- Overview of Survival Analysis
- Kaplan-Meier Estimator
- Cox Proportional Hazards Model
- Data Preparation for Survival Analysis in SPSS
- Handling Missing Data
- Data Quality Assurance
- Interpreting Results and Reporting
- Interpretation of Kaplan-Meier Output
- Reporting Cox Regression Results
- Conclusion
Survival analysis stands as a robust statistical method with versatile applications across diverse fields, including but not limited to medicine, finance, and social sciences. It serves as a critical analytical tool for researchers, enabling them to examine and interpret time-to-event data, where the primary focus lies in understanding the duration until a particular event of interest occurs. This methodological approach provides a dynamic framework for analyzing outcomes that evolve, offering insights into the factors influencing the timing of events and their impact on different cohorts. In the context of this blog, we embark on a comprehensive exploration of the fundamental concepts underpinning survival analysis, specifically employing the Statistical Package for the Social Sciences (SPSS). SPSS is a widely adopted software tool that facilitates the application of sophisticated statistical techniques, making it an invaluable resource for students and researchers in the field of statistics. By utilizing SPSS for survival analysis, individuals gain access to a user-friendly interface that streamlines complex calculations and statistical modeling, enabling a more efficient and intuitive exploration of time-dependent data. Whether you are seeking help with your SPSS homework or aiming to enhance your proficiency in survival analysis using SPSS, this guide is crafted to be a valuable resource for students navigating the complexities of statistical analysis.
The significance of comprehending these key concepts cannot be overstated, especially for statistics students who are actively engaged in academic pursuits and research projects. Mastery of survival analysis equips students with the necessary skills to navigate assignments effectively and contribute meaningfully to scientific inquiries. As assignments increasingly involve real-world datasets and practical applications, a solid understanding of survival analysis becomes an indispensable asset for statistics students seeking to unravel intricate patterns within temporal data.
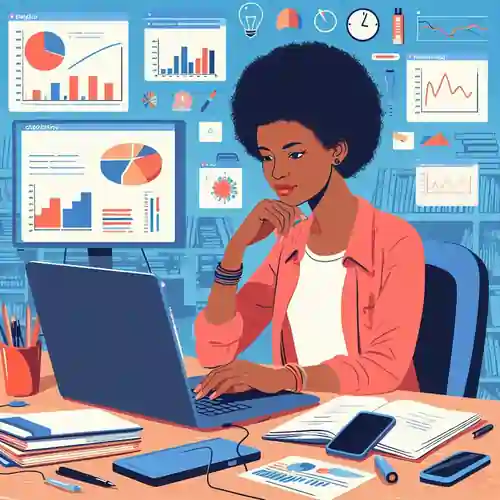
Survival analysis in SPSS introduces students to essential statistical techniques, such as the Kaplan-Meier estimator and the Cox proportional hazards model. The Kaplan-Meier estimator proves invaluable when dealing with censored data, a common scenario where not all subjects in a study have experienced the event of interest by the conclusion of the investigation. SPSS simplifies the implementation of the Kaplan-Meier estimator, allowing students to effortlessly generate survival curves that vividly illustrate the probability of events occurring over time. This visual representation aids in comparing survival experiences across different groups, a crucial aspect in many statistical analyses.
Overview of Survival Analysis
Survival analysis is a statistical method that plays a pivotal role in analyzing time-to-event data, focusing on the duration until a specific event of interest occurs. This versatile technique finds applications across diverse fields such as medicine, engineering, and social sciences, where understanding the time until an event happens is crucial. Whether it's gauging the time until a patient recovers from a medical condition, the duration until a machine malfunctions, or the period until a person secures a job post-graduation, survival analysis provides a robust framework for modeling and interpreting such temporal data.
Kaplan-Meier Estimator
The Kaplan-Meier estimator is a non-parametric method designed to estimate the survival function. This becomes especially crucial when dealing with censored data, a common scenario where not all individuals in a study have encountered the event by the study's conclusion. In the context of SPSS, employing the Kaplan-Meier estimator involves a straightforward process. Researchers commence by entering their data into the software, specifying the time variable representing the duration until the event and the event/censor variable indicating whether the event has occurred or is censored. Once these parameters are defined, SPSS facilitates the generation of a Kaplan-Meier survival curve, a graphical representation that vividly illustrates survival probabilities over time.
The Kaplan-Meier survival curve becomes an invaluable tool for statistics students as it allows for the visual comparison of survival probabilities among different groups. This capability enables students to discern patterns and trends in the data, making it an essential technique for those investigating the impact of diverse factors on survival outcomes. The simplicity and interpretability of the Kaplan-Meier estimator make it a cornerstone for introductory survival analysis.
Cox Proportional Hazards Model
On the other hand, the Cox proportional hazards model represents a more advanced and flexible approach in survival analysis. It operates as a regression model, enabling researchers to assess the influence of multiple covariates on the hazard of experiencing the event of interest. In SPSS, the Cox model is accessible through a user-friendly interface, making it an accessible yet powerful tool for statistics students engaged in more sophisticated analyses.
To implement the Cox proportional hazards model in SPSS, students input the time variable, event/censor variable, and covariates of interest. The model output includes hazard ratios and confidence intervals, providing a quantitative understanding of the factors influencing survival. This method is indispensable for students exploring nuanced relationships within their datasets, allowing them to uncover the intricate interplay between predictor variables and survival outcomes.
Data Preparation for Survival Analysis in SPSS
Data preparation is a fundamental aspect of any statistical analysis, and survival analysis in SPSS is no exception. Before delving into the intricacies of survival analysis, researchers and statistics students must acknowledge the critical role played by meticulous data preparation. This preparatory phase is multifaceted, encompassing several key components that are instrumental in ensuring the accuracy and reliability of the subsequent survival analysis. One of the primary challenges researchers encounter is missing data, a common occurrence that can significantly impact the validity of statistical analyses. In the realm of survival analysis, where the focus is on the time until an event occurs, missing data can be particularly problematic.
Handling Missing Data
One of the primary challenges encountered in survival analysis is the pervasive issue of missing data. Incomplete information can significantly skew results, leading to erroneous conclusions. SPSS, being a versatile statistical tool, equips users with a range of techniques to effectively handle missing data, with imputation methods standing out as a key player in this arena. Imputation involves estimating missing values based on observed data, and SPSS provides several imputation methods such as mean imputation, regression imputation, and multiple imputation. Statistics students must familiarize themselves with these options, understanding their strengths and limitations, to safeguard the reliability of their analyses.
In the context of survival analysis, where the timing of events is critical, imputing missing values for variables like time-to-event and censor indicators takes on heightened importance. Failure to address missing data in these variables could lead to biased survival estimates, potentially distorting the entire analysis. Therefore, students must exercise diligence in selecting and applying appropriate imputation methods to ensure the accuracy and integrity of their survival models.
Data Quality Assurance
The quality of the dataset serves as the bedrock upon which survival analyses are built. In the realm of SPSS, students are empowered with tools designed for data screening and cleaning, offering a systematic approach to identify and rectify outliers and anomalies that may lurk within the data. Data quality assurance involves a series of steps, including the identification and handling of outliers, addressing inconsistencies, and validating the overall integrity of the dataset. SPSS simplifies these processes, providing a user-friendly interface for tasks like identifying extreme values or assessing the distribution of variables.
By engaging in thorough data quality assurance, students enhance the robustness of their survival analyses. Outliers, if left unaddressed, can unduly influence results and compromise the validity of conclusions drawn from the analysis. Through the careful execution of data cleaning procedures, students ensure that their datasets are free from distortions, facilitating more accurate survival estimates and, consequently, more reliable insights into time-to-event phenomena.
Interpreting Results and Reporting
Interpreting the results of survival analysis in SPSS is a pivotal phase for statistics students, as it bridges the gap between raw data and actionable insights. Once the statistical computations are complete, students are confronted with a plethora of output tables that encapsulate the essence of their analysis. The journey from these tables to a comprehensive understanding of the findings is a nuanced process, requiring a keen eye for detail and a sound grasp of statistical concepts.
In the realm of survival analysis, the interpretation process often commences with the examination of Kaplan-Meier survival curves. These curves, generated by SPSS, vividly illustrate the estimated survival probabilities over time for different groups within the dataset. Students must scrutinize these curves, noting any divergences or convergence between groups.
Interpretation of Kaplan-Meier Output
When students embark on generating a Kaplan-Meier survival curve in SPSS, they are greeted with a trove of information encapsulated in output tables. The focal points of interpretation revolve around key metrics that unveil the dynamics of survival over time. One of these metrics is the median survival time, a central measure indicating the time at which 50% of the subjects have experienced the event of interest. This statistic serves as a robust summary of the survival distribution, offering a tangible reference point for understanding the central tendency of survival times. Another crucial element embedded in the Kaplan-Meier output is the log-rank test results. This statistical test compares the survival curves of different groups, assessing whether observed differences are statistically significant. A significant log-rank test implies that the survival experiences of the groups under scrutiny differ in a meaningful way.
Understanding and correctly interpreting the log-rank test results empower students to discern whether there are substantial disparities in survival between groups. Additionally, confidence intervals play a pivotal role in the interpretation process. These intervals provide a range within which the true population parameter, such as survival probability, is likely to fall. A narrower confidence interval signifies greater precision in the estimation, whereas a wider interval suggests increased uncertainty. Grasping the nuances of confidence intervals equips students with the ability to communicate the precision and reliability of their survival estimates effectively.
Reporting Cox Regression Results
Moving beyond the Kaplan-Meier analysis, statistics students often find themselves navigating the intricacies of Cox regression output in SPSS. This advanced statistical method unveils the impact of predictor variables on the hazard of experiencing the event of interest. The interpretation of Cox regression results is multifaceted, demanding a nuanced understanding of hazard ratios, p-values, and confidence intervals. Hazard ratios, a cornerstone of Cox regression output, quantify the relative risk of experiencing the event for one group compared to another. A hazard ratio greater than 1 indicates an increased risk, while a value less than 1 suggests a decreased risk. Students must discern the direction and magnitude of hazard ratios to unravel the comparative impact of predictor variables on survival.
Parallel to hazard ratios, p-values emerge as critical indicators of statistical significance. These values reflect the probability of observing the observed results under the assumption of no effect. A low p-value (typically less than 0.05) signifies statistical significance, prompting students to infer that the associated predictor variable exerts a significant influence on the hazard of the event. Conversely, a high p-value implies a lack of evidence to reject the null hypothesis, indicating non-significance. Complementing hazard ratios and p-values, confidence intervals for each covariate provide a range of plausible values for the true effect size. Understanding these intervals is paramount, as they offer insights into the precision of the estimated effects. Narrow intervals suggest more precise estimates, bolstering the confidence in the reported findings, while wider intervals introduce a degree of uncertainty.
Conclusion
Survival analysis, when conducted using the Statistical Package for the Social Sciences (SPSS), emerges as a crucial skill for statistics students. The significance of this statistical method extends beyond the realm of academia, finding applications in diverse fields where understanding time-to-event data is paramount. This blog has endeavored to unravel the intricacies of survival analysis in SPSS, emphasizing its role in offering profound insights that can directly influence decision-making processes across various domains.
One of the primary takeaways from this exploration is the recognition of survival analysis as a versatile tool. By delving into time-to-event data, researchers can uncover patterns, trends, and critical information that may remain obscured through conventional statistical methods. The Kaplan-Meier estimator, a non-parametric technique employed in SPSS, serves as a powerful ally in this pursuit. Through this method, students gain the ability to estimate survival probabilities over time, especially in scenarios where not all subjects have experienced the event of interest by the conclusion of the study. Armed with this knowledge, statistics students can adeptly compare survival curves across different groups, a skill that proves invaluable in discerning the impact of various factors on survival outcomes.