- Understanding the Basics of SPSS
- Overview of SPSS
- Data Entry and Management in SPSS
- Key Features for Experimental Design in SPSS
- Descriptive Statistics and Graphs: Unlocking Data Insights
- Hypothesis Testing: Building a Robust Statistical Foundation
- Advanced Analyses in SPSS
- Regression Analysis
- Factor Analysis and Cluster Analysis
- Troubleshooting and Tips for Efficient SPSS Usage
- Common Challenges in SPSS
- Maximizing Efficiency in SPSS
- Conclusion
Embarking on the journey of experimental design, students find themselves navigating a complex landscape where the ability to harness statistical tools is paramount. The essence of their research lies not just in the data they collect but in the meaningful insights they can extract from it. It is in this intricate realm that the significance of mastering statistical tools becomes undeniably crucial. Among the myriad options available, one tool emerges as a beacon in the world of statistical analysis—the Statistical Package for the Social Sciences, more commonly known as SPSS. SPSS is a robust software package developed by IBM, specifically designed to cater to the intricate needs of statistical analysis. What sets SPSS apart is not just its computational prowess but its user-friendly interface, making it accessible to students with varying levels of statistical expertise. This guide aims to be a compass for students navigating the vast landscape of SPSS, providing not only a roadmap through its features but also fostering a deep understanding of its application in the realm of experimental design. If you need assistance with your SPSS homework, consider this guide as a valuable resource, offering insights into SPSS features and assisting you in mastering the challenges of your assignments.
At the core of this guide's mission is the empowerment of students. As they grapple with assignments requiring statistical analysis, the guide serves as a companion, unraveling the complexities of SPSS step by step. By the end of this comprehensive journey, students will not only have a nuanced understanding of the software but also the confidence to wield it effectively in their research endeavors.
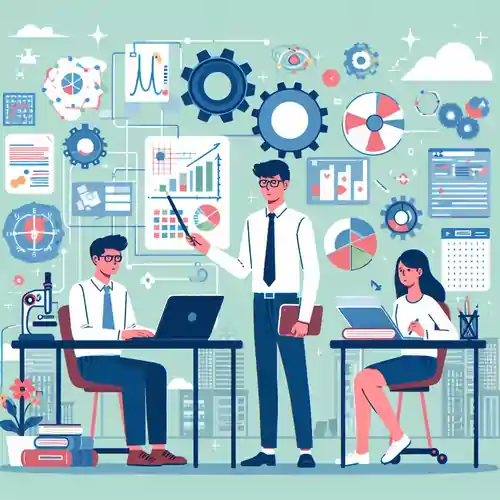
The beauty of SPSS lies in its versatility. It is not merely a tool for number crunching; rather, it's a sophisticated platform that supports a plethora of statistical procedures. From the rudimentary yet crucial task of data entry to the intricacies of advanced analyses, SPSS stands as an unwavering ally for students. The guide doesn't merely scratch the surface; it delves deep into the intricacies of SPSS, ensuring that students are equipped with the knowledge to harness its full potential.
Understanding the Basics of SPSS
Statistical Package for the Social Sciences (SPSS) is a robust software tool developed by IBM that has become synonymous with statistical analysis in various academic and research domains. Its widespread use can be attributed not only to its analytical power but also to its user-friendly interface, catering to individuals with diverse levels of statistical expertise. This section will delve deeper into the fundamental aspects of SPSS, covering its overall structure, versatility, and its pivotal role in simplifying statistical analyses for students.
Overview of SPSS
SPSS stands as a stalwart in the realm of statistical analysis, offering a comprehensive suite of tools for researchers and students alike. Developed by IBM, this software package has evolved to become a cornerstone in the analysis of social science data. What sets SPSS apart is its user-friendly interface, designed to accommodate users with varying levels of statistical proficiency. This accessibility makes it an ideal choice for students who may be navigating statistical waters for the first time.
Moreover, SPSS supports an extensive range of statistical procedures, making it an indispensable tool across diverse fields of study. Whether researchers are exploring trends in sociology, patterns in psychology, or conducting experiments in other scientific disciplines, SPSS provides a unified platform for conducting analyses efficiently. The software's adaptability to different research contexts makes it a go-to choice for anyone seeking to extract meaningful insights from their data.
Data Entry and Management in SPSS
Efficient data entry forms the bedrock of any successful statistical analysis, and SPSS excels in simplifying this critical phase of the research process. The software's intuitive interface guides students through the process of entering and managing their data with ease. Creating variables, defining data types, and organizing datasets become seamless tasks within the SPSS environment. One of the key advantages of SPSS in data management is its ability to handle large datasets without sacrificing performance. This becomes particularly beneficial for students dealing with extensive research data, ensuring that the software remains responsive and reliable even as the dataset grows in complexity.
Furthermore, SPSS facilitates the cleaning and preparation of data, saving students valuable time. The software offers features for identifying and handling missing data, outliers, and other data anomalies. This not only streamlines the data preparation phase but also contributes to the accuracy and integrity of the subsequent analyses.
Key Features for Experimental Design in SPSS
In the realm of experimental design, SPSS stands as an indispensable ally, offering a rich toolkit that empowers students to extract meaningful insights from their data. Two key features, Descriptive Statistics and Graphs, and Hypothesis Testing, play pivotal roles in laying the groundwork for robust statistical analyses.
Descriptive Statistics and Graphs: Unlocking Data Insights
Before students embark on the intricate journey of complex analyses, they must first grasp the fundamental characteristics of their data. SPSS facilitates this understanding by providing a diverse set of descriptive statistics, including measures like mean, median, and standard deviation. These measures offer insights into the central tendencies and distributions of variables, forming the bedrock of any analytical endeavor.
Furthermore, SPSS enhances the comprehension of data patterns through graphical representations such as histograms and box plots. These visual aids go beyond mere numerical values, offering an intuitive way to interpret data structures. Histograms vividly display the frequency distribution of a variable, while box plots provide a snapshot of the data's central tendency and spread. Together, descriptive statistics and graphs empower students to unravel the story hidden within their datasets, guiding them toward more informed research decisions.
Hypothesis Testing: Building a Robust Statistical Foundation
In the intricate dance of experimental design, hypothesis testing takes center stage as a critical performance. SPSS, with its user-friendly interface, serves as a seamless platform for conducting a myriad of tests crucial for validating hypotheses. The arsenal of tests includes staples like t-tests for comparing means, ANOVA for analyzing variances, and chi-square tests for examining associations between categorical variables. This section of the guide is a beacon for students navigating the hypothesis testing terrain. It not only elucidates the mechanics of formulating hypotheses but also guides students in selecting the most appropriate statistical tests for their specific research questions. The step-by-step walkthrough ensures that students not only run the tests efficiently but also decipher the results accurately.
Interpreting the results of hypothesis tests is often where students stumble. However, SPSS's output and visualization features simplify this process. The guide navigates students through result interpretation, demystifying p-values, confidence intervals, and effect sizes. Armed with this knowledge, students establish a robust statistical foundation for their experiments, ensuring the validity and reliability of their findings.
Advanced Analyses in SPSS
In the ever-evolving landscape of statistical analysis, mastering advanced tools is paramount for students seeking to derive nuanced insights from their data. SPSS, with its expansive repertoire, empowers researchers with the ability to conduct sophisticated analyses, enhancing the depth and scope of their experiments.
Regression Analysis
Regression analysis is a cornerstone in the toolkit of statisticians and researchers. For students navigating the intricate web of relationships between variables, SPSS provides an indispensable platform for conducting both simple and multiple regression analyses. Simple regression allows students to explore the linear relationship between two variables, elucidating how changes in one variable may predict changes in another. On the other hand, multiple regression extends this exploration to more than two variables, offering a nuanced understanding of the interplay among multiple factors.
This section of the guide is dedicated to demystifying the complexities of regression analysis within SPSS. Real-world examples will be dissected to illustrate how to interpret output tables, assess the statistical significance of coefficients, and evaluate the overall fit of the model. Step-by-step instructions will guide students through the entire process, from formulating research questions to drawing meaningful conclusions based on their analyses. By the end of this section, students will not only comprehend the theoretical underpinnings of regression but also gain practical skills to implement it confidently in their research projects.
Factor Analysis and Cluster Analysis
As research questions evolve, and experimental designs become increasingly intricate, the need for advanced techniques to discern underlying patterns within datasets becomes apparent. SPSS offers accessible interfaces for both factor analysis and cluster analysis, unleashing the potential to uncover latent structures and groupings within the data. Factor analysis is particularly beneficial when dealing with a multitude of variables, allowing researchers to identify latent constructs that might be driving the observed relationships. This section will guide students through the intricacies of factor analysis in SPSS, from choosing the appropriate extraction methods to interpreting factor loadings.
Real-world scenarios will be explored to exemplify how factor analysis can unveil hidden relationships, ultimately enriching the depth of students' research findings. Similarly, cluster analysis in SPSS enables students to categorize observations into meaningful groups based on similarities, revealing inherent structures within the data. The guide will walk students through the steps of cluster analysis, showcasing its application in diverse research scenarios. Whether identifying customer segments in market research or clustering biological specimens in scientific studies, SPSS facilitates a seamless exploration of data patterns.
Troubleshooting and Tips for Efficient SPSS Usage
Mastering SPSS is not without its challenges, even with its renowned user-friendly interface. Students often find themselves grappling with common issues that can impact the integrity of their analyses. This section is dedicated to addressing these challenges head-on, providing practical tips and solutions to empower students in overcoming obstacles and ensuring the reliability of their research.
Common Challenges in SPSS
Missing Data: One prevalent challenge is dealing with missing data, which can significantly skew results. SPSS offers various techniques to handle missing data, ranging from simple imputation methods to more complex statistical models. This section will guide students on how to identify and address missing data effectively, ensuring the completeness of their datasets.
Outliers: Outliers can exert undue influence on statistical analyses, potentially leading to erroneous conclusions. SPSS equips students with robust tools for identifying and handling outliers. This part of the guide will delve into outlier detection methods and outline steps to mitigate their impact, enabling students to conduct more accurate and reliable analyses.
Assumptions Violations: Many statistical tests in SPSS come with underlying assumptions that, if violated, can compromise the validity of the results. This section will elucidate common assumptions associated with tests like ANOVA or regression, and guide students on detecting and addressing violations. By ensuring that assumptions are met, students can have confidence in the robustness of their statistical inferences.
Maximizing Efficiency in SPSS
Time constraints are a constant companion for students navigating the demands of academic assignments. This part of the guide is tailored to help students optimize their workflow in SPSS, striking a balance between efficiency and precision.
Keyboard Shortcuts: Familiarizing oneself with SPSS keyboard shortcuts can significantly expedite tasks. This section will compile a list of essential shortcuts for common actions, allowing students to navigate the software more swiftly. A small investment in learning these shortcuts can yield substantial time savings in the long run.
Efficient Data Cleaning Techniques: Data cleaning is an integral part of the analysis process, but it doesn't have to be time-consuming. This guide will present efficient data cleaning techniques in SPSS, including automated processes for handling common issues like duplicates or inconsistencies. By adopting these techniques, students can ensure data quality without sacrificing precious time.
Conclusion
In the ever-evolving landscape of academia, where research is a cornerstone, the mastery of tools such as SPSS is a pivotal skill for students venturing into experimental design. As we draw the curtains on this comprehensive guide, it is essential to emphasize the profound significance of acquiring proficiency in SPSS. The journey through this tutorial has been a structured exploration, beginning with the rudimentary aspects of SPSS and culminating in the nuanced realm of advanced analyses.
The foundation laid in understanding SPSS's basic functionalities serves as a springboard for students to dive into the depths of statistical exploration. The user-friendly interface of SPSS facilitates a seamless entry into the world of statistical analysis, offering a nurturing environment for students with varying levels of expertise.