Claim Your Discount Today
Get 10% off on all Statistics homework at statisticshomeworkhelp.com! Whether it’s Probability, Regression Analysis, or Hypothesis Testing, our experts are ready to help you excel. Don’t miss out—grab this offer today! Our dedicated team ensures accurate solutions and timely delivery, boosting your grades and confidence. Hurry, this limited-time discount won’t last forever!
We Accept
- Descriptive Statistics: Painting a Picture of Data
- Mean, Median, and Mode: Unraveling Central Tendencies
- Variability Measures: Embracing Diversity in Data
- Inferential Statistics: Drawing Meaningful Conclusions
- Hypothesis Testing: Making Informed Decisions
- Confidence Intervals: Navigating the Uncertainty
- Inferential Statistics: Drawing Meaningful Conclusions
- Hypothesis Testing: Making Informed Decisions
- Confidence Intervals: Navigating the Uncertainty
- Conclusion:
Statistics, often hailed as the backbone of data science, stands as a formidable tool, wielding the power to extract profound insights from raw data. Its significance reverberates through various disciplines, influencing decision-making processes and shaping our understanding of the world. However, for students venturing into the realm of statistics, the path can be fraught with challenges. The intricacies of statistics assignments can be overwhelming, demanding a nuanced understanding of foundational concepts and practical applications. This blog endeavors to be more than a mere guide; it aspires to be a comprehensive companion, a beacon of practical homework help illuminating the path for students, enabling them to confront real-world problems with confidence and proficiency. Embarking on a statistical journey requires a solid grasp of foundational concepts. It's not just about crunching numbers; it's about unraveling the intricacies of variability, making informed inferences, and drawing meaningful conclusions. This blog will act as a gateway into the realm of statistical literacy, a foundational pillar that supports the entire edifice of statistical understanding. In this section, we will delve deep into the core tenets of statistical literacy, emphasizing the importance of concepts such as probability, sampling methodologies, and basic statistical measures.
Probability, a fundamental concept in statistics, underpins the uncertainty inherent in many real-world phenomena. Understanding probability is akin to deciphering the language of uncertainty, allowing students to navigate the complexities of random events and uncertain outcomes. By comprehending the principles of probability, students gain a powerful tool for predicting the likelihood of future events, a skill applicable in diverse fields from finance to healthcare. Basic statistical measures, such as mean, median, and mode, serve as the building blocks of statistical analysis.
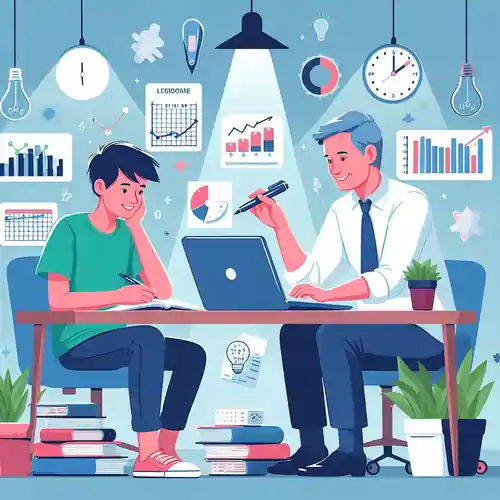
In this blog, we will explore these measures, elucidating their significance in summarizing and interpreting data, all of which will certainly help you complete your Statistics homework. Through practical examples and exercises, students will gain a hands-on understanding of how these measures unveil patterns and central tendencies within datasets.
Descriptive Statistics: Painting a Picture of Data
Descriptive statistics serves as the cornerstone for any meaningful statistical analysis, providing a systematic approach to understanding and interpreting data. In this section, we embark on a journey to unravel the significance of descriptive statistics, shedding light on how these tools enable students to make sense of complex datasets. The ultimate goal is to equip students with the necessary skills to summarize and present key features effectively.
Descriptive statistics, at its core, involves the utilization of various measures to describe and summarize data. Among these measures, the trio of mean, median, and mode stands out as fundamental tools in the statistician's toolkit. As we delve into the intricacies of these measures, students will gain a comprehensive understanding of central tendencies, laying the foundation for more advanced statistical analyses.
Mean, Median, and Mode: Unraveling Central Tendencies
Central tendencies, encapsulated by mean, median, and mode, represent the heart of descriptive statistics. These measures provide insights into the typical or central values within a dataset. In this sub-section, we unravel the nuances of mean, median, and mode, guiding students through the intricacies of each measure and elucidating when it is most appropriate to use them. The mean, often referred to as the average, represents the arithmetic average of a set of values. Median, on the other hand, is the middle value when data is arranged in ascending or descending order, and mode identifies the most frequently occurring value. Real-world examples will serve as beacons, illuminating the path for students to apply these measures effectively in diverse scenarios.
By understanding the central tendencies, students can discern the typical behavior of a dataset, identify outliers, and make informed decisions about the data's characteristics. Whether analyzing exam scores, income distributions, or product reviews, the mastery of mean, median, and mode empowers students to derive meaningful insights from the data at hand.
Variability Measures: Embracing Diversity in Data
Variability measures, including range and standard deviation, enrich the landscape of statistical analysis by providing a nuanced perspective on the spread of data points. As we navigate through this sub-section, students will gain a profound understanding of how to quantify variability and interpret its implications. Practical exercises will be interwoven into the learning journey, ensuring that students not only grasp the theoretical aspects but also cultivate the ability to apply these measures confidently in their assignments. The range, a straightforward measure, represents the difference between the maximum and minimum values in a dataset. Standard deviation, a more sophisticated measure, gauges the average deviation of each data point from the mean. Through engaging exercises, students will develop a hands-on understanding of these measures, allowing them to embrace the diversity inherent in datasets.
Understanding variability is crucial as it unveils the distribution and dispersion of data. Whether investigating the consistency of manufacturing processes or analyzing the volatility of financial markets, variability measures provide the statistical tools needed to make informed decisions. This sub-section serves as a bridge, connecting the theoretical concepts of variability to their real-world applications, ensuring that students emerge with a well-rounded skill set for tackling statistical challenges.
Inferential Statistics: Drawing Meaningful Conclusions
In the vast landscape of statistics, inferential statistics stands out as a powerful tool, enabling students to move beyond the confines of data samples and make insightful predictions about entire populations. This section serves as a guiding light for students delving into the intricacies of inferential statistics, emphasizing the importance of hypothesis testing, confidence intervals, and the nuanced understanding of p-values.
Hypothesis Testing: Making Informed Decisions
At the heart of inferential statistics lies the critical process of hypothesis testing. This sub-section serves as a comprehensive guide, breaking down the complex steps involved in hypothesis testing to demystify the subject for students. Hypothesis testing is not merely a theoretical concept; it is a practical tool for making informed decisions based on limited data. The journey through hypothesis testing begins with understanding the null hypothesis and formulating an alternative hypothesis. This sub-section will elaborate on the significance of these elements, providing students with a conceptual framework to approach real-world problems. Practical examples will be seamlessly integrated to illustrate how hypothesis testing becomes a bridge between statistical theory and its practical application.
Moreover, students will learn about the various types of errors associated with hypothesis testing – Type I and Type II errors. This nuanced understanding is crucial in real-world scenarios where the consequences of making errors can be significant. By exploring practical examples, students will grasp the implications of these errors and learn how to minimize them in their analyses.
Confidence Intervals: Navigating the Uncertainty
In the realm of inferential statistics, uncertainty is an ever-present companion. Confidence intervals emerge as a valuable tool for quantifying this uncertainty and providing a range within which population parameters are likely to reside. This sub-section aims to equip students with the skills to construct confidence intervals and interpret their significance. Students will delve into the mechanics of constructing confidence intervals, understanding the role of sample variability and the desired level of confidence. Real-life case studies will be explored to showcase instances where confidence intervals have played a pivotal role in decision-making. By engaging with these case studies, students will witness firsthand how confidence intervals enhance the reliability of statistical conclusions.
Furthermore, the sub-section will highlight the relationship between confidence intervals and hypothesis testing, emphasizing how these two components of inferential statistics complement each other. Students will gain insights into how confidence intervals provide additional depth to hypothesis testing results, offering a broader perspective on the uncertainty associated with statistical estimates.
Inferential Statistics: Drawing Meaningful Conclusions
In the vast landscape of statistics, inferential statistics emerges as a formidable and indispensable tool for students, extending beyond mere data analysis to empowering them with the ability to make predictions and draw far-reaching conclusions about entire populations. As students advance in their statistical journey, this section serves as an invaluable compass, navigating through the intricate terrain of hypothesis testing, confidence intervals, and the nuanced significance of p-values.
At its core, inferential statistics transcends the limitations of working with a specific dataset and ventures into the realm of broader insights. Hypothesis testing, a fundamental aspect of inferential statistics, involves a systematic process of formulating and assessing hypotheses about a population parameter based on sample data. This method allows students to move beyond describing the characteristics of a sample to making informed inferences about the population from which the sample was drawn.
Hypothesis Testing: Making Informed Decisions
At the heart of inferential statistics lies hypothesis testing, a pivotal process in drawing meaningful conclusions from a sample to a broader population. Imagine you have a small group of students, and you want to make a statement about the entire student body based on their opinions. Hypothesis testing provides a structured methodology for such scenarios. This sub-section will meticulously break down the steps involved in hypothesis testing, unraveling its complexity for students. From formulating null and alternative hypotheses to selecting significance levels, students will gain a comprehensive understanding of the process. The step-by-step guide is designed to demystify the intricacies, empowering students to apply hypothesis testing confidently in their assignments.
To bridge the gap between theory and application, practical examples will be seamlessly integrated. Students will explore real-world scenarios where hypothesis testing plays a crucial role. Whether it's determining the effectiveness of a new drug, assessing the impact of advertising on consumer behavior, or evaluating educational interventions, these examples will showcase the versatility of hypothesis testing in making informed decisions.
Confidence Intervals: Navigating the Uncertainty
Uncertainty is inherent in statistical analysis, and confidence intervals provide a robust framework for navigating this uncertainty. This sub-section will equip students with the skills to construct confidence intervals, allowing them to quantify the range within which population parameters are likely to fall. Constructing confidence intervals involves not only mathematical calculations but also a deep understanding of the underlying statistical concepts. Students will learn the nuances of selecting confidence levels and interpreting the results. The significance of confidence intervals in research and decision-making will be emphasized, emphasizing their role in providing a more nuanced perspective than point estimates.
Real-life case studies will be an integral part of this exploration, offering a glimpse into how confidence intervals are applied in diverse fields. From estimating the average income of a population to predicting the success rate of a marketing campaign, these case studies will demonstrate how confidence intervals enhance the reliability of statistical conclusions. By delving into these examples, students will develop a practical understanding of how to navigate uncertainty and make well-informed decisions in real-world situations.
Conclusion:
In the realm of academic pursuits, the mastery of statistics is akin to unlocking a treasure trove of knowledge and analytical prowess. As we draw the curtain on this comprehensive guide, it is imperative to underscore the pivotal role it plays in empowering students to navigate the intricate terrain of statistics homework with confidence and competence. The journey through statistics is akin to a voyage into the heart of data-driven decision-making. The foundations laid in this guide serve as the bedrock upon which students can construct a solid understanding of statistical principles. By comprehending the nuances of probability, sampling, and fundamental statistical measures, students not only gain theoretical insights but also cultivate a practical mindset essential for grappling with the diverse challenges posed by statistical assignments.
The exploration of descriptive statistics within this guide is analogous to providing students with a set of powerful lenses, enabling them to perceive the intricate details of datasets. The measures of central tendency—mean, median, and mode—become not just abstract concepts but tangible tools for students to summarize and interpret data effectively. As they grasp the significance of variability measures like range and standard deviation, students are equipped to embrace the diversity inherent in datasets, fostering a nuanced understanding that transcends mere numerical values. Moving beyond the descriptive, the guide extends its embrace to the realm of inferential statistics. Here, students are beckoned into the realm of hypothesis testing, confidence intervals, and the profound art of drawing meaningful conclusions from limited data. The step-by-step elucidation of hypothesis testing transforms it from a daunting procedure to a systematic and approachable method for making informed decisions. Confidence intervals, like a compass in the statistical wilderness, guide students through the uncertainty inherent in data, instilling in them the ability to navigate the complexities of statistical analysis with confidence.