- Grasping the Essence of Data Cleaning
- A Symphony of Precision
- The Crucible of Authenticity
- The Pillar of Reliability
- Navigating the Landscape of Outliers
- Confronting the Abyss of Missing Data
- Championing Credibility
- The Battle Against Bias
- Conclusion
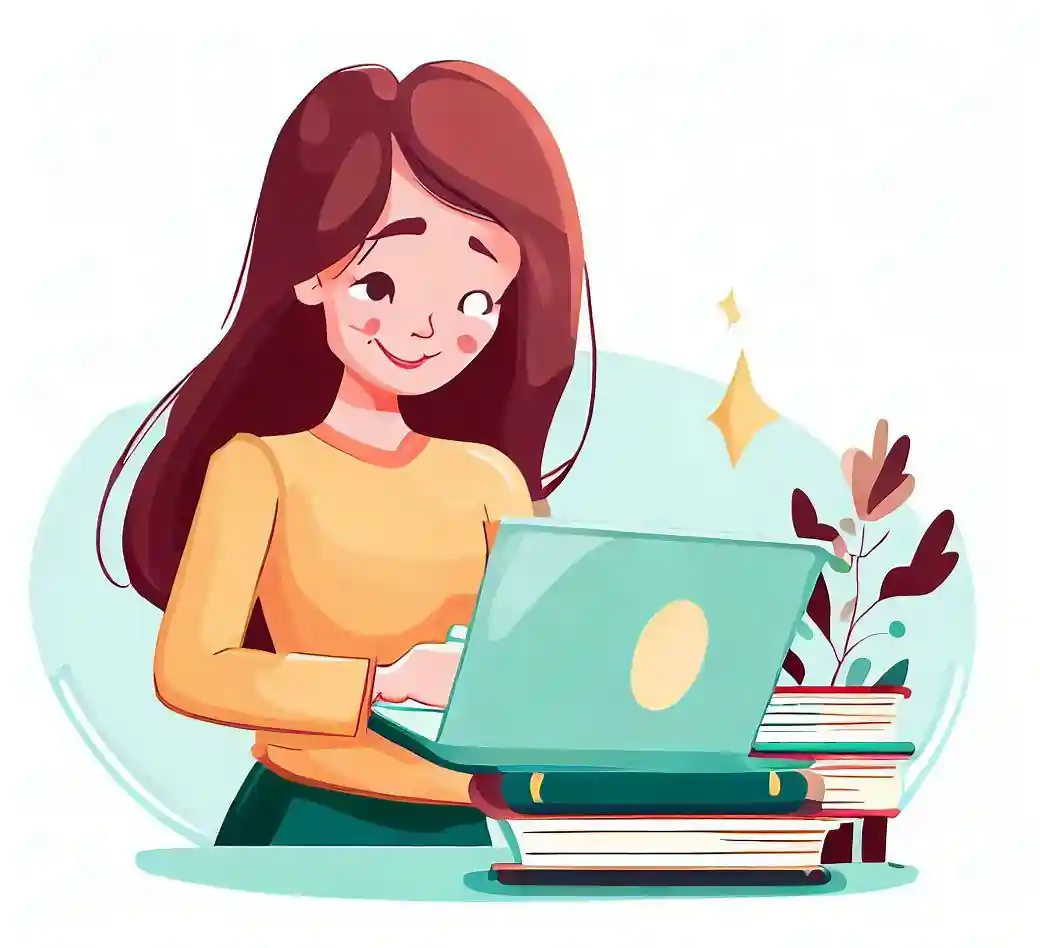
In an era where data flows ceaselessly from an array of sources, ranging from social media interactions to scientific experiments, harnessing this deluge of information for valuable insights has become the cornerstone of modern decision-making. Amidst this data revolution, the importance of data quality cannot be overstated, especially when completing your statistics homework. At the heart of this data quality assurance process lies the often-underestimated practice of data cleaning, a pivotal step in data analysis, particularly within the domain of statistics. In this comprehensive exploration, we unravel the profound significance of data cleaning in the context of your statistics assignments, dissecting its role in elevating accuracy, bolstering reliability, and fortifying the overall credibility of your analytical endeavors.
Grasping the Essence of Data Cleaning
At the nucleus of every data-driven endeavor lies the practice of data cleaning, a process akin to a virtuoso performance in the symphony of statistics. This methodological masterpiece, also known as data cleansing or data scrubbing, encompasses a meticulous choreography of identifying, rectifying, and mitigating the variegated errors, inconsistencies, and inaccuracies that often inhabit datasets. Like a seasoned detective, data cleaning unveils hidden secrets, rectifies fallacies, and orchestrates data harmony. Delving into the depths of this process reveals its multifaceted significance in the realm of statistics.
A Symphony of Precision
Data, raw and unprocessed, is akin to a raw gemstone with untapped brilliance. Data cleaning, the meticulous lapidary process, unveils its true potential. Think of it as the art of deciphering patterns in a chaotic tableau. Its essence lies in unraveling the tangled threads of errors that can emerge from the most unexpected sources: a keystroke error by a hurried data entry, an errant digit resulting from a technical hiccup, or a minuscule measurement discrepancy with outsized repercussions. The vigilant scrutiny data cleaning entails ensures that these glitches are not overlooked, but are rather unearthed, rectified, and mitigated.
The Crucible of Authenticity
Errors lurking within datasets are akin to shadows, casting doubt upon the authenticity and precision of the analysis. Imagine conducting a study on the correlation between sleep patterns and academic performance, only to realize that the very foundation of your analysis rests upon data inaccuracies. The integrity of your findings hinges on accurate data. Data cleaning, then, emerges as the sentinel guarding against distorted conclusions. With an eagle-eyed focus on data entries, data cleaning adeptly identifies anomalies, outliers, and disparities. By rectifying these errors, data cleaning forges a resilient dataset, which forms the bedrock for robust statistical analysis. The insights drawn are not built upon quicksand, but rather upon the solid rock of accurate data.
The Pillar of Reliability
In the realm of statistics, reliability stands as the beacon guiding the way through the murky waters of data analysis. Reliability encompasses the stability and consistency of measurements or observations—a cornerstone for any meaningful analysis. Yet, the presence of inconsistent or erroneous data can dismantle this pillar of reliability, rendering an analysis futile. This is where data cleaning assumes its role as the guardian of data's sanctity. It eradicates sources of bias and variability rooted in flawed data, thus augmenting the reliability of the subsequent analysis. This is especially crucial in assignments that demand precision, where decisions made based on unreliable data can have far-reaching consequences.
Navigating the Landscape of Outliers
Outliers, those enigmatic data points that defy convention by deviating significantly from the rest, pose a formidable challenge in statistical analysis. They are akin to rare gems that can either reveal profound insights or distort the entire narrative. Data cleaning is the compass navigating this intricate landscape. While some outliers might indeed offer windows into extraordinary phenomena, others can stem from errors, anomalies, or even misinterpretations. Ignoring outliers can skew vital statistical metrics, clouding interpretations. Data cleaning scrutinizes these outliers, distinguishing between genuine revelations and errors, thus ensuring that only those true to the dataset's essence are retained.
Confronting the Abyss of Missing Data
Data analysis often treads into the realm of the incomplete, where gaps in data—missing pieces of the puzzle—create a void that can jeopardize the integrity of results. The chasm of missing data is universal, stemming from non-responses, technical hiccups, or other glitches. Data cleaning wades bravely into this abyss with an arsenal of techniques, such as imputation, which involves filling in missing values based on existing data. This strategic approach mitigates the impact of missing data, resulting in analyses conducted on a more comprehensive dataset, fortified against bias and skewed outcomes.
Championing Credibility
In academia and research, credibility is the lifeblood of knowledge dissemination. A statistics assignment bereft of meticulous data cleaning is akin to presenting a masterpiece obscured by a veil of doubt. Its rigor and authenticity come into question. Yet, by embarking on a thorough data-cleaning regimen, you showcase a commitment to generating precise, dependable results. This is especially pivotal when your findings influence pivotal conversations or shape decision-making processes. Data cleaning, in this context, is not just a process; it's a declaration of dedication to the pursuit of truth.
- The Veil of Doubt
- The Dance of Rigor and Authenticity
- The Power of Influence
- The Declaration of Truth
Imagine embarking on a journey to unveil a masterpiece—a profound analysis forged from data, and insights that possess the potential to illuminate paths previously untrodden. Now, picture this masterpiece shrouded in a thick veil of doubt, its brilliance obscured by the lurking shadows of inaccuracies and inconsistencies. Such is the fate of a statistics assignment that neglects the meticulous process of data cleaning.
Without data cleaning, an analysis stands vulnerable to the skepticism that arises when doubts cloud its credibility. Errors, biases, and inaccuracies that often weave themselves into datasets cast suspicion on the authenticity of the findings. As doubts grow, the entire analysis becomes an exercise in uncertainty rather than a beacon of knowledge.
At the heart of data cleaning lies an unwavering dedication to rigor and authenticity. It's not just about the numbers, but about the commitment to delivering results that are founded on a bedrock of accurate data. By engaging in data cleaning, researchers and scholars affirm their allegiance to the principles of excellence and precision.
A thorough data-cleaning regimen is akin to painstakingly restoring a centuries-old painting. Each brushstroke is not just a movement; it's a declaration of dedication to restoring the masterpiece's authenticity. Similarly, each correction, each validation, and each adjustment made during data cleaning is a testament to the researcher's commitment to generating results that can be trusted.
The importance of data cleaning amplifies when the implications of research findings are far-reaching. In contexts where research fuels pivotal conversations or shapes decision-making processes, credibility is not just desirable—it's imperative. Consider policy decisions that are formulated based on statistical analyses or scientific breakthroughs that redefine paradigms. In these scenarios, the credibility of the research findings can make the difference between sound decisions and misguided choices.
A well-executed data-cleaning process ensures that the analysis can withstand scrutiny. When findings are based on a dataset that has been meticulously cleansed of errors and biases, their power to influence decisions is magnified. The clarity of insight is not clouded by doubts, and the credibility of the research becomes a beacon that guides decision-makers toward informed choices.
Data cleaning, in this profound context, becomes more than a process—it's a declaration of dedication to the pursuit of truth. It's a statement that the integrity of knowledge matters, and that the pursuit of accurate insights transcends mere formality. Data cleaning asserts that knowledge is not just a commodity but a responsibility—one that necessitates an unyielding commitment to rigor and authenticity.
As the digital age accelerates the pace of knowledge generation, the importance of credibility remains steadfast. In a world where information flows ceaselessly, where knowledge is exchanged across boundaries, the role of data cleaning in championing credibility takes on renewed significance. It transforms data from a muddled stream into a clear, pristine river of knowledge—one that can be trusted, referenced, and built upon.
The Battle Against Bias
The annals of data analysis are replete with tales of bias lurking in datasets. Biases can creep in from myriad sources—skewed sample selection, measurement peculiarities, or even human fallibility. These biases can clandestinely manipulate statistical outcomes, rendering them a mere reflection of bias rather than an objective representation of reality. Data cleaning emerges as the gallant knight in this ceaseless battle against bias. Armed with scrutiny and cleansing techniques, it embarks on a quest to mitigate bias, ensuring that findings are more universally applicable and reflective of the broader population.
- The Spectrum of Bias
- The Subversion of Objectivity
- Data Cleaning: The Unsung Hero
- The Quest for Universality
Bias, much like a shape-shifting specter, can take on various forms, lurking unnoticed in the very data we seek to analyze. One of its many guises is selection bias, where the sample chosen for analysis is not representative of the broader population, thus skewing the results. Imagine studying the dietary habits of a community by surveying only the most health-conscious members. The conclusions drawn would be inherently biased, failing to reflect the diversity of eating behaviors within the community.
Measurement bias, another form, stems from the very instruments used to collect data. These instruments, while reliable, may inadvertently introduce inaccuracies due to technical limitations or misinterpretations. An example is using a thermometer calibrated incorrectly to measure temperatures, leading to distorted results.
Cognitive bias, a more subtle variety, emanates from the imperfections of human perception and judgment. Confirmation bias, for instance, occurs when researchers unintentionally seek or interpret data in a way that confirms their preconceived notions. This can inadvertently shape the outcomes of analysis, compromising objectivity.
The impact of bias is far-reaching, altering the course of analysis by tilting the scales in favor of certain outcomes. When bias goes unchecked, statistical results cease to be an honest representation of reality. Instead, they mirror the distortion introduced by biases, rendering the analysis tainted and unreliable. This subversion of objectivity undermines the credibility of findings, which can have profound implications in decision-making processes, policy formulation, and scientific advancements.
In this tumultuous battle against bias, data cleaning emerges as the unsung hero—the gallant knight armed with an arsenal of techniques designed to confront and mitigate bias. Data cleaning is not mere janitorial work but a strategic maneuver to rectify the imbalances introduced by biases. By meticulously identifying, addressing, and mitigating the sources of bias within a dataset, data cleaning paves the way for more impartial, reliable, and robust analyses.
In its quest for universality, data cleaning is guided by a singular purpose: to ensure that the insights drawn from data are representative of the broader population, unaffected by the shadows of bias. It scrutinizes sample selection methods, striving to create samples that mirror the diversity of the entire population, not just a select subset. It recalibrates measurement techniques, striving to eliminate inaccuracies and distortions that might arise due to the instruments' limitations. It invites a diversity of perspectives, guarding against cognitive biases that can inadvertently sway interpretations.
Through these efforts, data cleaning transforms itself into a beacon of fairness, illuminating the path toward more objective and equitable analyses. It allows statistical outcomes to transcend the limitations of bias, emerging as authentic reflections of reality. By undertaking this arduous battle against bias, data cleaning imbues analyses with an aura of authenticity, elevating the credibility of findings and making them more potent instruments for informed decision-making.
Conclusion
Amidst the labyrinthine corridors of statistical analyses, data cleaning stands as a sentinel of truth. Far from being a perfunctory chore, data cleaning emerges as a critical linchpin that elevates the accuracy, reliability, and credibility of your analytical undertakings. Through painstaking data-cleaning endeavors, the raw, potentially blemished data metamorphoses into a dependable bedrock upon which insightful conclusions are forged. Whether you're unraveling trends, summoning predictions, or subjecting hypotheses to empirical scrutiny, the very bedrock of your data-driven journey pivots upon the fulcrum of data cleaning. Hence, as you embark upon your next statistics assignment, bear in mind the indomitable significance of data cleaning—it's the clarion call that unlocks the latent potential harbored within your data realms.