Claim Your Discount Today
Get 10% off on all Statistics Homework at statisticshomeworkhelp.com! This Spring Semester, use code SHHR10OFF to save on assignments like Probability, Regression Analysis, and Hypothesis Testing. Our experts provide accurate solutions with timely delivery to help you excel. Don’t miss out—this limited-time offer won’t last forever. Claim your discount today!
We Accept
- Why Study Recreational Sports Team Participation Statistics?
- What Is SPSS?
- Gathering and Preparing Data for Analysis
- Data Collection Methods
- Data Cleaning and Preparation
- Analyzing Recreational Sports Team Participation Data with SPSS
- Descriptive Statistics
- Inferential Statistics
- Data Visualization
- Advanced Statistical Techniques for Deeper Insights
- Factor Analysis
- Cluster Analysis
- Survival Analysis
- Advanced Regression Techniques
- Ethical Considerations in Sports Data Analysis
- Informed Consent
- Data Anonymization
- Data Security
- Transparent Reporting
- Conclusion
Recreational sports have always held a special place in the hearts of people around the world. They provide a refreshing break from the monotony of daily routines and offer an opportunity to indulge in physical activity, make new friends, and foster a sense of community. It's no wonder that recreational sports teams are so popular, drawing participants from diverse age groups and backgrounds. However, behind the scenes of this enjoyable and healthy pastime lies a fascinating world of statistics that can be both challenging and rewarding to explore. In this blog, we will embark on a journey into the realm of recreational sports team participation statistics using the powerful Statistical Package for the Social Sciences (SPSS) software. Whether you are a student seeking assistance with your SPSS assignment or simply intrigued by the dynamics of recreational sports, this comprehensive guide will provide valuable insights.
Why Study Recreational Sports Team Participation Statistics?
Before we dive into the technicalities, let's understand why delving into recreational sports team participation statistics is not only relevant but also highly valuable. For students who find themselves tasked with SPSS assignments related to this subject, mastering these statistics is not merely a requirement; it's a gateway to a broader understanding of the world of sports. But beyond the academic aspect, this knowledge has practical applications that extend to sports enthusiasts, coaches, and policymakers.
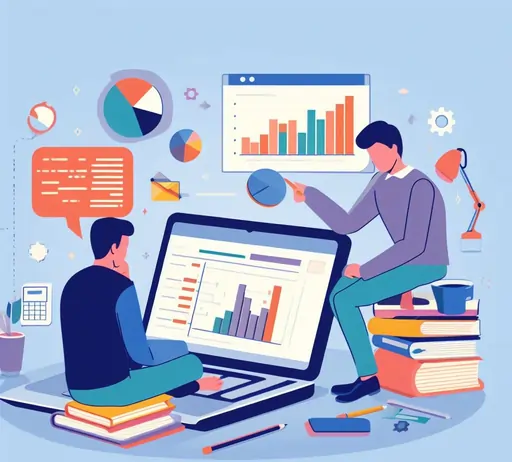
Imagine you're a student struggling to make sense of a complex SPSS assignment centered around recreational sports team participation. The assignment might involve analyzing data on participant demographics, engagement levels, or even factors influencing their choice of sports. This is where a solid understanding of recreational sports statistics, combined with proficiency in using SPSS, becomes your most potent ally. It's the tool that empowers you to not only complete your assignment successfully but also extract meaningful insights from the data.
What Is SPSS?
Before we go any further, let's address the elephant in the room: SPSS. If you're new to this software, it's important to understand what it is and why it's so essential in the world of statistics.
SPSS, short for the Statistical Package for the Social Sciences, is a robust and user-friendly software designed to facilitate data analysis and statistical modeling. It's widely used across various disciplines, including social sciences, business, and healthcare, for its ability to handle both simple and complex data analysis tasks. Its intuitive interface makes it accessible to users with varying levels of statistical expertise, making it an ideal choice for students and professionals alike.
Gathering and Preparing Data for Analysis
Gathering and preparing data for analysis is the essential foundation upon which successful data-driven decision-making and insights are built. In today's data-driven world, organizations across various sectors recognize the paramount importance of collecting, organizing, and refining data to extract meaningful insights. This process is not only crucial but also highly valuable in shaping strategies, identifying trends, and making informed choices.
Data Collection Methods
Before we can begin our statistical analysis, we need data. Data collection methods can vary widely, but when studying recreational sports team participation, several common approaches are typically employed:
- Surveys and Questionnaires: Designing surveys or questionnaires can help gather information about participants' demographics, reasons for joining a team, frequency of participation, and satisfaction levels.
- Observations: Observing sports events, practices, or team activities can provide valuable qualitative data. This might include noting the number of participants, their age groups, or the types of sports played.
- Secondary Data: Utilizing existing data sources, such as participation records from local sports organizations, can save time and resources.
Data Cleaning and Preparation
Once data is collected, it's essential to clean and prepare it for analysis. This involves:
- Data Screening: Identifying and addressing missing or erroneous data points to ensure the dataset is reliable.
- Data Transformation: Converting data into a suitable format for analysis. For instance, categorizing age groups or creating variables for specific sports.
- Data Coding: Assigning numerical values to categorical data for statistical analysis. For example, assigning numbers to gender categories (1 for male, 2 for female).
Analyzing Recreational Sports Team Participation Data with SPSS
Having obtained a pristine dataset, our next step entails delving into a range of essential statistical analyses that can be executed with the aid of SPSS. These analyses are instrumental in shedding light on the dynamics of participation within recreational sports teams:
Descriptive Statistics
Descriptive statistics provide a summary of the main features of the dataset. This includes measures such as mean, median, mode, standard deviation, and variance. Using SPSS, students can easily calculate these statistics to understand the central tendency and variability of factors like age, participation frequency, and satisfaction levels among recreational sports team participants.
Inferential Statistics
Inferential statistics help us draw conclusions and make predictions about a population based on a sample. Here are a few common inferential statistical techniques used in the context of recreational sports team participation:
- Hypothesis Testing: Students can use SPSS to test hypotheses related to recreational sports. For example, they could test whether there is a significant difference in participation frequency between different age groups.
- Regression Analysis: This technique can be used to understand the relationship between various factors, such as age, gender, and satisfaction, and how they affect sports participation. SPSS makes it easy to perform multiple regression analyses.
- Chi-Square Test: When dealing with categorical variables like the choice of sports, the chi-square test can help determine if there is an association between two variables. For example, whether gender and preferred sport are independent of each other among participants.
Data Visualization
SPSS also offers powerful data visualization tools. Students can create various charts and graphs, such as histograms, bar charts, and scatterplots, to visually represent the data. These visuals can aid in presenting findings effectively and making the data more understandable to a broader audience.
Advanced Statistical Techniques for Deeper Insights
While we've covered the fundamentals of recreational sports team participation statistics in the previous sections, it's essential to highlight some advanced statistical techniques that can provide deeper insights into this fascinating field of study. These techniques go beyond basic descriptive and inferential statistics and allow students to explore complex relationships and patterns within the data.
Factor Analysis
Factor analysis is a powerful statistical method used to identify latent factors or underlying dimensions within a dataset. In the context of recreational sports team participation, students can use factor analysis to uncover hidden variables that may influence participation. For example, they can explore whether certain motivations, such as social interaction or competition, are significant factors driving people to join sports teams. SPSS provides tools for conducting factor analysis, making it accessible to students.
Cluster Analysis
Cluster analysis is a technique used to group similar data points together based on predefined characteristics. In the realm of recreational sports team participation, students can apply cluster analysis to identify distinct segments of participants with similar profiles. For instance, they can group participants based on age, gender, preferred sport, and participation frequency to discover meaningful patterns. This can be particularly useful for sports organizations aiming to tailor their offerings to specific target groups.
Survival Analysis
Survival analysis is commonly used in medical research but can also be applied to sports participation data. This method allows students to examine the duration of participants' engagement in sports teams, shedding light on factors that may influence the longevity of their involvement. For example, students can investigate the survival rates of participants in various sports and identify factors that contribute to longer or shorter participation durations.
Advanced Regression Techniques
While basic regression analysis helps understand linear relationships between variables, advanced regression techniques offer more nuanced insights. Students can explore:
- Logistic Regression: This is useful when the outcome variable is binary, such as whether a participant continues or discontinues team participation. Logistic regression can help identify the factors that significantly affect the likelihood of participation continuation.
- Multilevel Regression: In cases where data is hierarchically structured (e.g., participants within teams within regions), multilevel regression can account for the nested nature of the data. Students can investigate how individual and team-level factors interact to influence participation outcomes.
Ethical Considerations in Sports Data Analysis
As students immerse themselves further into the domain of statistics concerning participation in recreational sports teams, it becomes imperative to underscore the significance of ethical considerations within the realm of data analysis. The responsible management and the preservation of privacy associated with handling sensitive participant data take center stage in this endeavor. In light of this, it is worthwhile to elucidate a set of fundamental ethical principles that should remain at the forefront of our deliberations. Here are some key ethical principles to keep in mind:
Informed Consent
Prior to gathering data from participants, it is imperative to guarantee that they have willingly granted informed consent. This involves the comprehensive elucidation of the study's objectives, the intended utilization of their data, as well as the protection of their rights as active participants. Upholding ethical standards in research is paramount, with a central focus on safeguarding the well-being and rights of each individual involved.
Data Anonymization
Protect the identities of participants by anonymizing their data. Remove or encrypt personally identifiable information to prevent potential harm or privacy breaches. SPSS offers features for anonymizing data, such as recoding or de-identifying variables.
Data Security
Maintain the security of collected data. Store it in secure locations, limit access to authorized personnel, and follow data protection regulations, such as GDPR or HIPAA, depending on the context. Data breaches can lead to severe consequences, both legally and ethically.
Transparent Reporting
When presenting findings, be transparent about data collection and analysis methods. Clearly state any limitations, biases, or conflicts of interest that may impact the results. Transparent reporting promotes trustworthiness in research.
Conclusion
In this extended exploration of recreational sports team participation statistics using SPSS, we've delved into advanced statistical techniques that students can leverage to gain deeper insights into this field. Factor analysis, cluster analysis, survival analysis, and advanced regression techniques provide valuable tools for uncovering hidden patterns and relationships within sports participation data.
Furthermore, we've emphasized the critical importance of ethical considerations in sports data analysis. Responsible handling of participant data not only ensures compliance with ethical standards but also contributes to the integrity and credibility of research in the field of recreational sports team participation.
As students continue their academic journey, mastering these advanced statistical techniques and adhering to ethical principles will equip them with the skills and knowledge needed to excel in assignments and make meaningful contributions to the understanding of recreational sports team participation. Whether pursuing careers in sports science, management, or policy development, these skills are invaluable for shaping the future of recreational sports experiences and promoting active, healthy lifestyles for all.