- Understanding Statistics Problems
- Key Concepts in Statistics
- Identifying Key Information
- Converting Data Points to Z-Scores
- Solving Common Types of Statistics Problems
- Percentage of Data Above or Below a Certain Point
- Using the Empirical Rule
- Finding Areas Under the Standard Normal Curve
- Advanced Statistics Problems
- Standard Errors and Sample Means
- Visualizing and Interpreting Data
- Reading Normal Distribution Curves
- Interpreting Histograms
- Box Plots
- Conclusion
Statistics can be an intimidating subject for many students, but with the right approach and tools, solving your statistics homework can become a manageable and even enjoyable task. This comprehensive guide will walk you through the steps to tackle typical statistics problems, providing clear explanations and examples. Whether you are working on problems related to normal distribution, z-scores, or probabilities, this guide has got you covered.
Understanding Statistics Problems
To solve any statistics problem effectively, you must first understand the question and identify the key information provided. This section will help you break down the problem and understand what is being asked.
Key Concepts in Statistics
Normal Distribution
The normal distribution, also known as the Gaussian distribution, is a continuous probability distribution that is symmetrical around the mean. This means most of the data points cluster around the mean, with fewer data points appearing as you move away from the mean.
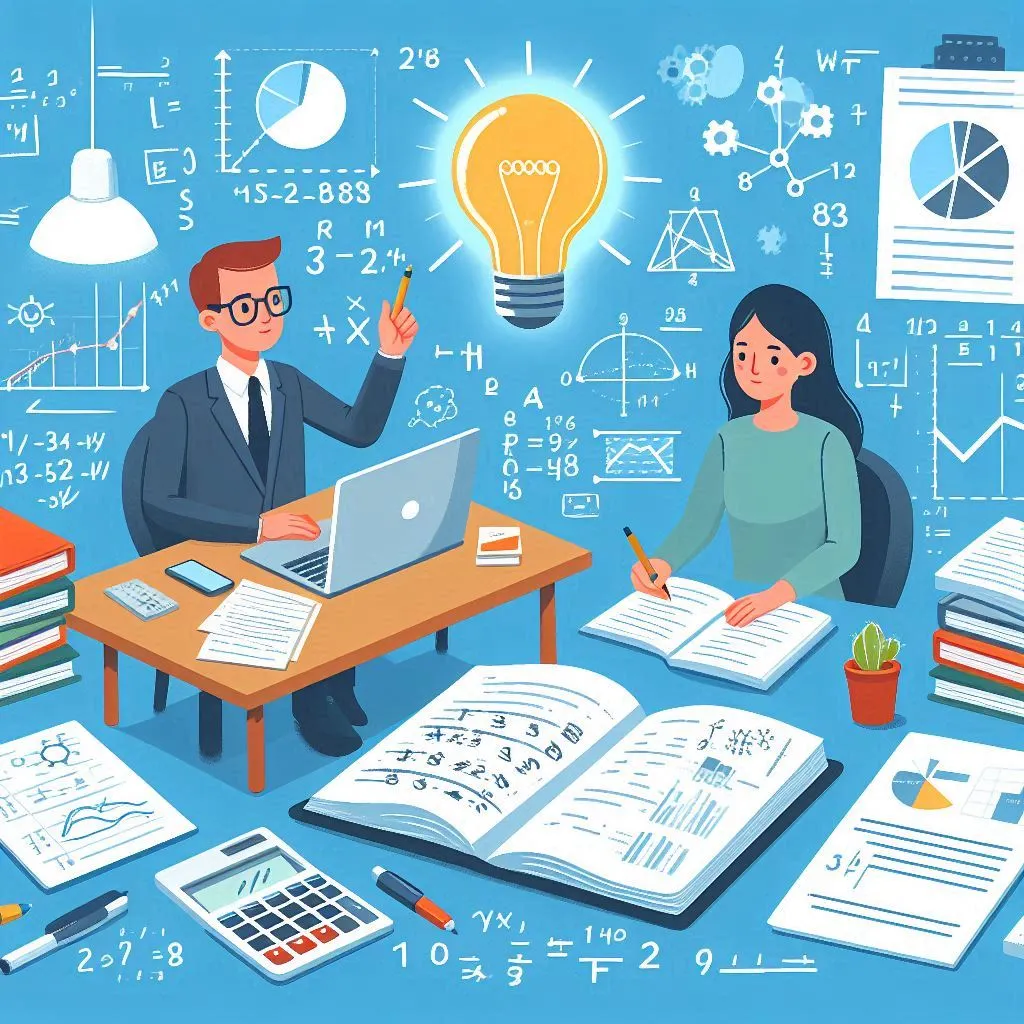
Mean and Standard Deviation
- Mean (μ): The average value of the dataset.
- Standard Deviation (σ): A measure of the amount of variation or dispersion in the dataset.
Z-Scores
A z-score represents the number of standard deviations a data point is from the mean. It is calculated using the formula: [ z = \frac{(X - \mu)}{\sigma} ] where (X) is the value you are converting, (\mu) is the mean, and (\sigma) is the standard deviation.
Identifying Key Information
When you read a statistics problem, identify the following:
- The mean (μ) and standard deviation (σ).
- The specific data points or intervals in the question.
- Whether the question asks for a percentage, probability, or a range of values.
Converting Data Points to Z-Scores
Converting raw data to z-scores is a crucial step in solving many statistics problems. It allows you to use standard normal distribution tables or calculators to find probabilities and percentages.
Solving Common Types of Statistics Problems
Now that you have a basic understanding of the key concepts, let's dive into solving some common types of statistics problems. This section will provide detailed explanations and examples to guide you through the process.
Percentage of Data Above or Below a Certain Point
Example Problem 1
Question: Assuming that the heights of college women are normally distributed with a mean of 62 inches and a standard deviation of 3.5 inches, what percentage of women are taller than 55 inches?
Solution:
- Calculate the Z-Score: [ z = \frac{(55 - 62)}{3.5} = -2 ]
- Find the Area: Use the standard normal distribution table to find the area to the left of (z = -2), which is approximately 0.0228.
- Calculate the Percentage: Subtract this value from 1 to find the area to the right: [ 1 - 0.0228 = 0.9772 ] Therefore, about 97.7% of women are taller than 55 inches.
Example Problem 2
Question: Assuming that the heights of college women are normally distributed with a mean of 65 inches and a standard deviation of 3.5 inches, what percentage of women are shorter than 68.5 inches?
Solution:
- Calculate the Z-Score: [ z = \frac{(68.5 - 65)}{3.5} = 1 ]
- Find the Area: Use the standard normal distribution table to find the area to the left of (z = 1), which is approximately 0.8413.
- Calculate the Percentage: Therefore, about 84.1% of women are shorter than 68.5 inches.
Example Problem 3
Question: Assuming that the heights of college women are normally distributed with a mean of 64 inches and a standard deviation of 1.5 inches, what percentage of women are between 65.5 inches and 68.5 inches?
Solution:
- Calculate the Z-Scores:
- For 65.5 inches: [ z_1 = \frac{(65.5 - 64)}{1.5} = 1 ]
- For 68.5 inches: [ z_2 = \frac{(68.5 - 64)}{1.5} = 2.33 ]
- Find the Areas:
- Area to the left of (z_1) = 0.8413
- Area to the left of (z_2) = 0.9901
- Calculate the Percentage: [ 0.9901 - 0.8413 = 0.1488 ] Therefore, about 14.9% of women are between 65.5 and 68.5 inches.
Using the Empirical Rule
The empirical rule, also known as the 68-95-99.7 rule, is a handy tool for quickly estimating probabilities and understanding the distribution of data within one, two, and three standard deviations of the mean.
Estimating Probabilities with the Empirical Rule
Example Problem 4
Question: At a certain excavation site, archaeological studies have used tree-ring dating to determine when people lived there. Wood from several excavations gave a mean of 1305 with a standard deviation of 22 years. Use the empirical rule to estimate a range of years centered about the mean in which about 68.3% of the data (tree-ring dates) will be found.
Solution:
- Identify the Mean and Standard Deviation: Mean (μ) = 1305, Standard Deviation (σ) = 22.
- Apply the Empirical Rule: About 68.3% of the data will fall within one standard deviation of the mean.
- Calculate the Range: [ 1305 - 22 = 1283 \quad \text{to} \quad 1305 + 22 = 1327 ] Therefore, about 68.3% of the data will be found between 1283 and 1327.
Converting X Intervals to Z Intervals
Example Problem 5
Question: Suppose a certain species of fawns between 1 and 5 months old have a body weight that is approximately normally distributed with mean (\mu) kilograms and standard deviation (\sigma) kilograms. Convert the following x interval to a z interval: ( x < 42.6 ).
Solution:
- Calculate the Z-Score: [ z = \frac{(42.6 - \mu)}{\sigma} ] Without the specific values for (\mu) and (\sigma), you would normally plug these in to find the z-score.
- Interpret the Z-Interval: Once you have the z-score, you can use standard normal distribution tables to interpret the interval.
Finding Areas Under the Standard Normal Curve
Understanding how to find areas under the standard normal curve is essential for interpreting probabilities and percentages related to z-scores.
Area to the Left of a Z-Score
Example Problem 6
Question: Find the area under the standard normal curve to the left of ( z = 1 ).
Solution:
- Use the Standard Normal Table: Find the area corresponding to ( z = 1 ), which is approximately 0.841.
- Interpret the Result: Therefore, about 84.1% of the data is to the left of ( z = 1 ).
Area to the Right of a Z-Score
Example Problem 7
Question: Find the area under the standard normal curve to the right of ( z = 3 ).
Solution:
- Use the Standard Normal Table: Find the area corresponding to ( z = 3 ), which is approximately 0.001.
- Interpret the Result: Therefore, about 0.1% of the data is to the right of ( z = 3 ).
Area Between Two Z-Scores
Example Problem 8
Question: Find the area under the standard normal curve between ( z = 1 ) and ( z = 2 ).
Solution:
- Use the Standard Normal Table:
- Area to the left of ( z = 1 ) = 0.841
- Area to the left of ( z = 2 ) = 0.977
- Calculate the Area: [ 0.977 - 0.841 = 0.136 ] Therefore, about 13.6% of the data is between ( z = 1 ) and ( z = 2 ).
Advanced Statistics Problems
In addition to basic probability and z-score problems, you may encounter more advanced problems involving standard errors and sample means. This section will guide you through these types of problems.
Standard Errors and Sample Means
When dealing with sample means, the standard error of the mean is a key concept. It measures how much the sample mean is expected to vary from the true population mean.
Calculating Standard Error
Example Problem 9
Question: Suppose that ( x ) has a distribution with ( \mu =
14 ) and ( \sigma = 8 ). If a random sample of size ( n = 53 ) is taken, find the standard error.
Solution:
- Calculate the Standard Error: [ \text{Standard Error} = \frac{\sigma}{\sqrt{n}} = \frac{8}{\sqrt{53}} \approx 1.10 ] Therefore, the standard error is approximately 1.10.
Probability of Sample Means
Example Problem 10
Question: The heights of 18-year-old men are approximately normally distributed with mean 68 inches and standard deviation 3 inches. What is the probability that the average height of a sample of ten 18-year-old men will be less than 69 inches?
Solution:
- Calculate the Standard Error: [ \text{Standard Error} = \frac{\sigma}{\sqrt{n}} = \frac{3}{\sqrt{10}} \approx 0.95 ]
- Calculate the Z-Score: [ z = \frac{(69 - 68)}{0.95} \approx 1.05 ]
- Find the Area: Use the standard normal distribution table to find the area to the left of ( z = 1.05 ), which is approximately 0.8531.
- Interpret the Result: Therefore, the probability is about 85.31%.
Visualizing and Interpreting Data
In addition to numerical calculations, visualizing data is crucial for understanding and interpreting statistical information. This section will cover how to interpret graphs and charts commonly used in statistics.
Reading Normal Distribution Curves
Normal distribution curves are bell-shaped and symmetrical around the mean. Key points to note:
- The highest point is at the mean.
- The curve approaches, but never touches, the horizontal axis.
Interpreting Histograms
Histograms are used to represent the frequency distribution of data. They show how data is distributed across different intervals. Look for:
- Symmetry or skewness.
- Peaks indicating the mode(s).
Box Plots
Box plots (or whisker plots) provide a visual summary of the data's distribution, highlighting the median, quartiles, and potential outliers. Key elements include:
- The box represents the interquartile range (IQR).
- The line inside the box represents the median.
- Whiskers extend to the smallest and largest values within 1.5 times the IQR from the quartiles.
Conclusion
Solving statistics homework assignments involves understanding key concepts such as normal distribution, z-scores, and probabilities. By breaking down the problems, converting data points to z-scores, and using tools like the standard normal distribution table and the empirical rule, you can tackle a wide range of statistics problems effectively. Additionally, visualizing data through graphs and charts can provide deeper insights into the data's distribution and trends. With regular practice and a systematic approach, you can master the skills needed to excel in statistics.