analysis, survival analysis, and multivariate analysis are among the advanced statistical techniques used to analyze complex biological data and uncover underlying patterns or relationships. Through statistical analysis, researchers can identify trends, patterns, and associations within biological data, providing valuable insights into biological processes and phenomena. Lastly, Biostatistics plays a crucial role in the presentation and communication of research findings, where it facilitates the effective visualization and dissemination of statistical results. Effective data visualization techniques, such as charts, graphs, and tables, enable researchers to present complex data in a clear and concise manner, enhancing understanding and facilitating interpretation.
Experimental Design and Sample Size Determination
In the realm of Biostatistics, the process of experimental design and sample size determination serves as the foundation upon which meaningful research is built. It is through this meticulous planning that researchers are able to navigate the complexities of biological systems and draw accurate conclusions from their investigations.
Importance of Experimental Design:
Experimental design is akin to the blueprint of a research project, outlining the framework within which data will be collected, analyzed, and interpreted. At its core, it serves to ensure the validity and reliability of the results obtained. By carefully crafting the experimental design, researchers can minimize potential sources of bias, thereby bolstering the credibility of their findings. In Biostatistics, experimental design takes on added significance due to the inherent variability and complexity of biological systems. Researchers utilize experimental design to formulate hypotheses, identify variables of interest, and determine the appropriate methodology for data collection. By establishing clear protocols and controls, researchers can mitigate confounding factors and isolate the effects of specific variables, thus enhancing the robustness of their conclusions.
Furthermore, proper experimental design maximizes efficiency by optimizing the use of resources, such as time, funding, and manpower. By strategically planning the experimental procedures, researchers can streamline the data collection process and ensure that resources are allocated effectively. This not only facilitates the timely completion of the research project but also enhances the overall productivity of the scientific endeavor. Moreover, experimental design plays a pivotal role in enhancing the statistical power of the study. Statistical power refers to the probability of detecting a true effect or difference when it exists.
Sample Size Determination:
Determining the appropriate sample size is a critical aspect of Biostatistics that directly impacts the validity and reliability of research findings. In essence, sample size determination entails striking a delicate balance between statistical precision and practical feasibility. Biostatisticians employ various methods to ascertain the optimal sample size for their research projects, with the overarching goal of ensuring sufficient statistical power to detect meaningful effects or differences. One commonly used approach is power analysis, which involves calculating the statistical power of the study based on factors such as effect size, significance level, and sample variability. By conducting power analyses, researchers can determine the minimum sample size required to achieve a desired level of statistical power, thereby guiding the recruitment of study participants.
Additionally, researchers utilize sample size calculations to estimate the sample size needed to detect a specified effect size with a given level of confidence. This involves considering factors such as the anticipated effect size, desired level of significance, and expected variability within the sample population. By employing statistical formulas and software tools, researchers can derive precise estimates of the sample size required to achieve their research objectives. Adequate sample size is paramount in Biostatistics as it directly influences the reliability and generalizability of research findings. Insufficient sample sizes can lead to underpowered studies, wherein researchers may fail to detect true effects or differences due to inadequate sample representation. Conversely, excessively large sample sizes may result in unnecessary resource expenditure and logistical challenges.
Data Collection and Management
In the realm of Biostatistics, the process of data collection and management is foundational to the success and integrity of any research endeavor. This multifaceted aspect involves not only the collection of data but also its organization, storage, documentation, and eventual preparation for analysis. Let's delve deeper into the intricacies of data collection methods and the importance of data management and cleaning in the field of Biostatistics.
Data Collection Methods:
Data collection methods in Biostatistics are diverse and adaptable, tailored to suit the specific requirements of each research project and the nature of the data being collected. One of the most common methods employed is through surveys, where researchers gather information directly from individuals or groups through structured questionnaires or interviews. Surveys provide a valuable means of collecting quantitative and qualitative data on various aspects of biological phenomena, such as health behaviors, patient experiences, or environmental factors. Another prevalent method is experimental studies, where researchers manipulate variables under controlled conditions to observe their effects on biological systems. These studies, often conducted in laboratory settings, allow for rigorous testing of hypotheses and the establishment of causal relationships between variables. Observational studies, on the other hand, involve the passive observation of subjects in their natural environment, without intervention from the researcher. These studies are particularly useful in exploring associations between variables or identifying risk factors for diseases.
Clinical trials represent a specialized form of experimental study conducted in the field of medicine and healthcare. These trials evaluate the efficacy and safety of medical interventions, such as drugs, vaccines, or medical devices, through carefully designed protocols involving human participants. Clinical trials are essential for advancing medical knowledge and informing clinical practice. Retrospective studies, also known as historical studies or retrospective chart reviews, involve the analysis of existing data collected from medical records, databases, or archives.
Data Management and Cleaning:
Once data is collected, it undergoes a series of steps to prepare it for analysis. Data management involves the systematic organization, storage, and documentation of data to facilitate easy retrieval and analysis. BioStatisticians utilize various tools and techniques to manage data effectively, including database management systems, spreadsheet software, and statistical programming languages. Furthermore, data cleaning is a crucial step in the data preparation process, aimed at identifying and rectifying errors or inconsistencies in the dataset. Biostatisticians employ a range of techniques to clean and preprocess data, ensuring that it is accurate, complete, and suitable for analysis.
This includes identifying and correcting data entry errors, handling missing values through imputation or deletion, and detecting and addressing outliers or anomalies in the data. Proper data management and cleaning are essential for ensuring the accuracy and reliability of statistical analyses. By maintaining high data quality standards, Biostatisticians can mitigate the risk of biased or erroneous results, ultimately enhancing the validity and robustness of research findings in the field of Biostatistics.
Statistical Analysis and Interpretation
Statistical analysis is a cornerstone of Biostatistics, enabling researchers to extract valuable insights from biological data and make informed decisions. Biostatisticians utilize a diverse array of statistical analysis techniques tailored to the specific characteristics of the data and the research objectives.
Statistical Analysis Techniques:
In the realm of Biostatistics, various statistical analysis techniques are employed to explore and understand biological phenomena. Descriptive statistics serve as the initial step in data analysis, providing summaries and visual representations that elucidate key characteristics of the data, such as central tendency, variability, and distribution. This includes measures like mean, median, mode, variance, and standard deviation, along with graphical representations like histograms, box plots, and scatter plots. Hypothesis testing is another crucial statistical tool utilized by Biostatisticians to assess the significance of observed differences or associations in biological data. By formulating null and alternative hypotheses and conducting appropriate statistical tests, researchers can determine whether the observed results are statistically significant, thereby providing evidence for or against the research hypotheses. Common hypothesis tests in Biostatistics include t-tests, chi-square tests, ANOVA, and non-parametric tests.
Regression analysis is widely employed in Biostatistics to examine the relationship between one or more predictor variables and a response variable of interest. This technique allows researchers to model and quantify the associations between variables, predict outcomes, and identify potential confounding factors. Depending on the nature of the data and the research question, Biostatisticians may utilize simple linear regression, multiple regression, logistic regression, or other regression models to analyze biological data effectively. Survival analysis is a specialized statistical technique commonly used in biomedical research to analyze time-to-event data, such as survival times or time until recurrence of a disease. This method accounts for censoring and time-dependent variables, enabling researchers to estimate survival probabilities, compare survival curves between groups, and identify factors influencing survival outcomes.
Interpretation of Results:
Interpreting statistical results accurately is paramount in Biostatistics, as it forms the cornerstone of deriving meaningful insights and making informed decisions. Biostatisticians wield their expertise to dissect and interpret statistical findings within the context of the research question and study objectives. Upon conducting statistical analyses, Biostatisticians assess the significance of the results to ascertain their relevance and reliability. This involves scrutinizing p-values, confidence intervals, effect sizes, and other relevant metrics to determine the statistical significance of observed effects or associations.
Moreover, Biostatisticians delve beyond mere statistical significance, delving into the practical significance and real-world implications of their findings. They critically evaluate the magnitude and direction of observed effects, identifying meaningful trends or patterns that contribute to advancing knowledge in the field of biology. Furthermore, interpretation of results involves contextualizing statistical findings within the broader scientific landscape. Biostatisticians consider existing literature, theoretical frameworks, and biological plausibility to elucidate the implications of their findings and contribute to the existing body of knowledge.
Presentation and Communication of Findings
In the realm of Biostatistics, the presentation and communication of findings are pivotal aspects that bridge the gap between data analysis and real-world application. This phase involves transforming statistical insights into formats that are comprehensible and impactful to various stakeholders, including fellow researchers, policymakers, healthcare professionals, and the general public. Effective presentation and communication strategies ensure that the significance of the research findings is conveyed accurately and that decisions based on these findings are well-informed and evidence based.
Effective Data Visualization:
Effective data visualization serves as a powerful tool for Biostatisticians to communicate complex statistical findings in a clear and concise manner. By utilizing graphical representations such as charts, graphs, and tables, Biostatisticians can transform raw data into visually appealing formats that facilitate understanding and interpretation. Well-designed visualizations not only enhance the comprehension of statistical trends and patterns but also enable comparisons and correlations to be easily identified. Biostatisticians employ a variety of visualization techniques based on the nature of the data and the intended audience. For instance, bar charts and pie charts are commonly used to represent categorical data, while line graphs and scatter plots are preferred for visualizing trends and relationships in continuous data.
Additionally, heat maps and geographic information systems (GIS) are utilized to display spatial patterns and distributions in geographical data. The choice of visualization technique depends on factors such as the complexity of the data, the research objectives, and the preferences of the target audience. Regardless of the method used, the primary goal of effective data visualization remains consistent: to convey statistical findings in a visually engaging and informative manner that facilitates comprehension and decision-making.
Clear and Accessible Reporting:
Clear and accessible reporting of statistical findings is essential for disseminating research results and fostering transparency in scientific communication. Biostatisticians employ various mediums to communicate their findings, including research papers, reports, presentations, and scientific conferences. Regardless of the medium, clear and accessible reporting involves presenting statistical findings in a structured and comprehensible format that is accessible to a diverse audience. Biostatisticians adhere to established guidelines and standards for reporting statistical analyses, ensuring that their findings are reproducible and accessible to the broader scientific community. This includes providing detailed descriptions of the study design, data collection methods, statistical analysis techniques, and interpretation of results. Additionally, Biostatisticians employ clear and concise language to convey complex statistical concepts, avoiding jargon and technical terms that may be unfamiliar to non-specialists.
In addition to traditional reporting methods, Biostatisticians also utilize emerging technologies and platforms to disseminate their findings. For example, interactive data visualizations, online dashboards, and social media platforms can be employed to engage with diverse audiences and facilitate knowledge sharing and collaboration. Overall, clear and accessible reporting of statistical findings is essential for advancing scientific knowledge, informing decision-making, and fostering collaboration within the scientific community. By adhering to principles of transparency, reproducibility, and accessibility, Biostatisticians play a crucial role in ensuring that statistical findings are communicated effectively and contribute to meaningful advancements in the field of biology and beyond.
Conclusion:
In conclusion, Biostatistics plays a vital role in real-life research projects across various fields of biology. Its practical applications encompass experimental design, data collection and management, statistical analysis and interpretation, and presentation and communication of findings. By understanding and applying these principles, students can effectively solve Biostatistics assignments and contribute to advancements in biological research.