Claim Your Discount Today
Get 10% off on all Statistics homework at statisticshomeworkhelp.com! Whether it’s Probability, Regression Analysis, or Hypothesis Testing, our experts are ready to help you excel. Don’t miss out—grab this offer today! Our dedicated team ensures accurate solutions and timely delivery, boosting your grades and confidence. Hurry, this limited-time discount won’t last forever!
We Accept
- Importing and Preparing Time Series Data in SPSS
- Data Exploration and Visualization
- Time Series Decomposition
- Basic Time Series Models in SPSS
- Autoregressive Integrated Moving Average (ARIMA) Models
- Exponential Smoothing Models
- Advanced Time Series Analysis Techniques in SPSS
- Seasonal Decomposition of Time Series (STL)
- Dynamic Factor Analysis (DFA)
- Evaluating and Interpreting Time Series Models
- Model Diagnostics and Residual Analysis
- Forecasting and Prediction Intervals
- Conclusion
Time series analysis, a robust statistical method, stands as a cornerstone in understanding the dynamics of sequential data points gathered over time. Its applications span a multitude of disciplines, permeating through economics, finance, biology, and environmental science. The ability to discern patterns, unveil trends, and extract valuable insights from time-dependent data is crucial in making informed decisions and predictions. For students venturing into the intricate realm of time series analysis, the Statistical Package for the Social Sciences (SPSS) emerges as an indispensable tool, providing a structured and user-friendly environment for conducting in-depth analyses. In this comprehensive guide, we embark on an exploration of the fundamental concepts of time series analysis in SPSS, aiming to empower students with the skills necessary to approach assignments with confidence. At its core, time series analysis involves the meticulous study of the inherent patterns and trends within data collected over successive time intervals. Unlike cross-sectional data, time series data introduces a temporal dimension, where the order of observations is of paramount importance. The ability to decipher the chronology of events aids in uncovering meaningful relationships and understanding the evolution of variables over time. If you need help with your SPSS homework, delving into time series analysis concepts in SPSS can provide valuable insights and support to tackle your assignments effectively. The journey into time series analysis within SPSS commences with the pivotal step of data importation and exploration. SPSS facilitates this process by accommodating various file formats, such as Excel and CSV, ensuring compatibility with diverse datasets. Once the data is imported, the exploration phase becomes paramount. It involves scrutinizing the dataset for completeness, consistency, and potential outliers. This preliminary examination lays the foundation for subsequent analyses, guiding students towards a nuanced understanding of the data they are working with. Understanding the nature of time-dependent data is the first critical aspect of time series analysis. Recognizing the sequential order of observations is imperative for accurate modeling and interpretation. SPSS streamlines this process, providing tools for visualizing time series data through line charts and scatter plots. Exploratory data analysis techniques offered by SPSS empower students to identify trends, seasonality, and potential outliers, enabling them to make informed decisions about the subsequent steps in their analysis.
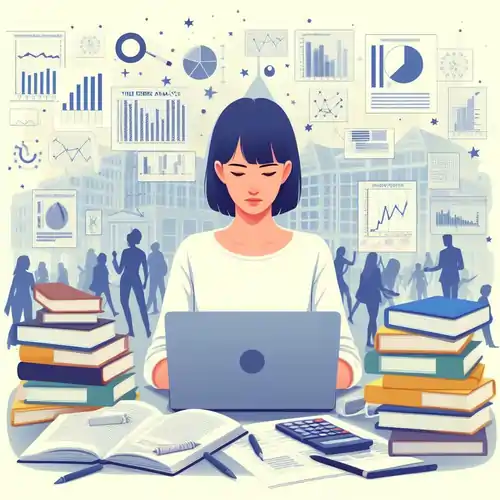
Importing and Preparing Time Series Data in SPSS
Embarking on the journey of time series analysis within SPSS requires meticulous attention to the initial step: importing the data. SPSS stands out for its versatility, supporting various file formats, such as Excel and CSV. This flexibility empowers users to seamlessly work with diverse datasets, a crucial feature given the wide array of data types encountered in academic and professional settings.
Data Exploration and Visualization
Before diving into the intricacies of time series analysis, an indispensable preliminary step is visualizing the data. SPSS caters to this need with a robust set of tools, offering features such as line charts and scatter plots. The goal of exploratory data analysis at this stage is to gain an intuitive understanding of the dataset's dynamics. In the realm of time series analysis, visualizations serve as the compass guiding researchers through the data landscape. Line charts, for instance, vividly portray the trends and patterns embedded within the temporal sequence. Scatter plots become invaluable in highlighting potential relationships and identifying outliers that might significantly impact subsequent analyses.
Moreover, the visual exploration phase is not merely about aesthetics. It's a strategic step in identifying nuances within the data, such as seasonality or irregularities, that may influence the choice of analytical approaches. This visual foundation sets the stage for more informed and targeted analytical decisions.
Time Series Decomposition
As the data exploration unfolds, the focus naturally shifts to understanding the intricate components of the time series dataset. Time series decomposition is a pivotal technique facilitated by SPSS to unravel the underlying elements – trend, seasonality, and noise. SPSS simplifies the decomposition process through dedicated procedures, streamlining the isolation of individual components. The ability to dissect the time series into its constituent parts is invaluable. It allows students to discern the long-term trends shaping the data, seasonal fluctuations occurring over specific periods, and the noise or irregularities that might obscure the true patterns.
This decomposition not only enhances comprehension but also influences subsequent modeling decisions. Armed with a nuanced understanding of the data's composition, students can make informed choices about the types of models that best capture and represent these components. Whether predicting future trends or understanding historical patterns, time series decomposition becomes the bedrock for robust and accurate analyses.
Basic Time Series Models in SPSS
After completing the initial steps of importing and preparing time series data in SPSS, the next phase for students involves the construction of basic time series models. This stage is pivotal, as it sets the foundation for more advanced analyses and insights. Choosing the right model is critical and depends on the specific characteristics of the data and the objectives of the analysis.
Autoregressive Integrated Moving Average (ARIMA) Models
One of the fundamental and widely utilized tools in time series analysis is the Autoregressive Integrated Moving Average (ARIMA) model. ARIMA models are particularly valuable for forecasting future values based on historical trends within the data. SPSS streamlines the construction of ARIMA models, making it accessible for students with varying levels of statistical expertise. ARIMA models consist of three components: autoregressive (AR), differencing (I), and moving average (MA). The autoregressive component captures the relationship between an observation and its preceding values, the differencing component addresses non-stationarity by transforming the data, and the moving average component accounts for the correlation between a current observation and a residual from previous observations.
SPSS facilitates the implementation of ARIMA models through an intuitive interface, allowing students to input parameters, choose appropriate lag values, and fine-tune the model to fit their specific dataset. The software also provides diagnostic tools to evaluate the model's performance, enabling students to identify potential issues and refine their models for more accurate predictions. Understanding the intricacies of ARIMA models is crucial for students aiming to make informed decisions based on time series data. Mastery of this technique empowers them to not only forecast future values but also to comprehend the underlying patterns and trends within the data, contributing to a comprehensive analysis.
Exponential Smoothing Models
In addition to ARIMA models, another essential tool in the arsenal of basic time series models is Exponential Smoothing. Specifically, the Holt-Winters method is a valuable approach for capturing trends and seasonality within time series data. SPSS offers user-friendly interfaces that simplify the implementation of Exponential Smoothing Models, making it accessible for students with varying levels of statistical proficiency. Exponential smoothing models work by assigning exponentially decreasing weights to past observations, giving more importance to recent data points. The Holt-Winters method, in particular, introduces components for level, trend, and seasonality, making it suitable for datasets that exhibit these patterns.
SPSS guides students through the process of configuring Exponential Smoothing Models, allowing them to adjust parameters and assess the model's performance. This hands-on experience is invaluable for students seeking a practical understanding of how these models operate and contribute to forecasting future values. As students delve into basic time series models like ARIMA and Exponential Smoothing in SPSS, they not only gain proficiency in utilizing the software but also develop a deeper comprehension of the temporal dynamics inherent in their data. This foundational knowledge prepares them for more advanced analyses and equips them with the skills necessary for making informed decisions in a variety of academic and professional scenarios.
Advanced Time Series Analysis Techniques in SPSS
As students delve deeper into the realm of time series analysis within the Statistical Package for the Social Sciences (SPSS), they are presented with a myriad of advanced techniques that elevate their analytical capabilities. These sophisticated methods not only enrich their understanding of temporal patterns but also provide nuanced insights into the complexities of time series data. This section explores two pivotal techniques in SPSS: Seasonal Decomposition of Time Series (STL) and Dynamic Factor Analysis (DFA).
Seasonal Decomposition of Time Series (STL)
Seasonal Decomposition of Time Series, abbreviated as STL, stands out as a robust method for dissecting time series data into distinct components, namely trend, seasonality, and residual elements. The integration of STL algorithms within SPSS empowers students to embark on in-depth analyses, unraveling the intricate structures embedded in their temporal data. STL addresses a fundamental challenge in time series analysis—the need to disentangle various components contributing to observed patterns. By decomposing the time series into trend, seasonality, and residuals, students gain a comprehensive understanding of how these elements interact and influence the overall data dynamics.
Within SPSS, the implementation of STL is user-friendly, allowing students to easily apply this technique to their datasets. Visualization tools in SPSS further enhance the interpretability of the results, enabling students to grasp the cyclical patterns, long-term trends, and irregularities inherent in their time series data. The insights derived from STL are invaluable for decision-making and forecasting. For instance, identifying seasonal fluctuations in sales data or understanding the recurring patterns in environmental variables becomes more achievable through the application of STL in SPSS. Students can confidently utilize this technique to extract meaningful information from their time series data, laying the groundwork for informed analysis and strategic planning.
Dynamic Factor Analysis (DFA)
Dynamic Factor Analysis (DFA) represents a significant advancement in time series analysis, extending beyond traditional methodologies by considering latent factors that exert influence across multiple time series simultaneously. SPSS seamlessly integrates DFA capabilities, providing students with a powerful tool to explore the intricate interdependencies between different variables over time. In traditional time series analysis, each variable is typically examined in isolation. DFA, however, acknowledges that various factors may simultaneously impact multiple variables, introducing a more realistic and nuanced perspective. This proves particularly crucial in domains where variables interact and influence each other, such as economics, finance, and epidemiology.
SPSS simplifies the application of DFA, allowing students to model complex relationships between variables and uncover hidden patterns that may not be apparent through individual analyses. By considering latent factors, students gain a more holistic view of the underlying dynamics driving their time series data. The incorporation of DFA in SPSS aligns with the evolving nature of data analysis, where researchers and analysts seek methods that capture the complexity of real-world scenarios. Students utilizing DFA in SPSS not only enhance their analytical toolkit but also contribute to the advancement of methodologies that can address the intricacies of contemporary datasets.
Evaluating and Interpreting Time Series Models
Upon the completion of constructing time series models, students enter a critical phase – the evaluation and interpretation of their models. This process is integral to ensuring the reliability and effectiveness of the chosen models. In this section, we will delve into the tools and metrics provided by SPSS for thorough model assessment.
Model Diagnostics and Residual Analysis
A cornerstone of evaluating time series models lies in comprehending the residuals, which are the differences between the observed and predicted values. SPSS, as a robust statistical tool, offers an array of diagnostic tools and visualizations to facilitate this critical analysis. Residuals are a reflection of the model's accuracy, and understanding their patterns and trends is essential for model validation. SPSS's diagnostic tools include residual plots, autocorrelation functions, and Ljung-Box tests. Residual plots provide a visual representation of the discrepancies between actual and predicted values across different time points. Analyzing these plots helps identify any systematic patterns or outliers in the residuals, offering insights into potential model deficiencies.
Autocorrelation functions assist in assessing the independence of residuals over time. A lack of autocorrelation indicates that the model has effectively captured the temporal patterns in the data. On the other hand, significant autocorrelation might suggest overlooked patterns or inadequacies in the model. The Ljung-Box test is a statistical method employed to examine whether the residuals exhibit significant temporal dependencies. Conducted on lagged residuals, this test aids in identifying if there are any remaining patterns or information not captured by the model. SPSS automates the execution of these tests, streamlining the diagnostic process for students.
Forecasting and Prediction Intervals
As students progress in evaluating time series models, the next pivotal aspect is forecasting and prediction intervals. SPSS simplifies this process by providing tools to generate forecasted values along with associated prediction intervals. These intervals play a crucial role in conveying the uncertainty inherent in any forecasting endeavor. Forecasting values alone provide a point estimate of future observations, but prediction intervals offer a range within which these values are likely to fall. SPSS calculates these intervals based on the inherent variability and uncertainty in the time series data.
The width of the prediction interval reflects the degree of uncertainty associated with the forecasts – a wider interval indicates greater uncertainty, while a narrower interval suggests higher confidence in the predictions. Interpreting these intervals is paramount for decision-making. Students must understand that forecasting, by nature, involves a degree of uncertainty, and prediction intervals quantify this uncertainty. SPSS's user-friendly interfaces present these intervals alongside forecasted values, allowing students to make informed decisions considering the range of potential outcomes.
Conclusion
In conclusion, mastering time series analysis in SPSS is a valuable skill for students across disciplines. This guide has provided a structured approach to understanding, implementing, and interpreting time series models using SPSS. From importing and preparing data to advanced techniques and model evaluation, students can now navigate the complexities of time-dependent data with confidence. As students engage in assignments requiring time series analysis, the knowledge gained from this guide will serve as a solid foundation. The ability to apply these techniques in SPSS not only enhances academic performance but also equips students with practical skills applicable in various professional settings.
Continuous practice and exploration of diverse datasets will further refine their expertise in time series analysis, fostering a deeper understanding of temporal patterns and trends. In the ever-evolving landscape of data analytics, proficiency in time series analysis using SPSS positions students at the forefront of research and decision-making. As they embark on their academic and professional journeys, the insights gained from this guide will undoubtedly contribute to their success in unraveling the complexities of time-dependent data.