- Foundations of Multivariate Analysis
- Understand Descriptive Statistics:
- Revise Matrix Algebra:
- Types of Multivariate Analysis
- Principal Component Analysis (PCA):
- Factor Analysis:
- Cluster Analysis:
- Multiple Regression Analysis:
- Data Preprocessing
- Data Cleaning:
- Standardization and Normalization:
- Assumptions and Validations
- Multivariate Normality:
- Homoscedasticity:
- Interpretation of Results
- Eigenvalues and Eigenvectors:
- Factor Loadings:
- Software Proficiency
- R or Python:
- Statistical Software (SPSS, SAS, etc.):
- Effective Problem-Solving Strategies for Tackling Multivariate Analysis Homework
- Understand the Problem:
- Break Down the Steps:
- Consult Resources:
- Practice with Datasets:
- Seek Help When Needed:
- Review and Reflect:
- Conclusion
As you delve into the realm of statistics and data analysis, you'll inevitably encounter the fascinating field of multivariate analysis. Multivariate analysis is a statistical technique used to complete your multivariate analysis homework and examine relationships among multiple variables simultaneously. Its applications span various disciplines, including economics, biology, psychology, and more. Whether you're a student embarking on homework or someone seeking a deeper understanding of this intricate subject, this blog post will guide you through the crucial topics you should grasp before tackling multivariate analysis homework and provide effective strategies to solve the problems efficiently.
Foundations of Multivariate Analysis
When embarking on the journey of multivariate analysis, it's crucial to establish a strong foundation in the fundamental concepts that underpin this field. Before delving into the more complex techniques, you need to be well-versed in the following key areas:
Understand Descriptive Statistics:
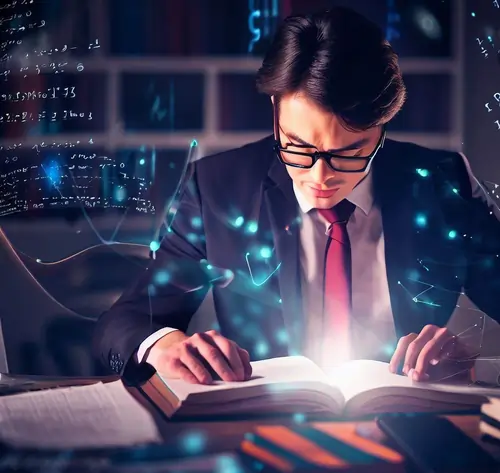
Descriptive statistics serve as the bedrock of any statistical analysis, including multivariate analysis. These statistics allow you to summarize and gain insights from your data. Here are some key components you should be familiar with:
- Mean: The arithmetic average of a set of values. It provides an indication of the central tendency of your data.
- Median: The middle value in a dataset when it's arranged in order. It's a robust measure of central tendency that's not affected by extreme values.
- Mode: The value that appears most frequently in a dataset. It helps identify the most common value or category.
- Standard Deviation: A measure of the dispersion or spread of data points around the mean. It indicates the level of variability in your data.
A solid grasp of these descriptive statistics is essential because they enable you to summarize and understand the distribution of your variables before moving on to more complex analyses.
Revise Matrix Algebra:
Matrix algebra is a fundamental mathematical tool in multivariate analysis. Many multivariate techniques involve manipulating data matrices to uncover relationships and patterns. Here are some key concepts you should review:
- Matrix Multiplication: This operation involves combining rows and columns of matrices to produce a new matrix. In multivariate analysis, this can be used to transform data or perform calculations.
- Transpose:The transpose of a matrix involves flipping its rows and columns. It's often used in operations involving matrices and vectors.
- Determinant: A scalar value associated with a square matrix that has various applications, including in solving systems of linear equations.
Understanding matrix operations is vital because multivariate analysis often involves working with data in a matrix format. Techniques like Principal Component Analysis (PCA) and Linear Discriminant Analysis (LDA) heavily rely on matrix algebra for dimensionality reduction and feature extraction.
Types of Multivariate Analysis
Multivariate analysis is a diverse field that offers a range of techniques to analyze complex relationships among multiple variables. Each technique is designed to address specific types of data and research questions. Let's delve into some of the key techniques within multivariate analysis:
Principal Component Analysis (PCA):
Principal Component Analysis is a dimensionality reduction technique that's particularly useful when dealing with high-dimensional data. The primary goal of PCA is to transform the original variables into a new set of variables called principal components. These components are orthogonal (uncorrelated) and capture the maximum variance present in the data. By doing so, PCA simplifies the data while retaining the essential patterns and relationships. It's often used for tasks like data compression, noise reduction, and identifying important features in datasets with many variables.
Factor Analysis:
Factor Analysis is employed when you have a large number of variables that may be influenced by a smaller number of underlying latent variables or factors. The technique aims to uncover these latent factors that explain the observed correlations among variables. Factor Analysis helps in reducing the complexity of the data by representing the original variables in terms of a smaller number of factors. This is especially useful for understanding the underlying structure of a dataset and identifying key factors that influence the observed relationships.
Cluster Analysis:
Cluster Analysis is utilized to group similar data points together based on their attributes. It's often employed when you want to identify patterns or groupings within a dataset. The technique doesn't require prior knowledge of group homework; instead, it relies on the inherent similarities or dissimilarities between data points. Cluster Analysis is widely used in fields like biology (taxonomy), marketing (customer segmentation), and social sciences (identifying behaviour patterns).
Multiple Regression Analysis:
Multiple Regression Analysis is a versatile technique that examines the relationships between a dependent variable and multiple independent variables. It's used when you want to understand how multiple factors influence an outcome. In essence, it helps you assess the impact of several variables simultaneously, considering their potential interactions and contributions. Multiple Regression is extensively used in fields like economics, social sciences, and business to make predictions, infer causal relationships, and understand the drivers of various outcomes.
Data Preprocessing
Before diving into multivariate analysis techniques, proper data preprocessing is essential to ensure the quality and reliability of your analysis:
Data Cleaning:
Data cleaning involves identifying and rectifying issues in your dataset, such as missing values, outliers, and inconsistencies. Missing values can distort results, while outliers can significantly impact the analysis. Ensuring your data is accurate and complete is crucial for obtaining meaningful insights.
Standardization and Normalization:
Standardization and normalization are techniques used to bring variables to a common scale. This is important because variables might have different measurement units or ranges, which can introduce bias in the analysis. Standardization scales variables to have a mean of 0 and a standard deviation of 1, while normalization scales variables to a range of 0 to 1. These steps ensure that each variable contributes equally to the analysis.
Assumptions and Validations
To ensure the validity of your multivariate analysis results, you need to verify that certain assumptions are met:
Multivariate Normality:
Many multivariate techniques assume that the data follows a multivariate normal distribution. This means that the variables are normally distributed both individually and in combination. Checking for multivariate normality is essential, as violating this assumption can lead to inaccurate results.
Homoscedasticity:
Homoscedasticity refers to the assumption that the variance of errors is consistent across different levels of predictor variables. In other words, the spread of residuals should be relatively constant. Violating this assumption can affect the reliability of regression analysis results.
Interpretation of Results
Interpreting the outcomes of multivariate analysis is a critical step to extract meaningful insights:
Eigenvalues and Eigenvectors:
In techniques like Principal Component Analysis (PCA), eigenvalues and eigenvectors play a crucial role. Eigenvalues indicate the proportion of variance explained by each principal component, while eigenvectors determine the direction and composition of these components. Understanding these concepts helps you grasp the significance of each principal component in reducing data dimensionality.
Factor Loadings:
Factor Analysis involves interpreting factor loadings, which represent the relationships between variables and underlying latent factors. Factor loadings indicate the strength and direction of these relationships. By analyzing factor loadings, you can understand which variables contribute most to each factor and how they are related.
Software Proficiency
To effectively implement multivariate analysis techniques, proficiency in using relevant software is essential:
R or Python:
R and Python are popular programming languages for data analysis. Learning to use libraries like sci-kit-learn in Python or packages like stats in R can simplify the implementation of multivariate techniques and provide greater flexibility in customizing your analyses.
Statistical Software (SPSS, SAS, etc.):
Statistical software such as SPSS and SAS offer user-friendly interfaces for various multivariate analyses. These tools can be especially helpful if you're not comfortable with programming or need to work with large datasets efficiently.
Effective Problem-Solving Strategies for Tackling Multivariate Analysis Homework
Tackling multivariate analysis homework might seem like a formidable task, but employing the right strategies can make it more manageable and even enjoyable. Here are some effective problem-solving strategies to help you navigate your multivariate analysis homework:
Understand the Problem:
Before you start your analysis, take the time to thoroughly understand the problem statement and the specific multivariate technique required. Clarify any doubts or uncertainties you have regarding the objectives and expectations of the analysis.
Break Down the Steps:
Multivariate analysis can involve complex calculations and processes. To prevent feeling overwhelmed, break down the analysis into smaller, manageable steps. This approach not only makes the task seem less daunting but also ensures a systematic approach to solving the problem.
Consult Resources:
Don't hesitate to tap into available resources to enhance your understanding. Textbooks, online tutorials, and academic resources can provide valuable insights into the concepts and techniques you find challenging. Utilize these resources to clarify doubts and deepen your knowledge.
Practice with Datasets:
Theory is important, but practice is essential for mastery. Work with real-world datasets to apply the techniques you're learning. Experiment with different multivariate analysis methods to gain hands-on experience and develop a deeper understanding of their applications.
Seek Help When Needed:
If you encounter difficulties or roadblocks, don't hesitate to seek help. Reach out to your instructors, classmates, or online communities specializing in data analysis. Discussing your challenges with others can lead to fresh perspectives and solutions you might not have considered.
Review and Reflect:
After completing your analysis, take the time to review your results, interpretations, and the steps you took. Reflect on what you've learned from the analysis and consider how you can improve your approach next time. This reflective process enhances your critical thinking skills and helps you build on your knowledge.
Conclusion
Multivariate analysis is a captivating field that offers insights into complex relationships within datasets. Before embarking on multivariate analysis homework, ensure you have a strong foundation in statistics, understand the types of analyses available, and are proficient in data preprocessing and software usage. Following effective problem-solving strategies will not only help you tackle homework efficiently but also enhance your understanding of this versatile analytical approach. As you delve deeper into multivariate analysis, remember that practice, patience, and perseverance are key to mastering this valuable skill.