Claim Your Discount Today
Get 10% off on all Statistics Homework at statisticshomeworkhelp.com! This Spring Semester, use code SHHR10OFF to save on assignments like Probability, Regression Analysis, and Hypothesis Testing. Our experts provide accurate solutions with timely delivery to help you excel. Don’t miss out—this limited-time offer won’t last forever. Claim your discount today!
We Accept
Check Out Our Portfolio of Data Analysis Case Study Homework Solutions
Ever wondered about the caliber of our work? See our portfolio featuring completed data analysis case studies. Each example demonstrates our precision and skill, revealing how we approach and resolve intricate problems. Browse through our solutions to understand how we can transform your case study into a standout submission.
Data Analysis
Data Analysis
Data Analysis
High-Quality Data Analysis Case Study Homework Help That You Can Afford
Our service provides top-notch data analysis case study homework help at affordable prices. Our rates are designed to fit student budgets while ensuring the highest quality. We assess each homework individually to provide a fair quote. Below is a guiding price table for your reference. Contact us for a personalized quote based on your specific needs.
Service Category | Basic | Standard | Advanced | Premium |
---|---|---|---|---|
Simple Data Analysis | $50 | $75 | $100 | $125 |
Intermediate Analysis | $75 | $100 | $125 | $150 |
Complex Analysis | $100 | $125 | $150 | $175 |
Comprehensive Study | $125 | $150 | $175 | $200 |
Urgent Requests | 20% | 20% | 20% | 20% |
Prices vary depending on the complexity of the homework. For an accurate quote, please reach out to us with your homework details.
- Take the Stress Out of Statistics with Professional Data Analysis Case Study Homework Help
- Why Struggle with Tight Deadlines? Let Us Help with Your Data Analysis Case Study Homework
- Hiring a Data Analysis Case Study Homework Doer Is Simpler Than You Think
- Trust Us to Do Your Data Analysis Case Study Homework Every Time
- Reasons To Choose Our Data Analysis Case Study Homework Help
Take the Stress Out of Statistics with Professional Data Analysis Case Study Homework Help
Data analysis case studies involve examining and interpreting complex datasets to uncover patterns, trends, and insights. These homework require a thorough understanding of statistical methods and analytical tools to provide actionable recommendations based on the data. Mastery of these skills is crucial for accurate and effective analysis.
Feeling overwhelmed by your data analysis case study homework? Our reliable statistics homework help service is here to lighten your load. We specialize in providing reliable data analysis case study homework help, ensuring that every homework is completed with accuracy and clarity. With us, you can trust that your case study will be handled by experts who understand the intricacies of data analysis.
We are dedicated to offering top-notch data analysis case study homework assistance. Our team of professionals meticulously analyzes your data, applies the right statistical methods, and delivers results that meet academic standards. Whether it's deciphering complex data sets or presenting your findings, we manage every detail to relieve your stress and help you succeed.
Choose us for dependable and efficient support. As the only reliable company online providing this level of expertise, we guarantee a stress-free experience. Let us take care of your data analysis needs so you can focus on other important aspects of your studies. Quality work and timely results are what you can expect from our professional service.
Why Struggle with Tight Deadlines? Let Us Help with Your Data Analysis Case Study Homework
Facing tight deadlines for your data analysis case study homework can be stressful. Don’t let time constraints compromise your academic performance. Our service provides swift help with data analysis case study homework, ensuring that you meet your deadlines without sacrificing the quality of your work. Our team of experts is equipped to handle urgent homework efficiently.
When you choose us, you benefit from professional assistance with data analysis case study homework from highly skilled professionals. Our experts are not only fast but also meticulous in their work, guaranteeing precise results under pressure. We understand the importance of delivering work on time, and we have strategies in place to make sure your case study is completed promptly.
To ensure we meet even the tightest deadlines, our team:
- Prioritizes tasks to focus on urgent homework first
- Utilizes efficient data analysis tools to speed up the process
- Maintains clear communication to update you on progress
- Adheres to strict quality checks to ensure accuracy
- Offers flexible support to adjust to any last-minute changes
Hiring a Data Analysis Case Study Homework Doer Is Simpler Than You Think
If you need help with your data analysis case study homework but think it’s too complicated to arrange, think again. Hiring our expert services is a straightforward process that saves you time and effort. We are here to simplify the task of getting professional assistance for your homework.
Our team of skilled data analysis case study homework helpers is just a few steps away. Begin by submitting your homework details through our easy-to-use order form. Based on the information you provide, we’ll give you a fair and transparent price quote. Once you make the initial payment, our data analysis case study homework writers will immediately begin working on your case study.
Here’s how the process works:
- Submit your homework details through our order form
- Receive a competitive price quote tailored to your homework
- Make the initial payment to start the work
- Get notified when your homework is completed
- Pay the remaining balance and download your completed work
With these simple steps, you can access expert help quickly and efficiently. Enjoy a hassle-free experience and high-quality results without the usual stress.
Trust Us to Do Your Data Analysis Case Study Homework Every Time
When you need someone reliable to do your data analysis case study homework, trust us to deliver outstanding results consistently. Our reputation for excellence in handling homework means you can rely on us every time. We understand the importance of meeting deadlines and providing high-quality work that exceeds expectations.
Our goal is to ensure that every homework we handle is done with precision and care. When you ask us to write your data analysis case study homework, you’re choosing a service known for its consistent performance. We’ve built our reputation on delivering top-notch results and maintaining high standards.
Here’s why you can trust us:
- Delivered homework punctually 99.95% of the time
- Achieved good grades 96% of the time, surpassing competitors
- Provided tailored solutions to meet specific homework requirements
- Maintained clear communication throughout the process
- Used advanced tools and methods for accurate analysis
- Offered competitive pricing without compromising quality
Choose us for reliable, high-quality data analysis case study help every time.
Reasons To Choose Our Data Analysis Case Study Homework Help
When you need reliable data analysis case study homework help, selecting the right service is crucial. Our team at statistics homework helper stands out for its commitment to providing exceptional support and quality. Here’s why choosing us can make a difference in your academic experience.
- Transparency in Pricing: We offer clear and upfront pricing with no hidden fees, ensuring you know exactly what you’re paying for. Our straightforward approach helps you make informed decisions without any surprises.
- Payment Security: Your financial transactions are protected through secure payment systems, giving you peace of mind throughout the process. We prioritize your security and confidentiality in all dealings.
- Track Record: Our impressive track record speaks for itself. We consistently deliver high-quality results and maintain a strong reputation for reliability in handling complex homework.
- Flexibility: We adapt to your needs, offering flexible solutions tailored to your specific requirements. Whether you have tight deadlines or unique homework specifications, we can accommodate your needs.
- Response Time: Our quick response time ensures that your questions and concerns are addressed promptly. We are committed to providing timely support and keeping you updated on your homework’s progress.
Choose us for dependable, transparent, and efficient data analysis case study homework help.
Find Answers to Your Data Analysis Case Study Questions in Our Blogs
Unlock valuable insights with our blogs dedicated to data analysis case studies. Our posts offer detailed explanations, updates, and tips designed to help you excel in your coursework. Whether you're looking to enhance your knowledge or stay current with best practices, our expertly crafted content provides the answers and guidance you need.
Real Experiences: See How We’ve Helped Students with Data Analysis Case Study Homework
Discover firsthand accounts from students who have benefited from our expert assistance with data analysis case studies. These reviews showcase how our dedicated support has made a difference in their academic journeys, highlighting our commitment to quality and reliability.
Introducing Our Top-Rated Statistics Data Analysis Case Study Homework Experts
Meet our team of exceptional experts, each bringing a wealth of experience and specialized knowledge to provide top-quality data analysis case study homework help. Our professionals come from diverse academic backgrounds and have completed numerous homework, ensuring that you receive the best assistance possible. Discover more about our experts below.
.webp)
Loretta Walk
Ph.D. in Environmental Statistics
🇬🇧 United Kingdom
Loretta Walk, from Vancouver, has a Ph.D. in Environmental Statistics from the University of British Columbia and has completed over 900 spatial statistics homework. Her expertise includes handling complex spatial data sets and providing clear, actionable insights. Dr. Martinez’s ability to simplify intricate spatial statistics concepts makes her an excellent choice for both academic and professional projects.
.webp)
Dr. Ryan Patel
Master's degree in Spatial Data Science
🇦🇺 Australia
Dr. Ryan Patel, based in Sydney, holds a Master's degree in Spatial Data Science from the University of Sydney. With over 750 completed homework, Dr. Patel has a strong background in using advanced spatial statistics tools and techniques. His practical experience and academic knowledge ensure that he provides precise and actionable insights for various spatial statistics projects.
.webp)
Dr. Julia Zhang
Master's degree in Geostatistics
🇨🇦 Canada
Dr. Julia Zhang is a spatial data analyst with a Master's degree in Geostatistics from the University of Toronto and has successfully completed over 600 homework. Her background in both academic research and practical applications equips her with a comprehensive understanding of spatial data analysis methods. Dr. Zhang is known for her meticulous approach and ability to handle a diverse range of spatial statistics tasks.
.webp)
Dr. Henry Brooks
Ph.D. in Spatial Statistics
🇦🇺 Australia
Dr. Henry Brooks holds a Ph.D. in Spatial Statistics from the University of Edinburgh and has over 10 years of experience in the field. With a remarkable track record of over 800 completed spatial statistics homework, Dr. Brooks is well-versed in both theoretical and practical aspects of spatial analysis. His expertise spans various software tools and techniques, making him a valuable asset for complex and nuanced projects.
.webp)
Zoe Martinez
Master’s degree in Data Analytics
🇦🇺 Australia
Based in Australia, Zoe Martinez holds a Master’s degree in Data Analytics and has completed more than 500 homework in fraud detection and prevention. Zoe is known for her expertise in applying data analytics to detect and prevent fraud. Her homework focus on practical solutions and effective strategies for combating fraud, providing students with a robust understanding of the field.
.webp)
Liam Patel
Masters in Statistics
🇦🇺 Australia
From the UK, Liam Patel holds a Master’s degree in Statistics and has completed over 600 homework in fraud detection and prevention. Liam is recognized for his expertise in financial fraud detection and fraud prevention technologies. His homework is characterized by their clear, actionable recommendations and adherence to current industry standards.
.webp)
Isabella Wang
Ph.D. in Information Security
🇦🇺 Australia
Isabella Wang, from Canada, has a Ph.D. in Information Security with a specialization in fraud detection and prevention. With over 650 homework completed, Isabella excels in areas such as fraud risk assessment and anti-fraud strategies. Her work integrates theoretical concepts with practical examples, ensuring a thorough understanding of fraud prevention practices.
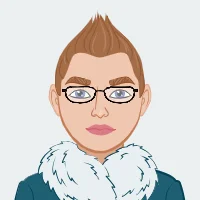
Ethan White
Master’s degree in Cybersecurity
🇦🇺 Australia
Based in the USA, Ethan White holds a Master’s degree in Cybersecurity and has completed over 750 homework in fraud detection and prevention. Ethan's expertise includes financial fraud detection techniques, anti-fraud strategies, and the implementation of fraud prevention technologies. His work is known for its depth and practical application, providing students with actionable insights into modern fraud prevention methods.
.webp)
Dr. Debra Smith
Ph.D. in Statistics
🇺🇸 United States
Dr. Debra Smith holds a PhD in Applied Statistics from the University of Toronto and has completed around 900 homework. Known for her detailed and accurate statistical analyses, Dr. Smith is proficient in tackling both standard and complex problems in categorical data analysis. Her dedication to quality and timely delivery makes her a top expert in the field.
.webp)
Dr. Richard Jones
Ph.D. in Statistics
🇺🇸 United States
Dr. Richard Jones earned his PhD in Statistical Science from the National University of Singapore and has worked on over 500 homework. His broad expertise spans various statistical methods, from basic categorical data analysis to advanced modeling techniques. His precise and detailed work makes him a great choice for high-quality solutions.
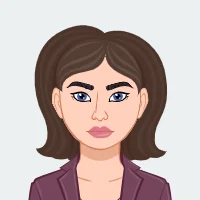
Dr. Kristen Meier
Ph.D. in Statistics
🇺🇸 United States
Dr. Kristen Meier, a PhD graduate from Emporia State University, has handled over 650 homework. With a strong background in both theoretical and applied statistics, Dr. Meier excels at providing clear, actionable insights through detailed analyses. Her expertise helps students achieve top grades with tailored, comprehensive solutions.
.webp)
Dr. Stephen Howe
Ph.D. in Statistics
🇺🇸 United States
Dr. Stephen Howe holds a PhD in Statistics from the University of Cambridge and has completed over 750 homework. His extensive experience in both academic and practical statistics ensures high-quality and insightful solutions for complex problems. Dr. Howe' deep understanding of statistical tools guarantees accurate results for every homework.
.webp)
Mia Roberts
Ph.D. in Statistics
🇺🇸 United States
Mia Roberts, a graduate of the University of Melbourne with a degree in Applied Mathematics, has worked on over 500 distributional analysis homework. Her analytical prowess and keen insight into statistical models make her a standout expert. Mia’s dedication to her work and her ability to break down complex statistical concepts into understandable terms ensure top-notch assistance for her students.
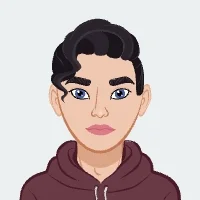
Liam Brooks
Masters in Statistics
🇺🇸 United States
Liam Brooks is a professional statistician with a Master’s degree from the University of Chicago. He has completed over 900 distributional analysis homework and is praised for his problem-solving skills and efficiency. Liam’s expertise lies in applying statistical theories to practical problems, making him adept at handling diverse homework requirements. His thorough approach ensures that each homework is handled with precision and depth.
.webp)
Jenna Collins
Ph.D. in Statistics
🇺🇸 United States
Jenna Collins is a Ph.D. candidate in Statistical Science from Harvard University with a focus on distributional analysis. With over 600 homework completed, Jenna has a robust grasp of various statistical distributions and their applications. Her analytical skills and commitment to academic excellence make her a reliable choice for students seeking expert help. Jenna's background in research and data analysis enhances her ability to provide high-quality, insightful solutions.
.webp)
Alex Turner
Masters in Statistics
🇺🇸 United States
Alex Turner holds a Master’s degree in Statistics from the University of London and has over 8 years of experience in the field. He has successfully completed more than 750 homework on distributional analysis, showcasing his deep understanding of statistical methods and analytical techniques. Alex is known for his meticulous attention to detail and his ability to simplify complex concepts for students. His extensive experience ensures that he delivers accurate and comprehensive solutions tailored to each student's needs.
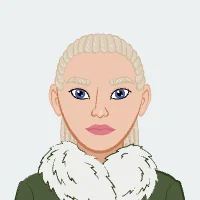
Liam Wilson
Ph.D. in Statistics
🇺🇸 United States
Liam Wilson is an expert in statistical analysis with a Ph.D. from the Australian National University and has handled over 900 exploratory data analysis homework. Liam's strong analytical skills and deep understanding of statistical theory allow him to perform detailed and high-quality analyses. His work is characterized by precision and clarity, making him a reliable choice for students seeking top-notch exploratory data analysis support.
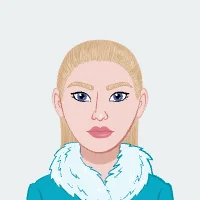
Fiona Carter
Master's degree in Statistics
🇦🇺 Australia
Fiona Carter, with a Master's degree in Statistics from the University of Melbourne, has completed over 500 exploratory data analysis homework. Her focus is on using statistical methods to uncover insights and trends in diverse datasets. Fiona’s expertise in data exploration, visualization, and interpretation ensures that students receive accurate and insightful analysis for their homework. She is known for her clear and effective communication of complex data concepts.
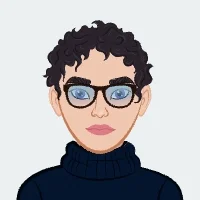
James O'Connor
Master's degree in Data Science
🇬🇧 United Kingdom
James O'Connor is a seasoned data analyst with a Master's degree in Data Science from Stanford University. With more than 600 completed homework, James excels in providing comprehensive exploratory data analysis solutions tailored to student needs. His expertise lies in analyzing large datasets and extracting meaningful patterns using modern data analytics tools and methods. James is praised for his analytical skills and ability to present complex data in an understandable format.
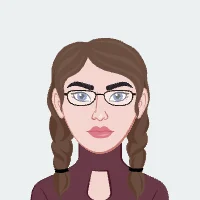
Dr. Alice Mitchell
Ph.D. in Statistics
🇦🇺 Australia
Dr. Alice Mitchell holds a Ph.D. in Statistics from the University of Cambridge and has completed over 750 exploratory data analysis homework. Her extensive academic background and practical experience make her an exceptional choice for handling complex data analysis tasks. Alice specializes in transforming raw data into actionable insights using various statistical techniques and advanced visualization tools. She is known for her meticulous attention to detail and ability to deliver high-quality work promptly.
Related Topics
Frequently Asked Questions
Explore our FAQ section to find clear answers to common queries. Whether you're curious about our pricing, turnaround times, or confidentiality, we've covered all the essential details. Our goal is to provide transparent and straightforward information to help you understand how we can assist with your data analysis case study needs.