- Understanding the Assignment
- Missing Data Removal and Techniques for Imputation:
- Advanced Imputation Techniques and Analysis of Sensitivity:
- Prioritization, planning and Time Administration:
- Data Collection That Works:
- Cleaning and Preprocessing of Data-Analyzing Exploratory Data:
- Choosing the Best Analytical Techniques:
- Effective Data Analysis and Clear and Concise Reporting:
- Conclusion:
Data analysis assignments can be extremely difficult, especially when time is of the essence. The pressure to complete a task efficiently and effectively in a short period of time can be overwhelming. You can, however, conquer these assignments and achieve outstanding results by taking a strategic approach, implementing effective time management techniques, and engaging in meticulous planning. In this blog post, we will look at various strategies and techniques for dealing with data analysis homework when you have a tight deadline.
Understanding the Assignment
It is critical to thoroughly understand the assignment requirements in order to effectively handle data analysis homework with a tight deadline. Read and analyze the instructions provided by your professor or client carefully. Pay special attention to the key objectives, the type of data that must be analyzed, the specific analysis techniques to be used, and any other guidelines or constraints that have been specified. Gaining a clear understanding of the task at hand will allow you to plan and allocate your time more efficiently, increasing your chances of successfully completing the assignment. If any aspects of the assignment are unclear, do not be afraid to ask your professor or supervisor for clarification to avoid any misunderstandings or mistakes later on. Understanding the nature of missing data can help guide appropriate data handling techniques.
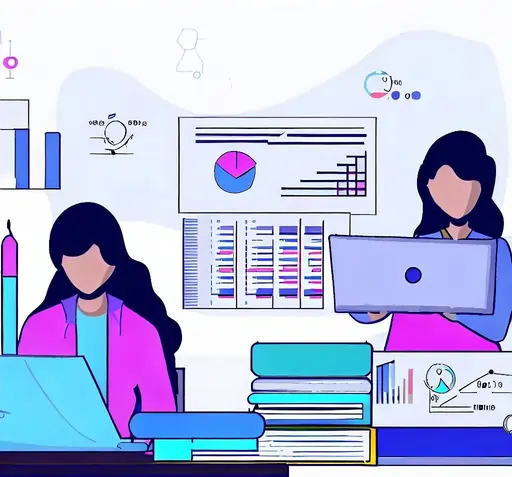
Missing Data Removal and Techniques for Imputation:
One simple solution is to delete observations with missing data. This technique is appropriate when missing data is minimal and distributed randomly across the dataset (MCAR). However, deleting missing data can result in a smaller sample size and potential bias if the messiness is not random. Use this approach with caution and consider its impact on the overall analysis.
Imputation is the process of replacing missing values with estimated values. Mean imputation, regression imputation, and multiple imputation are some of the imputation techniques available. Mean imputation substitutes the mean of the available data for that variable for missing values. Missing values are predicted using regression imputation based on their relationship with other variables. Using statistical models, multiple imputation generates multiple plausible imputed datasets. Each imputed dataset is analyzed independently before the results are combined. Based on the nature of the missing data and the analysis assumptions, select the appropriate imputation technique.
Advanced Imputation Techniques and Analysis of Sensitivity:
Advanced imputation methods like k-nearest neighbors (KNN), expectation-maximization algorithm (EM), and hot-deck imputation can also be used. KNN imputation estimates missing values based on the values of the dataset's nearest neighbors. Based on the observed data and model assumptions, the EM algorithm iteratively estimates missing values. Missing values are assigned using hot-deck imputation, which matches similar units in the dataset. These advanced methods may result in more accurate imputations, but they necessitate a greater understanding of the underlying algorithms and assumptions.
When dealing with missing data, sensitivity analysis is critical. Sensitivity analysis investigates the impact of various missing data handling techniques on the analysis's results. It enables you to evaluate the robustness of your conclusions under various imputation scenarios. You can assess the consistency and reliability of your findings by comparing the results obtained from different imputation methods.
Prioritization, planning and Time Administration:
Creating a comprehensive plan and effectively prioritizing your tasks is a critical step in managing data analysis homework with a short deadline. Divide the task into smaller, more manageable parts or milestones. Begin by identifying the necessary data cleaning steps, then proceed to the exploratory data analysis stage, and finally to the more complex analysis techniques. By breaking down your workload into smaller tasks, you can tackle them one at a time, making the overall process less daunting. Prioritizing tasks based on their importance and interdependence is critical for a smooth workflow. Allow enough time for each task, and keep in mind the complexity and estimated time required for completion. It is critical to set realistic deadlines and avoid procrastination in order to make steady progress toward completing the assignment within the timeframe specified. Flexibility is also essential because unexpected challenges may arise, necessitating changes to your plan along the way.
When faced with a short deadline for data analysis homework, effective time management is critical. Make a well-organized schedule outlining specific time slots for each task. Allow enough time for critical stages such as data collection, cleaning, analysis, and reporting. Consider the complexity and anticipated time required for each task, and set deadlines that are realistic. Avoid the temptation to procrastinate and stick to your schedule in order to make consistent progress toward completing the assignment within the timeframe specified. To ensure effective time management, it is critical to maintain focus, avoid distractions, and prioritize your work.
Data Collection That Works:
The data collection process is the foundation of any data analysis project. When working against a tight deadline, it is critical to collect relevant and accurate data as quickly as possible. Find credible sources that are relevant to the goals of your assignment. Use a variety of resources, including online databases, academic journals, surveys, and any other relevant sources of information. It is critical to ensure the data's quality and validity by cross-referencing information, verifying sources, and removing any outliers or inconsistencies. Take extra precautions at this stage, as the dependability and accuracy of the data collected will have a significant impact on the validity and credibility of your analysis.
Cleaning and Preprocessing of Data-Analyzing Exploratory Data:
Cleaning and preprocessing your data are critical steps in ensuring the accuracy and reliability of your analysis. You will need to deal with missing data, outliers, standardize variables, and address any inconsistencies in the dataset during this stage. Any oversights or mistakes during this process can lead to incorrect conclusions and interpretations, so paying attention to detail is critical. Use appropriate statistical techniques and software tools to help with data cleaning. Document the steps taken during data cleaning for transparency, reproducibility, and future reference.
Before diving into complex analysis techniques, exploratory data analysis (EDA) is required. EDA allows you to gain a thorough understanding of the dataset, identify underlying patterns, and gain preliminary insights. Explore distributions, correlations, and outliers by visualizing the data with graphs, charts, and summary statistics. You will be better equipped to choose appropriate analysis methods for your assignment if you thoroughly explore the dataset's characteristics and relationships. EDA provides useful insights that will lead you to a more precise and targeted analysis approach.
Choosing the Best Analytical Techniques:
When you have a short deadline for data analysis homework, choosing the best analysis techniques is critical. Consider the assignment's objectives as well as the nature and characteristics of the data. Choose analysis techniques that are relevant to the research questions or goals of your assignment. Whether it is regression analysis, hypothesis testing, clustering, or another method, make sure that the techniques used are appropriate for the data and research objectives. Consider the assumptions and limitations of each technique before making an informed decision on which methods will produce the most accurate and meaningful results within the timeframe specified. Consulting textbooks, research papers, or looking for online resources can help you choose the best analysis techniques for your assignment.
Effective Data Analysis and Clear and Concise Reporting:
Once you've decided on the best analysis techniques, it's time to put them into action. Using software tools like R, Python, or statistical software packages can greatly simplify your analysis process. To avoid confusion and errors, it is critical to write well-documented and organized code. Breaking down complex analysis steps into smaller, more manageable tasks can help ensure accuracy and reliability by verifying intermediate results at each stage. To ensure the robustness of your analysis, pay close attention to parameter tuning and sensitivity analysis. Always interpret your findings in the context of the assignment objectives and draw meaningful conclusions from them.
Your data analysis findings must be effectively communicated in a clear and concise manner. Prepare an organized report that summarizes your findings, interpretations, and conclusions. Make use of visualizations, tables, and charts to present your findings in an appealing and understandable manner. Explain the implications of your findings and make any relevant recommendations or suggestions for additional research. Before submitting your report, thoroughly proofread it to ensure that there are no grammatical or typographical errors. Incorporating a section on limitations and potential future directions can give you a more complete picture of your analysis.
Conclusion:
Handling data analysis homework with a short deadline can be a daunting task, but success is attainable with proper planning, effective time management, and a systematic approach. You can overcome the challenges and achieve your desired results by gaining a thorough understanding of the assignment, planning and prioritizing tasks, managing time efficiently, and following a structured process encompassing data collection, cleaning, analysis, and reporting. Remember to stay focused and diligent, and to seek help or clarification as needed. Best wishes for your data analysis assignments.