- What is Statistical Power?
- Using Excel for Power Analysis
- Step 1: Define the Parameters
- Step 2: Choose the Appropriate Statistical Test
- Step 3: Calculate the Sample Size
- Step 4: Interpret the Results
- Conclusion
Statistical power analysis is undeniably a pivotal tool within the realm of statistics. Its primary purpose is to furnish researchers and students with the means to quantify the likelihood of detecting an effect in a study, provided that it genuinely exists. This probability estimation is not merely an academic exercise but a linchpin in the intricate process of experimental design. The outcome of a power analysis can make or break a study by ensuring that it is sufficiently powered to yield results that carry substantive significance. This is where Microsoft Excel, a universally accessible and user-friendly software, emerges as a valuable ally for conducting power analyses. In the forthcoming sections of this blog post, we will embark on an in-depth exploration of the foundational concepts of statistical power analysis using MS Excel. This step-by-step guide has been meticulously crafted with the aim of providing students with the resources they need to not only comprehend the intricacies of power analysis but to excel in assignments that necessitate its application.
For those seeking assistance with your Statistical Power Analysis Using MS Excel, this blog post will serve as an invaluable resource. We will unravel the core principles of power analysis, elucidate the significance of effect size, delve into the nuances of the significance level, and underscore the criticality of achieving a desired power level. Armed with this knowledge, students can confidently navigate the complexities of statistical analysis, harnessing the power of Excel's functions to calculate sample sizes effectively. Moreover, the insights shared here will empower students to interpret and leverage the results of their power analyses, ensuring that their research assignments are not merely academically rigorous but also practically impactful. So, as you embark on your journey into the realm of statistical power analysis using MS Excel, remember that this blog post is your trusted guide, offering both knowledge and practical guidance to ensure your success.
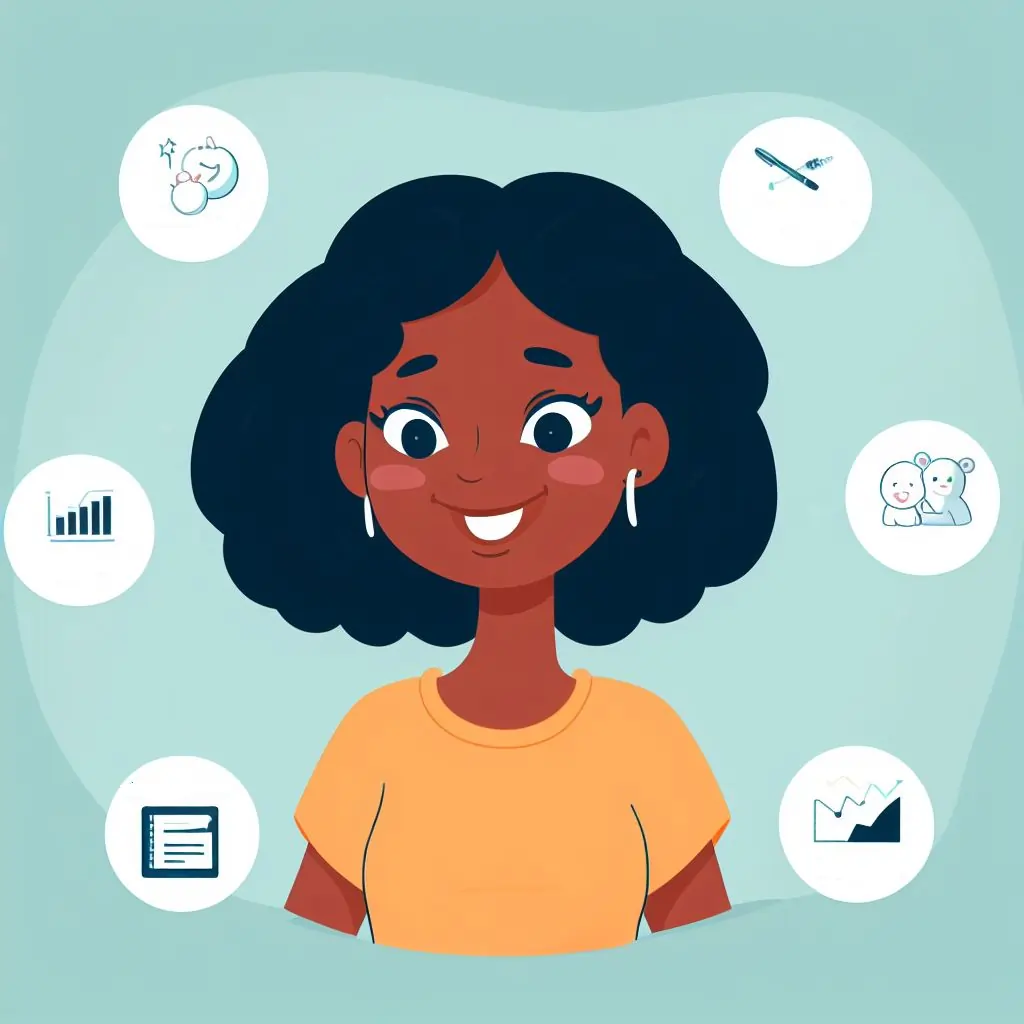
What is Statistical Power?
Before delving into the Excel-based analysis, it's imperative to establish a comprehensive understanding of what statistical power entails. Statistical power, often denoted as "power" (1 - β), represents a pivotal concept in the realm of statistics. It can be elucidated as the probability of correctly rejecting the null hypothesis when, in actuality, it is false. In simpler terms, it measures the efficacy of a statistical test in discerning an effect or difference if it genuinely exists within the population under investigation.
To grasp the significance of statistical powerfully, it is essential to recognize that it is contingent upon several interrelated factors. These influential factors encompass the sample size, effect size, significance level (α), and the specific statistical test being employed. It is essential to emphasize that these factors are not isolated but intricately connected. Adjusting any of these factors can potentially exert a substantial impact on the overall power of the statistical test being conducted.
In essence, statistical power is the bedrock upon which the reliability and robustness of statistical analyses rest. The ability to correctly identify and conclude the presence of an effect or difference is a fundamental objective of statistical research. A high degree of statistical power signifies an increased likelihood of achieving this objective, thereby enhancing the credibility and validity of research findings. Consequently, an adept comprehension of statistical power serves as an indispensable tool for researchers and students alike, enabling them to design studies that can yield meaningful and dependable outcomes.
Using Excel for Power Analysis
Now that we've defined statistical power let's explore how to conduct a power analysis using Microsoft Excel. We will break down the process into four main steps:
Step 1: Define the Parameters
Before opening Excel, it's essential to have a clear understanding of your research question and the parameters required for the power analysis. These parameters include:
1: Effect Size (ES)
Effect size represents the magnitude of the difference or the strength of the relationship you expect to find. It is essential to choose a realistic effect size based on prior research or your domain knowledge. A larger effect size generally requires a smaller sample size to achieve adequate power.
2: Significance Level (α)
The significance level (α) is the probability of committing a Type I error, which is the likelihood of incorrectly rejecting the null hypothesis when it is true. Commonly used values for α include 0.05 (5%) or 0.01 (1%). Choose an α level that aligns with your research standards.
3: Desired Power (1 - β)
The desired power (1 - β) represents the probability of correctly rejecting the null hypothesis when it is false. Researchers often aim for a power of 0.80 or higher, indicating an 80% chance of detecting an effect if it exists. You can adjust this value based on your research goals.
Step 2: Choose the Appropriate Statistical Test
The choice of statistical test depends on your research design and the data you plan to collect. Common tests include t-tests, ANOVA, regression analysis, and chi-squared tests, among others. Excel has built-in functions for many of these tests, making it a versatile tool for power analysis.
Step 3: Calculate the Sample Size
Once you have defined your parameters and selected the appropriate test, you can use Excel to calculate the required sample size for your study. Excel provides various functions and tools to perform these calculations:
1: t-Tests
If you are conducting a t-test, you can use the =POWER function in Excel. Here's how:
a: Calculate Sample Size for a One-Sample t-Test
Suppose you want to perform a one-sample t-test to compare a sample mean to a known population mean. Use the formula:
=POWER(1-α, (N-1), ES, 1)
Where:
α: Significance level
N: Sample size
ES: Effect size
b: Calculate Sample Size for a Two-Sample t-Test
For a two-sample t-test comparing means between two groups, use the formula:
=POWER(1-α, 2(N-1), 2ES, 1)
Where:
α: Significance level
N: Sample size per group
ES: Effect size
2: ANOVA
If you plan to conduct an analysis of variance (ANOVA), Excel can help determine the necessary sample size:
a: Calculate Sample Size for One-Way ANOVA
For a one-way ANOVA with k groups, use the formula:
=POWER(1-α, k*(N-1), (k-1)*ES, 1)
Where:
α: Significance level
N: Total sample size (across all groups)
ES: Effect size
b: Calculate Sample Size for Two-Way ANOVA
For a two-way ANOVA, use the following formula:
=POWER(1-α, (N-1), 2ES, 1)
Where:
α: Significance level
N: Total sample size
ES: Effect size
c: Other Tests
For tests like chi-squared tests or regression analysis, you may need to use different Excel functions or create custom calculations based on your specific research requirements.
Step 4: Interpret the Results
Having employed Excel to compute the requisite sample size, you will possess a crucial piece of information that will guide your research endeavors. Here's how to effectively interpret and apply this information:
Practical Application: Evaluate whether the computed sample size aligns with the constraints and resources of your research. If not, contemplate adjusting the effect size, significance level, or power level to strike a balance between research objectives and practicality.
Sample Size Justification: In research proposals and reports, elucidate the chosen sample size by referencing the power analysis. Justify why the selected sample size is congruent with your research aims.
Budget Allocation and Resource Planning: Profoundly comprehend the requisite sample size aids in planning data collection, budget allocation, and resource distribution. This ensures that your research is resource-efficient while yielding meaningful outcomes.
Conclusion
Statistical power analysis is an essential tool for researchers and students alike, ensuring that their studies have a high probability of detecting meaningful effects. Microsoft Excel can be a valuable ally in conducting power analyses, thanks to its user-friendly interface and built-in functions.
In this blog post, we've covered the basics of statistical power analysis, including the definition of power and the critical parameters involved. We've also provided step-by-step instructions on how to use Excel for power analysis, focusing on different types of statistical tests.By following these steps, students can enhance their understanding of statistical power and its practical application. Excel empowers students to make informed decisions regarding sample sizes in their research projects, ultimately improving the quality of their assignments and research endeavors.
As you delve deeper into the world of statistics, remember that statistical power analysis is not a one-size-fits-all solution. It requires careful consideration of your research goals, effect sizes, significance levels, and chosen tests. However, with practice and a solid grasp of Excel's capabilities, you can confidently tackle power analysis and contribute to more robust and insightful research.So, whether you're an aspiring researcher or a student working on assignments, harness the power of statistical analysis with Excel, and watch your studies thrive.