When tackling assignments that require statistical analysis, a solid understanding of how to implement correlation and regression models can greatly enhance your ability to interpret complex datasets and make well-informed decisions. These analytical techniques are crucial for extracting meaningful insights from data, enabling you to explore and understand the relationships between variables, forecast future trends, and evaluate hypotheses. Correlation analysis helps in identifying the strength and direction of relationships between pairs of variables, while regression analysis provides a detailed framework for modeling these relationships and predicting outcomes.
Correlation and regression models offer structured methods to quantify and visualize relationships, allowing you to assess how changes in one variable may impact another. By mastering these techniques, you can uncover underlying trends, detect patterns, and generate forecasts that are essential for strategic decision-making in business, research, and other domains. These models help you transform raw data into actionable insights, guiding decisions such as optimizing marketing strategies, evaluating the effectiveness of campaigns, or forecasting sales performance.
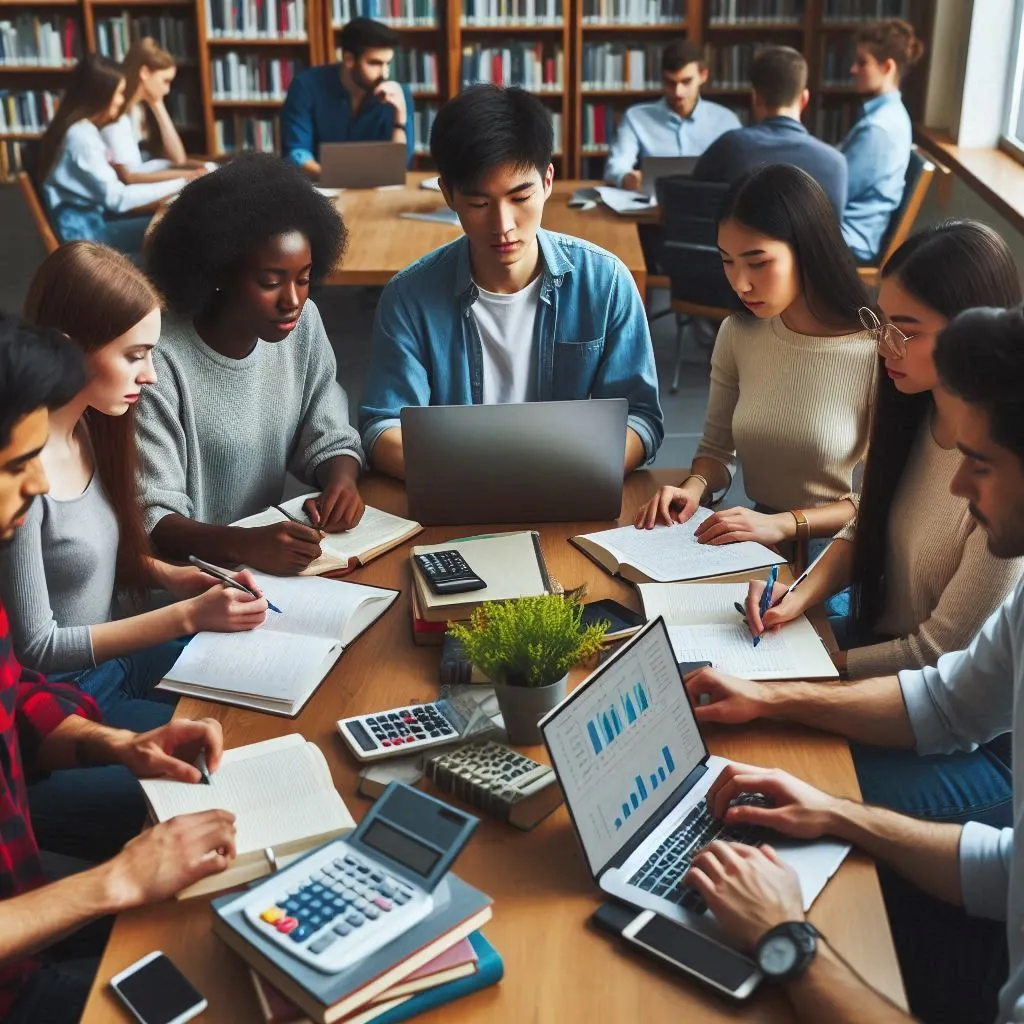
Furthermore, implementing these models in Excel provides a practical and accessible means of performing sophisticated statistical analyses without requiring advanced software. Excel's functionalities, such as the CORREL and LINEST functions, allow for efficient computation and interpretation of correlation coefficients and regression equations, respectively. This makes Excel an invaluable tool for students and professionals alike who need to apply statistical methods in various assignments and real-world scenarios.
Below is a comprehensive framework designed to guide you through assignments involving correlation and regression models in Excel. This framework ensures that you can apply these statistical methods effectively, regardless of the specific context of your assignment. It covers everything from data preparation and analysis to interpretation and presentation, providing a structured approach to achieving accurate and insightful results. By following this framework, you will enhance your analytical skills and be better equipped to tackle a wide range of statistical challenges, ultimately leading to more informed and effective decision-making. Whether you're new to these techniques or seeking to refine your approach, this guide is invaluable for anyone needing excel homework help to excel in their assignments.
Understanding Correlation and Regression Analysis
When diving into statistical analysis, correlation and regression analysis are fundamental techniques that offer critical insights into the relationships between variables. These methods are pivotal for identifying patterns, making predictions, and guiding informed decision-making based on empirical data. Understanding how to apply and interpret these techniques can significantly enhance your ability to analyze data and derive actionable conclusions. Whether you're a student or a professional, mastering these techniques is essential, especially if you seek statistics homework help to support your studies or work.
Correlation Analysis delves into the relationship between two variables, evaluating how they move in relation to each other. This is quantified using the correlation coefficient, a statistical measure that ranges from -1 to 1. A coefficient close to 1 indicates a strong positive relationship, suggesting that as one variable increases, the other variable tends to increase proportionally. For example, a high correlation coefficient between advertising spend and sales revenue would imply that increased spending is associated with higher sales. Conversely, a coefficient near -1 signifies a strong negative relationship, where an increase in one variable corresponds to a decrease in the other. For instance, a negative correlation between product price and quantity sold would suggest that as prices increase, sales tend to decrease. A coefficient around 0 implies a weak or negligible linear relationship between the variables, indicating that the variables do not move together in any discernible pattern. This measure is crucial for assessing the degree to which variables are associated, helping to reveal underlying trends and dependencies in your data.
Regression Analysis builds on the insights provided by correlation analysis by modeling the relationship between variables, thus enabling more detailed predictions and a deeper understanding of how variables interact. This technique helps establish how one or more independent variables (predictors) impact a dependent variable (outcome). The core components of regression analysis include:
- Slope Coefficient:This value represents the rate at which the dependent variable changes for each unit change in the independent variable. It reflects the strength of the relationship and indicates whether the change is positive or negative. For instance, a positive slope coefficient in a regression analysis of advertising spend and sales would suggest that as advertising expenditure increases, sales revenue also increases. Conversely, a negative slope would indicate that higher advertising spend leads to lower sales, although this would be unusual in practice.
- Y-Intercept: This value denotes the expected value of the dependent variable when the independent variable is zero. It provides the baseline from which changes in the dependent variable are measured and helps to contextualize the starting point of the regression model. For example, in a regression model predicting sales based on advertising spend, the y-intercept would represent the predicted sales when no money is spent on advertising.
In regression analysis, the relationship between variables is typically expressed through a mathematical equation of the form Y=mX+B. This equation allows for the prediction of the dependent variable Y based on the independent variable X, where m represents the slope coefficient and B represents the y-intercept. This predictive model is invaluable for forecasting future trends, assessing potential outcomes, and making data-driven decisions. For example, if you are analyzing customer purchase behavior, a regression model can help predict future sales based on current marketing efforts or economic conditions.
Both correlation and regression analyses are integral to understanding and interpreting complex datasets. They provide a structured framework for analyzing how variables interact, predicting future values, and supporting decision-making with empirical evidence. Mastery of these techniques not only helps in uncovering meaningful patterns and trends but also enables you to draw more accurate and actionable conclusions from your data. By effectively applying these methods, you can enhance your analytical capabilities, contribute valuable insights to your field, and make more informed decisions based on robust statistical evidence.
Step-by-Step Approach to Statistical Analysis in Excel
When undertaking assignments that involve statistical analysis, mastering tools such as correlation and regression models is essential for deriving meaningful insights and making informed decisions. These statistical techniques are invaluable for understanding relationships between variables, predicting future trends, and applying data-driven strategies. Excel, with its robust analytical functions, is an excellent platform for performing these analyses. Below is an expanded, detailed step-by-step approach to help you implement correlation and regression models in Excel, ensuring your analysis is thorough, accurate, and adaptable to various scenarios.
- Data Preparation:Begin by meticulously organizing your dataset in Excel to ensure that it is clean, accurate, and appropriately structured for analysis. This step involves not only entering your data into clearly labeled columns but also conducting preliminary checks to identify and rectify any errors or inconsistencies. Clean the dataset by removing or correcting any inaccuracies and ensure that data is formatted correctly. You may need to calculate descriptive statistics such as means, medians, ranges, and standard deviations to summarize the central tendency and variability of your data. Properly structuring and preparing your data is crucial for accurate and reliable statistical analysis, setting a solid foundation for subsequent steps.
- Correlation Analysis:Perform a detailed correlation analysis using Excel’s built-in functions to measure the strength and direction of the relationship between two variables. Apply the CORREL function to compute the correlation coefficient, which ranges from -1 to 1. A coefficient close to 1 indicates a strong positive correlation, meaning that as one variable increases, the other tends to increase as well. Conversely, a coefficient near -1 suggests a strong negative correlation, where an increase in one variable corresponds to a decrease in the other. A coefficient around 0 implies a weak or negligible relationship. Interpreting these coefficients involves assessing how strongly variables are related and supporting your findings with scholarly sources that explain the relevance of correlation coefficients in various business contexts, such as customer behavior analysis or financial forecasting.
- Performing Regression Analysis: Conduct a thorough regression analysis using Excel’s advanced regression tools, such as the Analysis Toolpak or the LINEST function. This step involves creating a regression model to quantify the relationship between independent and dependent variables. Analyze the regression output to determine key components, including the slope coefficient (m), which indicates the rate of change in the dependent variable for each unit change in the independent variable, and the y-intercept (B), which represents the value of the dependent variable when the independent variable is zero. Express the regression equation in the format Y=mX+B, allowing you to make precise predictions about the dependent variable based on variations in the independent variable. This detailed regression analysis provides a robust framework for understanding and forecasting data trends.
- Interpret and Evaluate: In this step, explain how the regression equation derived from your analysis can be used to forecast future values by applying it to new or hypothetical scenarios. Evaluate the strength and reliability of your regression model using metrics such as R-squared, which measures the proportion of variability in the dependent variable explained by the independent variable. A higher R-squared value indicates a better fit of the model to the data. Enhance your analysis by inserting a trendline into your Excel chart to visualize the regression line and assess how well the data points align with it. This visual representation helps in understanding the predictive power and accuracy of your model, providing a clearer picture of its effectiveness.
- Application in Business: Discuss the practical applications of correlation and regression models in business scenarios, emphasizing their importance in making data-driven decisions. Explain how these statistical tools can be used to forecast sales trends, evaluate the effectiveness of marketing campaigns, or optimize operational strategies. Highlight how correlation can reveal significant relationships between key business variables, while regression analysis provides actionable insights for future planning and strategic decision-making. Illustrate the relevance of these models with real-world examples, such as predicting future sales based on past performance or assessing the impact of marketing efforts on customer engagement.
- Essay Composition:Assemble your findings into a well-structured and coherent essay. Begin with an introductory paragraph that sets the context for your analysis, outlining the objectives and significance of the study. The body of the essay should provide a detailed account of your analytical process, including the steps taken, the key findings, and your interpretations of the results. Ensure that you incorporate relevant data and insights to support your analysis. Conclude with a summary that highlights the main insights and implications of your findings. Make sure your essay adheres to APA formatting guidelines, includes citations from scholarly sources, and is free from errors. This structured approach will ensure that your analysis is presented clearly and professionally.
- Submission:Prepare for submission by organizing your Excel worksheet, ensuring it includes all relevant calculations, outputs, and charts related to your analysis. Alongside the worksheet, submit a well-crafted one-page essay that synthesizes your findings and adheres to assignment guidelines. Review your work thoroughly to ensure it is well-organized, meets all required criteria, and is formatted correctly. Properly preparing and submitting your work ensures that your analysis is presented effectively and professionally, meeting the expectations of your assignment or project.
By following these detailed steps, you can successfully tackle assignments involving correlation and regression analysis, providing a comprehensive and insightful analysis of your data. This approach ensures that you utilize Excel’s capabilities effectively, leading to accurate and actionable conclusions.
Conclusion
In summary, effectively utilizing correlation and regression analysis in Excel provides a powerful toolkit for interpreting complex datasets and making data-driven decisions. By meticulously preparing your data, conducting thorough correlation and regression analyses, and critically evaluating your findings, you gain valuable insights into the relationships between variables and their potential impact on future outcomes. Correlation analysis helps identify the strength and direction of relationships between variables, while regression analysis allows you to model these relationships and make precise predictions.
Applying these analytical methods to real-world scenarios, such as forecasting business performance or evaluating the effectiveness of marketing strategies, highlights their practical significance in business decision-making. Understanding how to interpret and evaluate the results of these analyses ensures that you can leverage statistical tools to inform strategic choices and optimize operations.
Ultimately, mastering these analytical techniques not only enhances your ability to tackle similar assignments with confidence but also equips you with essential skills for navigating data-driven environments. By synthesizing your findings into a well-structured essay, you demonstrate not only your analytical capabilities but also your ability to communicate complex insights clearly and effectively. This comprehensive approach ensures that your work is both insightful and professionally presented, reflecting your proficiency in statistical analysis and its applications in various contexts.