- Excel Functions for Time Series Data
- Moving Averages
- Exponential Smoothing
- Trend Analysis and Seasonal Decomposition
- Trend Analysis in Excel
- Seasonal Decomposition in Excel
- Forecasting Techniques in Excel
- Time Series Forecasting with Excel
- Evaluating Forecast Accuracy in Excel
- Advanced Time Series Analysis Techniques
- Autocorrelation and Partial Autocorrelation
- Box-Jenkins Methodology
- Conclusion:
Time series analysis stands as a cornerstone skill for students venturing into diverse fields such as statistics, finance, economics, and an array of other disciplines. The ability to comprehend and interpret time-dependent data is of paramount importance, as it not only provides insights into historical patterns but also serves as a foundation for predicting future trends. However, the complexity of time series data can pose a challenge to beginners, necessitating the adoption of effective tools and methodologies to unravel its intricacies. In the realm of academic pursuits, mastering time series analysis is not merely a theoretical exercise; it is a practical necessity. The significance of this skill becomes evident when students are faced with assignments and projects that demand a comprehensive understanding of trends, patterns, and the ability to make informed forecasts. Whether it's unraveling the economic dynamics of a region, predicting stock market trends, or understanding the impact of time on various variables, time series analysis is an indispensable tool in the toolkit of a well-rounded student. Whether you need assistance with your Excel homework or are navigating the complexities of time series analysis, mastering Excel for data manipulation and visualization is crucial for effectively analyzing and presenting time series data. The focus of this blog is to provide a guiding light for beginners navigating the complexities of time series analysis, specifically within the familiar confines of Microsoft Excel. Excel, being a ubiquitous and accessible tool, levels the playing field for students regardless of their familiarity with complex statistical software. The blog aims to bridge the gap between theoretical concepts and practical applications, offering insights and techniques that not only facilitate understanding but also empower students to apply these skills to real-world scenarios.
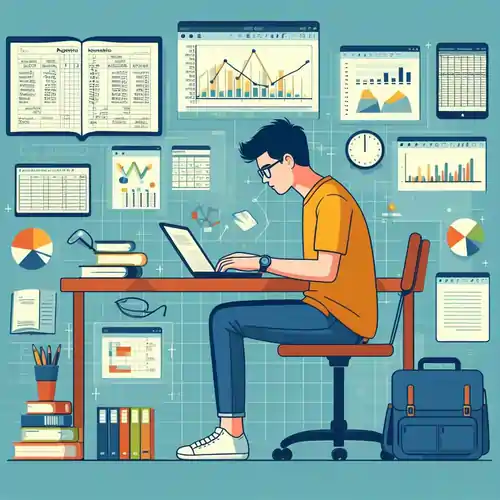
Time series data, by its nature, involves the recording of observations over a continuum, creating a chronological sequence that becomes the canvas for analysis. This chronological ordering enables the exploration of trends and patterns, giving rise to the ability to make predictions about future occurrences. It is within this chronological context that Excel emerges as a powerful ally for students. Its user-friendly interface and wide-ranging functionalities make it an ideal platform for delving into time series analysis without the steep learning curve associated with more specialized statistical software.
Excel Functions for Time Series Data
Time series data often exhibits inherent fluctuations, and uncovering meaningful trends within this complexity is essential for accurate analysis. In the realm of Excel, a versatile and widely-used tool, specific functions cater to the unique requirements of time series data analysis. This section will delve into the Excel functions designed for time series data, shedding light on two key techniques: Moving Averages and Exponential Smoothing.
Moving Averages
Moving averages stand as a cornerstone in the arsenal of tools for time series analysis. The primary objective is to smooth out the inherent noise and fluctuations in the data, making discernible patterns and trends more apparent to the analyst. In the context of Excel, the calculation of moving averages can be executed through two main methods. The first and more straightforward method involves utilizing the built-in AVERAGE function in Excel. Students can seamlessly compute the moving average by selecting a specific window of data points and applying the AVERAGE function, which calculates the mean of the selected range.
This simple yet effective technique aids in visualizing trends by reducing the impact of short-term fluctuations. For those seeking a more advanced approach, Excel offers the Data Analysis ToolPak, an add-in that provides an array of powerful tools for data analysis, including moving averages. This tool enables students to compute various types of moving averages, such as simple, weighted, or exponential moving averages, depending on the specific characteristics of their time series data.
Exponential Smoothing
Moving averages stand as a cornerstone in the arsenal of tools for time series analysis. The primary objective is to smooth out the inherent noise and fluctuations in the data, making discernible patterns and trends more apparent to the analyst. In the context of Excel, the calculation of moving averages can be executed through two main methods. The first and more straightforward method involves utilizing the built-in AVERAGE function in Excel. Students can seamlessly compute the moving average by selecting a specific window of data points and applying the AVERAGE function, which calculates the mean of the selected range.
This simple yet effective technique aids in visualizing trends by reducing the impact of short-term fluctuations. For those seeking a more advanced approach, Excel offers the Data Analysis ToolPak, an add-in that provides an array of powerful tools for data analysis, including moving averages. This tool enables students to compute various types of moving averages, such as simple, weighted, or exponential moving averages, depending on the specific characteristics of their time series data.
Trend Analysis and Seasonal Decomposition
Time series data, with its inherent sequential structure, often conceals valuable insights beneath the surface. Trend analysis and seasonal decomposition are pivotal techniques in time series analysis, unraveling the patterns hidden within the chronological flow of data. In this section, we will delve into the capabilities of Excel in performing trend analysis and seasonal decomposition, empowering students to extract meaningful information from their datasets.
Trend Analysis in Excel
One of the fundamental aspects of time series analysis is the identification and interpretation of trends within the data. This task is pivotal for understanding the underlying patterns that can influence future outcomes. In Excel, students have access to versatile tools, including linear regression and the TREND function, which play a crucial role in uncovering these trends.
Linear Regression in Excel
Linear regression is a statistical method that helps us understand the relationship between two variables—specifically, how one variable changes concerning another. In the context of time series analysis, linear regression allows students to model the trend within their data. Excel simplifies this process by providing a user-friendly interface for implementing linear regression. By utilizing the built-in regression analysis tools, students can fit a linear model to their time series data, revealing the overall trend over time.
The TREND Function
Excel's TREND function is a powerful tool for time series trend analysis. It enables students to extend an existing trendline into the future, predicting future values based on historical data. By understanding how to use the TREND function, students can make informed projections and gain insights into the potential direction of their time series data. This function not only aids in forecasting but also provides a visual representation of the identified trend through the generated trendline on a graph.
Seasonal Decomposition in Excel
In time series data, it's not uncommon to encounter recurring patterns or seasonality. Seasonal effects can manifest on a monthly, quarterly, or yearly basis, and recognizing and isolating these components is crucial for a comprehensive understanding of the data. Excel's Seasonal Decomposition of Time Series (STL) tool proves to be an invaluable resource for students seeking to disentangle these seasonal components from the overall trend.
Understanding Seasonal Decomposition
Seasonal decomposition involves breaking down a time series into its underlying components, namely trend, seasonality, and remainder (residuals). By identifying and isolating the seasonal component, analysts can better comprehend the cyclical patterns that repeat at regular intervals. Excel's STL tool automates this process, providing a structured approach to extracting seasonal effects from time series data.
Implementation of STL in Excel
This section will delve into the practical application of the STL tool in Excel, guiding students through the steps to perform seasonal decomposition. Understanding the nuances of this tool is crucial for accurately interpreting time series data, especially when faced with recurring patterns that can impact the overall analysis. Real-world examples will be used to illustrate how to apply STL effectively, demonstrating its significance in uncovering the seasonality within time series datasets.
Forecasting Techniques in Excel
Forecasting is a pivotal aspect of time series analysis, equipping students with the ability to predict future values based on historical data. In this section, we will delve into the forecasting techniques available in Excel, emphasizing the importance of understanding tools such as the FORECAST and TREND functions.
Time Series Forecasting with Excel
Time series forecasting in Excel involves leveraging powerful functions designed to analyze historical data and project future trends. The FORECAST function is a fundamental tool that allows students to estimate a future value based on a linear trend observed in past data points. By providing the function with the historical x and y values, students can predict the y value for a given x in the future. The TREND function is another indispensable tool for time series forecasting in Excel. Unlike the FORECAST function, TREND extends beyond linear relationships, accommodating polynomial trends. This function is particularly useful when dealing with data that exhibits non-linear patterns.
Students can use TREND to generate forecasts that align with more complex trends, enhancing the accuracy and applicability of their predictions. Understanding these forecasting functions is vital for students, as it equips them with the capability to make informed predictions in diverse academic and professional contexts. Whether in finance, economics, or business analytics, the ability to forecast future values is a valuable skill that sets individuals apart in decision-making processes.
Evaluating Forecast Accuracy in Excel
While making forecasts is crucial, ensuring their accuracy is equally essential. Excel provides tools for students to evaluate the reliability of their predictions, offering insights into the effectiveness of forecasting models. In this context, metrics such as Mean Absolute Error (MAE) and Root Mean Squared Error (RMSE) play a pivotal role. Mean Absolute Error (MAE) is a metric that quantifies the average magnitude of errors between predicted and actual values. Students can calculate MAE by taking the absolute difference between each predicted and actual value, then averaging these differences. A lower MAE indicates a more accurate forecasting model.
Root Mean Squared Error (RMSE) is another metric that evaluates forecast accuracy by measuring the square root of the average of squared errors. RMSE gives more weight to larger errors, providing a comprehensive assessment of forecasting model performance. Like MAE, a lower RMSE signifies a more accurate prediction. This section guides students on how to use these metrics effectively in Excel. By comparing forecasted values with actual outcomes, students can calculate MAE and RMSE, gaining insights into the strengths and weaknesses of their forecasting models. This evaluation process is crucial for refining models, enhancing predictive accuracy, and building confidence in the forecasting results.
Advanced Time Series Analysis Techniques
Time series analysis becomes more intricate as we delve into advanced techniques, offering students a deeper understanding of the intricate relationships within sequential data. Two pivotal techniques in this realm are Autocorrelation and Partial Autocorrelation, and the Box-Jenkins Methodology, commonly known as ARIMA modeling.
Autocorrelation and Partial Autocorrelation
In the realm of time series analysis, Autocorrelation and Partial Autocorrelation functions stand as indispensable tools for unraveling the underlying structure of sequential data. Autocorrelation, in essence, measures the correlation between a time series and a delayed or lagged version of itself. In simpler terms, it helps identify patterns that repeat at various intervals. Using Excel to calculate autocorrelation functions empowers students to visually and quantitatively discern the relationships between data points at different time lags. Excel's built-in functions simplify the computation of autocorrelation coefficients, allowing students to assess the degree of correlation between observations at varying time intervals. This proficiency not only enhances their analytical skills but also aids in recognizing trends and cyclic patterns within time series data.
Partial Autocorrelation takes the analysis a step further by considering the correlation between data points while removing the effects of the intermediate time lags. This nuanced approach allows students to discern more refined dependencies within the data, enabling a deeper understanding of the underlying temporal patterns. Excel's user-friendly interface facilitates the calculation and interpretation of partial autocorrelation functions, providing students with a valuable tool for unraveling complex time series dependencies.
Box-Jenkins Methodology
The Box-Jenkins Methodology, often synonymous with ARIMA modeling, presents a comprehensive and systematic approach to time series analysis. ARIMA stands for Autoregressive Integrated Moving Average, encapsulating the three key components of this methodology.
- Autoregressive (AR): This component captures the relationship between an observation and several lagged observations. Excel allows students to implement ARIMA modeling by using functions like CORREL to calculate autocorrelations and build autoregressive components, enhancing their ability to model dependencies in their time series data.
- Integrated (I): Integration involves differencing the time series data to make it stationary. Stationary data simplifies the modeling process by removing trends or seasonality. Excel's Data Analysis ToolPak provides students with the tools to perform differencing effortlessly, streamlining the application of the Box-Jenkins Methodology.
- Moving Average (MA): The moving average component captures the correlation between an observation and a residual error from a moving average model. In Excel, students can utilize the Analysis ToolPak to calculate moving averages, facilitating the integration of this component into their ARIMA models.
Conclusion:
Mastering time series analysis using Excel is not just a skill; it is a gateway to a myriad of opportunities for students across various disciplines. Excel, a widely accessible and user-friendly platform, offers a spectrum of tools that cater to the needs of beginners delving into the intricate world of time-dependent data. The significance of this mastery becomes apparent as students progress from basic techniques like moving averages to the more sophisticated methods such as ARIMA modeling. At the foundational level, Excel's moving averages feature stands as a fundamental tool in the time series analysis toolkit. This technique, easily implemented through the AVERAGE function or the Data Analysis ToolPak, serves to smooth out fluctuations within data.