- Introduction to Variance and Covariance
- Importance of Variance and Covariance in Statistical Analysis
- Real-Life Applications of Variance and Covariance
- Understanding the Confidence Interval for Variance
- The Role of Covariance in Regression Analysis
- Positive and Negative Covariance
- Non-Negative Definite Covariance Matrices
- Conclusion
Understanding variance and covariance is fundamental to mastering statistical analysis, which is crucial for completing various homework and excelling in academic performance. These concepts are essential for analyzing data distributions and relationships, which are frequently encountered in statistics homework and homework. By gaining a solid grasp of these concepts, students can enhance their ability to interpret data, identify patterns, and make informed decisions based on their analyses. Additionally, understanding variance and covariance is important for performing more advanced statistical techniques such as regression analysis, hypothesis testing, and multivariate analysis.
We will delve into the relationship between variance and covariance, providing a comprehensive guide to help students tackle similar homework. We'll explore how these concepts are applied in real-world scenarios, discuss their significance in statistical analysis, and provide practical tips for understanding and calculating them. By the end, you should have a clearer understanding of how variance and covariance work together to describe data distributions and relationships. If you ever find yourself stuck, remember that expert help is available to solve your statistics homework and guide you through complex concepts, ensuring that you can confidently complete your homework and excel in your studies.
Introduction to Variance and Covariance
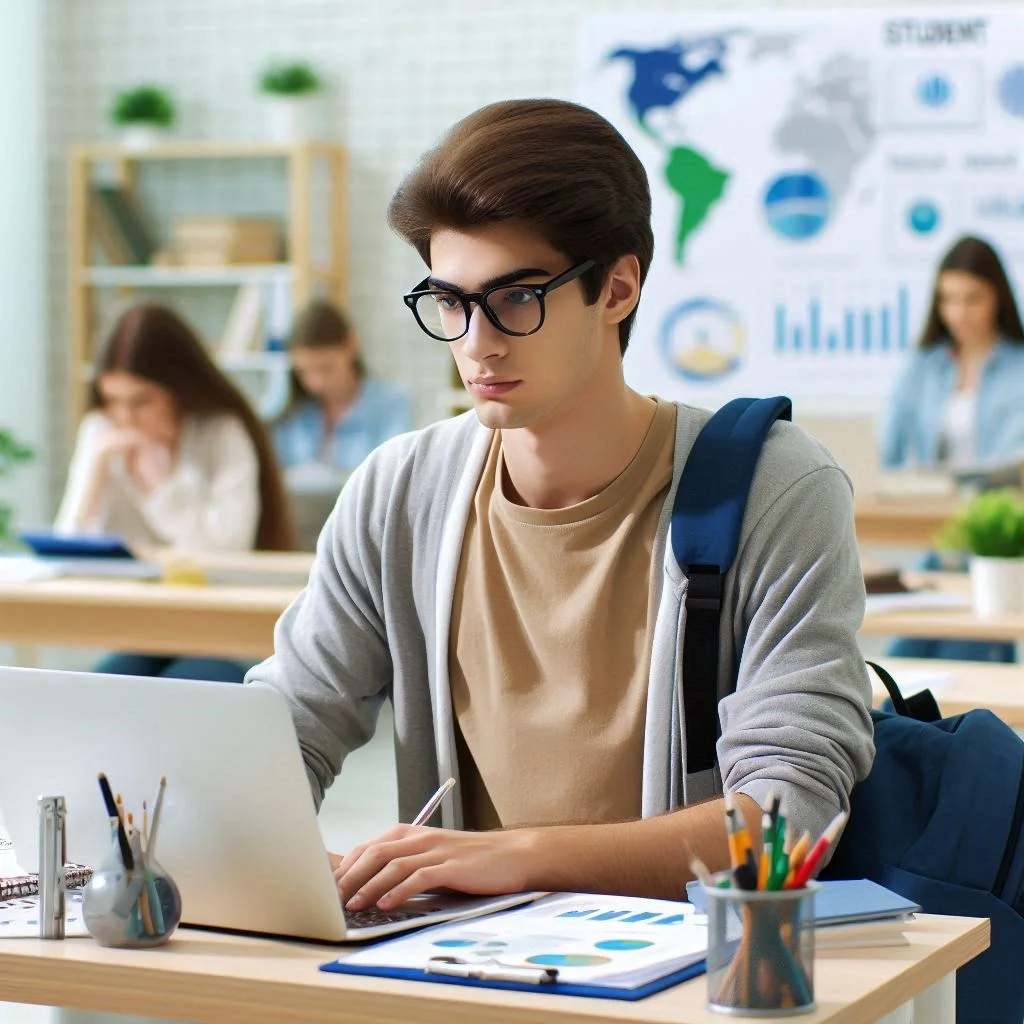
Variance measures the spread of data points in a data set. It tells us how much the data points differ from the mean of the data set. A higher variance indicates that the data points are more spread out from the mean, while a lower variance indicates that they are closer to the mean.
Covariance measures the degree to which two variables change together. If the variables tend to increase together, the covariance is positive. If one variable tends to increase when the other decreases, the covariance is negative. Covariance helps in understanding the relationship between two variables.
Importance of Variance and Covariance in Statistical Analysis
Variance is a critical concept in statistics as it provides insight into the variability within a data set. This measure is essential for understanding the distribution and spread of data, which is fundamental for many statistical analyses, including hypothesis testing, confidence intervals, and regression analysis. When working on homework, recognizing how variance affects your data can significantly improve the accuracy of your results. Understanding variance also allows students to assess risk and reliability in various contexts, such as financial investments, product quality control, and experimental research. By grasping the concept of variance, students can make informed decisions and interpretations based on their data.
Covariance plays a vital role in determining the relationship between two variables. It is especially useful in the fields of finance, economics, and social sciences, where understanding the relationship between variables can lead to better decision-making. For instance, in finance, covariance can help in portfolio management by assessing how different assets move in relation to one another. By understanding the covariance between assets, investors can diversify their portfolios to minimize risk and maximize returns. In economics, covariance helps in studying the relationship between economic indicators, such as GDP growth and unemployment rates, enabling policymakers to formulate effective economic policies.
Real-Life Applications of Variance and Covariance
In real-life scenarios, variance is used in various fields such as finance, quality control, and environmental studies. For example, in finance, variance is used to measure the volatility of stock prices. In quality control, it helps in determining the consistency of product manufacturing. Understanding these applications can help students relate their homework to real-world situations, making the concepts easier to grasp. For instance, in environmental studies, variance is used to analyze the dispersion of pollutants in air and water, helping researchers develop strategies to mitigate environmental hazards. By applying variance in practical contexts, students can appreciate its significance and develop a deeper understanding of its implications.
Covariance is widely used in finance to understand the relationship between different financial assets. It helps in the construction of investment portfolios by assessing how different stocks or assets move together. In the field of economics, covariance is used to study the relationship between different economic indicators, such as inflation and unemployment rates. By analyzing covariance, economists can identify underlying patterns and relationships between variables, providing insights into economic trends and potential policy interventions. Additionally, in the social sciences, covariance is used to explore the relationships between variables such as education level and income, helping researchers understand societal dynamics and inform policy decisions.
Understanding the Confidence Interval for Variance
A confidence interval for variance provides a range of values within which the true variance of the population is expected to lie. This concept is crucial for making inferences about population variability based on sample data. In homework, students often need to calculate and interpret confidence intervals, which helps in understanding the reliability of their data. Confidence intervals for variance are particularly useful in quality control and clinical trials, where it is important to assess the variability and consistency of measurements. By mastering the calculation and interpretation of confidence intervals, students can enhance their analytical skills and apply statistical methods effectively in various contexts.
The Role of Covariance in Regression Analysis
In regression analysis, covariance is used to determine the relationship between the independent and dependent variables. A positive covariance indicates that as the independent variable increases, the dependent variable tends to increase as well, and vice versa. Understanding this relationship is essential for developing accurate regression models in homework. Regression analysis is widely used in various fields such as economics, biology, and social sciences to model and predict outcomes based on observed data. By mastering the use of covariance in regression analysis, students can develop robust models and make informed predictions based on their data.
Positive and Negative Covariance
Covariance can be either positive or negative, indicating the direction of the relationship between two variables. Positive covariance indicates that the variables move in the same direction, while negative covariance indicates that they move in opposite directions. Recognizing the type of covariance is crucial for interpreting data relationships in homework. For example, in finance, positive covariance between assets suggests that they tend to move together, while negative covariance indicates that they move inversely. Understanding positive and negative covariance helps students make informed decisions about diversification and risk management in their investment portfolios.
Non-Negative Definite Covariance Matrices
A covariance matrix is a square matrix that shows the covariance between different variables. For a matrix to be non-negative definite, it must not have any negative eigenvalues. This property ensures that the covariance matrix is mathematically valid and can be used in various multivariate analyses, such as principal component analysis (PCA) and factor analysis. Non-negative definite covariance matrices are essential in fields such as genetics, finance, and machine learning, where multivariate data analysis is crucial for understanding complex relationships and making predictions. By understanding the properties of non-negative definite covariance matrices, students can effectively apply multivariate statistical methods in their homework and research.
Conclusion
Understanding variance and covariance is crucial for anyone studying statistics. These concepts are not only fundamental for academic success but also for real-world applications in various fields. By grasping the relationship between variance and covariance, students can enhance their analytical skills, making them better prepared for complex data analysis tasks. Always remember to approach your homework methodically, and don't shy away from seeking help when needed. With practice and the right resources, you can master these essential statistical tools and apply them effectively in your studies and beyond.