Claim Your Discount Today
Get 10% off on all Statistics homework at statisticshomeworkhelp.com! Whether it’s Probability, Regression Analysis, or Hypothesis Testing, our experts are ready to help you excel. Don’t miss out—grab this offer today! Our dedicated team ensures accurate solutions and timely delivery, boosting your grades and confidence. Hurry, this limited-time discount won’t last forever!
We Accept
- Understanding Hypothesis Testing
- Formulating the Hypothesis
- Calculating the Test Statistic and P-Value
- Making the Decision
- Constructing Confidence Intervals
- Constructing Confidence Intervals for Proportions
- Constructing Confidence Intervals for Means
- Practical Application: Using Minitab
- Tips for Success in Hypothesis Testing and Confidence Intervals
- Understanding the Assumptions
- Using Statistical Software Efficiently
- Practicing Regularly
- Conclusion
Hypothesis testing and confidence intervals are fundamental tools in the field of statistics, providing the means to make data-driven decisions. This guide is designed to help students understand these concepts and apply them effectively to solve various statistical problems, such as determining population proportions and estimating means. By following the structured approach outlined here, you will gain the skills needed to confidently handle similar assignments.
Understanding Hypothesis Testing
Hypothesis testing is a systematic method used to make decisions based on sample data. It involves making an initial assumption, called the null hypothesis, and then using statistical evidence to test whether this assumption can be rejected in favor of an alternative hypothesis.
Formulating the Hypothesis
The first step in hypothesis testing is to clearly define your null and alternative hypotheses. These hypotheses form the basis of your statistical test.
Null Hypothesis (H0)
The null hypothesis represents a statement of no effect or no difference. It is the default assumption that there is no relationship between variables or that a parameter equals a specific value.
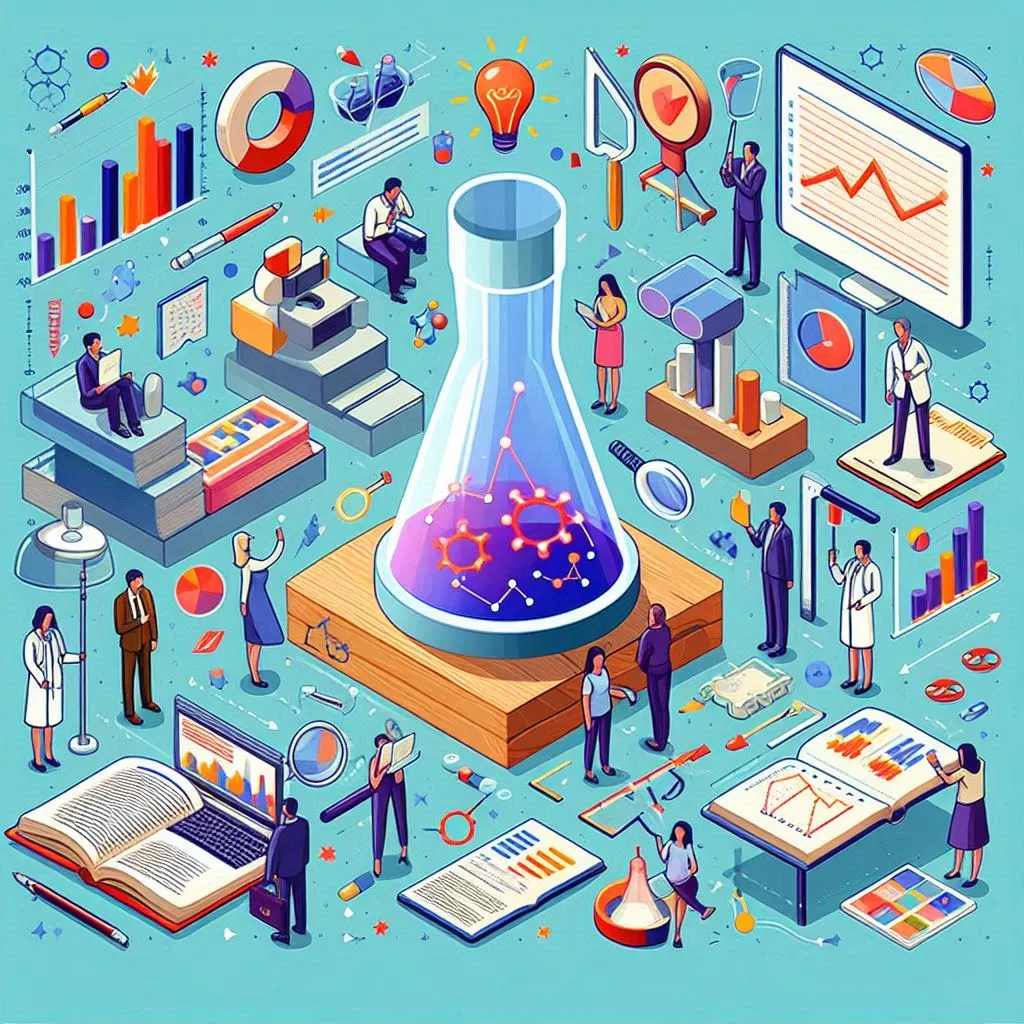
Example:
- H0: The population proportion of full-time World Campus students is 0.5.
- H0: The population mean air travel cost per college student is $1600 per year.
Alternative Hypothesis (H1)
The alternative hypothesis is what you aim to prove. It represents a statement of effect or difference and is considered when the null hypothesis is rejected.
Example:
- H1: The population proportion of full-time World Campus students is not 0.5.
- H1: The population mean air travel cost per college student is different from $1600 per year.
Calculating the Test Statistic and P-Value
Once the hypotheses are defined, the next step is to use statistical software, such as Minitab, to calculate the test statistic and p-value. These values are crucial for making decisions about the hypotheses.
Using Minitab for Calculations
1. Input Data:
- Enter your sample data into Minitab.
- For proportions, use the 1-Proportion Test.
- For means, use the 1-Sample t-Test.
2. Calculate Test Statistic:
- Minitab computes the test statistic based on your data and the chosen test.
3. Determine P-Value:
- Minitab provides the p-value, which indicates the probability of observing the sample data if the null hypothesis is true.
Making the Decision
The decision to reject or fail to reject the null hypothesis is based on the p-value and the significance level (α), often set at 0.05.
Decision Rule
- If p-value ≤ α, reject the null hypothesis (evidence suggests an effect or difference).
- If p-value > α, fail to reject the null hypothesis (insufficient evidence to suggest an effect or difference).
Drawing Conclusions
After making the statistical decision, translate it into a real-world conclusion.
Example:
- If you reject the null hypothesis, conclude that there is statistical evidence that the proportion of full-time World Campus students is different from 0.5.
- If you fail to reject the null hypothesis, conclude that there is insufficient evidence to suggest the mean air travel cost is different from $1600 per year.
Constructing Confidence Intervals
Confidence intervals provide a range of values within which the true population parameter is likely to fall. They offer a measure of precision for your estimates.
Constructing Confidence Intervals for Proportions
To construct a confidence interval for proportions, use the following formula:
[ \hat{p} \pm Z \sqrt{\frac{\hat{p}(1-\hat{p})}{n}} ]
where (\hat{p}) is the sample proportion, (Z) is the Z-value corresponding to the confidence level (e.g., 1.96 for 95%), and (n) is the sample size.
Assumptions for Normal Approximation
For the normal approximation to be valid, the following conditions must be met:
- (np \geq 10)
- (n(1-p) \geq 10)
Example Calculation
Given:
- o Sample size ((n)) = 100
- o Sample proportion ((\hat{p})) = 0.27
Calculation: [ 0.27 \pm 1.96 \sqrt{\frac{0.27(1-0.27)}{100}} ]
Result:
- The 95% confidence interval for the proportion of full-time students.
Constructing Confidence Intervals for Means
To construct a confidence interval for means, use the following formula:
[ \bar{x} \pm t \frac{s}{\sqrt{n}} ]
where (\bar{x}) is the sample mean, (t) is the t-value corresponding to the confidence level and degrees of freedom, (s) is the sample standard deviation, and (n) is the sample size.
Assumptions for the t-Distribution
For the t-distribution to be appropriate, the sample data should be approximately normally distributed, especially for small sample sizes.
Example Calculation
Given:
- Sample size ((n)) = 125
- Sample mean ((\bar{x})) = $1750
- Sample standard deviation ((s)) = $435
Calculation: [ 1750 \pm t \frac{435}{\sqrt{125}} ]
Result:
- The 95% confidence interval for the mean travel cost.
Practical Application: Using Minitab
Minitab is a powerful tool for statistical analysis, enabling you to perform hypothesis tests and construct confidence intervals efficiently.
Example Assignment:Proportion of Full-Time Students
1. Formulate Hypotheses:
- H0: (p = 0.5)
- H1: (p \neq 0.5)
2. Calculate Test Statistic and P-Value:
- Input data into Minitab and perform a 1-Proportion Test.
3. Decision Making:
- Compare the p-value to your significance level and make a decision.
4. Conclusion:
- Translate the decision into a real-world conclusion about the proportion of full-time students.
5. Construct Confidence Interval:
- Use Minitab to find the 95% confidence interval for the proportion.
Example Assignment:Mean Travel Cost
1. Formulate Hypotheses:
- H0: (\mu = 1600)
- H1: (\mu \neq 1600)
2. Calculate Test Statistic and P-Value:
- Input data into Minitab and perform a 1-Sample t-Test.
3. Decision Making:
- Compare the p-value to your significance level and make a decision.
4. Conclusion:
- Translate the decision into a real-world conclusion about the mean travel cost.
5. Construct Confidence Interval:
- Use Minitab to find the 95% confidence interval for the mean travel cost.
Tips for Success in Hypothesis Testing and Confidence Intervals
To master hypothesis testing and confidence intervals, it is important to understand the underlying assumptions, use statistical software effectively, and practice regularly.
Understanding the Assumptions
Each statistical test and confidence interval has specific assumptions that must be met for the results to be valid.
Proportion Tests
- Ensure that (np \geq 10) and (n(1-p) \geq 10).
- This ensures that the sample size is large enough for the normal approximation to be valid.
Mean Tests
- Check for normality in the sample data, especially for small sample sizes.
- For large samples, the Central Limit Theorem assures that the sample mean will be approximately normally distributed.
Using Statistical Software Efficiently
Statistical software like Minitab can significantly streamline the process of hypothesis testing and confidence interval construction.
Inputting Data
- Enter data accurately to ensure reliable results.
- Utilize the software’s data visualization tools to understand your data better.
Performing Tests
- Select the appropriate test based on your hypotheses and data type.
- Follow the software’s guided steps to perform tests and interpret results.
Practicing Regularly
Regular practice with different datasets will enhance your proficiency in hypothesis testing and confidence interval construction.
Working with Diverse Data
- Practice with data from various fields to understand different contexts and applications.
- Analyze both real-world datasets and simulated data to broaden your experience.
Reflecting on Results
- After completing each analysis, reflect on the results and the steps you took.
- Identify any challenges faced and seek to understand them better for future analyses.
Conclusion
Mastering hypothesis testing and confidence intervals is essential for making informed decisions based on data. By following the steps outlined in this guide and practicing regularly, you will develop the skills needed to tackle similar statistics assignments with confidence. Remember to understand the assumptions, use statistical software effectively, and continuously practice improving your proficiency. Happy studying!