Claim Your Discount Today
Celebrate Christmas and New Year with www.statisticshomeworkhelper.com! Enjoy a holiday 15% discount on all statistics homework, ensuring expert assistance at an unbeatable value. Let our team simplify your studies while you enjoy the holiday spirit. Don’t miss this seasonal offer to save money and excel academically. Start the New Year strong—grab this deal now!
We Accept
Free Assignment Samples Available for Your Reference
Explore our free assignment samples to see the quality of multiple linear regression equations homework help we offer. At statisticshomeworkhelper.com, our multiple linear regression equations homework experts provide exceptional help with multiple linear regression equations homework. Check out these examples to understand how our statistics homework help can elevate your assignments. Get a glimpse of our expertise and approach to delivering top-notch academic support.
SPSS
Statistical Models
R Programming
Statistical Models
SPSS
Affordable Multiple Linear Regression Equations Homework Help Tailored Just for You
Get affordable multiple linear regression equations homework help tailored specifically for you at statisticshomeworkhelper.com. Our multiple linear regression equations homework experts provide personalized help with multiple linear regression equations homework to fit your needs. Enjoy quality statistics homework help without overspending. Our services are designed to offer exceptional support while respecting your budget. Trust us for expert assistance that meets your academic goals affordably.
Description | Price Range |
---|---|
Basic Multiple Linear Regression Equations Homework Help | $15 - $30 |
Intermediate Assistance with Regression Models | $35 - $60 |
Advanced Analysis and Solutions for Regression Problems | $65 - $100 |
Complex Regression Projects and Extensive Analysis | $120 - $200+ |
Priority Service for Any Level of Homework | Additional 50% |
Consultation on Regression Equations and Analysis | $20 - $50 per hour |
- Multiple Linear Regression Equations Homework Help
- Why Investing is Essential in Online Multiple Linear Regression Equations Homework Help?
- Why Our Multiple Linear Regression Equations Homework Help Service is The Best Choice for Students?
- Varied Multiple Linear Regression Equations Homework Topics We Expertly Manage
- Easy Steps to Reach Our Multiple Linear Regression Equations Homework Help Team
- Different Payment Methods Available for Easy Transactions
Multiple Linear Regression Equations Homework Help
At statisticshomeworkhelper.com, we offer comprehensive multiple linear regression equations homework help tailored to your academic needs. Our dedicated multiple linear regression equations homework experts are equipped with the skills and knowledge to tackle a wide range of issues in your assignments.
Whether you need assistance with complex models, variable selection, or data normalization, our experts provide precise and effective help with multiple linear regression equations homework. We understand the importance of accurate and timely statistics homework help, which is why our team is committed to delivering top-quality solutions.
Our services ensure that you receive not only the correct answers but also detailed explanations to enhance your understanding. With a focus on clarity and precision, we aim to support you in achieving excellent grades. Trust our experienced team to guide you through every step of your multiple linear regression equations homework.
From initial consultation to final submission, we are here to assist you with expert advice and support. If you are worried about who will do my multiple linear regression equations homework? Choose us for reliable and effective assistance in your multiple linear regression equations homework.
Why Investing is Essential in Online Multiple Linear Regression Equations Homework Help?
Investing in online multiple linear regression equations homework help is crucial due to the complexity and challenges involved in these assignments. With high academic expectations and tight deadlines, obtaining expert help with multiple linear regression equations homework ensures accuracy. Multiple linear regression equations homework experts provide valuable insights and solutions that address the intricate aspects of your homework.
At statisticshomeworkhelper.com, we offer specialized statistics homework help to meet these demands, ensuring that your work meets the highest standards of precision and quality. This investment not only enhances your academic performance but also alleviates the stress of meeting stringent deadlines. If you are worried about who will do my multiple linear regression equations homework? Rest assured, our proficient multiple linear regression equations homework help team is ready to help you every step of the way!
- Complexity of Concepts: The advanced nature of multiple linear regression equations can be overwhelming, making it challenging to grasp essential concepts without professional guidance.
- High Academic Expectations: Achieving high marks requires precise and accurate solutions, which can be difficult to attain without the assistance of a multiple linear regression equations homework expert.
- Tight Deadlines: Meeting strict deadlines adds pressure, and managing complex regression problems under time constraints can lead to errors or incomplete work.
- Risk of Inaccurate Solutions: Incorrect analysis or misunderstanding of multiple linear regression models can result in significant academic penalties, emphasizing the need for reliable help with multiple linear regression equations homework.
- Lack of Resources: Limited access to high-quality resources and support can hinder your ability to complete assignments correctly, making professional assistance essential for overcoming these obstacles.
- Fear of Poor Grades: Without expert multiple linear regression equations homework help, there’s a high risk of receiving subpar grades due to complex problems and intricate details.
Why Our Multiple Linear Regression Equations Homework Help Service is The Best Choice for Students?
Our Multiple Linear Regression Equations Homework Help service stands out for its exceptional features and benefits. At statisticshomeworkhelper.com, we connect students with top multiple linear regression equations homework experts who provide thorough and accurate help with multiple linear regression equations homework. Our experts are skilled in tackling complex problems, including analyzing data sets, calculating confidence intervals, and understanding multiple predictors while keeping variables held constant. We also offer expertise in interpreting dependent variables and constructing ANOVA tables.
With our reliable statistics homework help online, we ensure that academic standards and deadlines are met with precision. With a focus on clarity, precision, and personalized support, our service helps students excel and achieve their academic goals efficiently. If you are worried about who will do my multiple linear regression equations homework? Choose us for reliable, expert multiple linear regression equations homework assistance that makes a difference in your academic performance.
- Expert Guidance: Connect with top multiple linear regression equations homework experts who provide precise and detailed solutions tailored to your specific assignment needs.
- Timely Delivery: Receive prompt multiple linear regression equations homework help that meets your deadlines, ensuring you stay on track with your academic schedule and avoid last-minute stress.
- High-Quality Solutions: Our service guarantees high standards in help with multiple linear regression equations homework, offering accurate and comprehensive answers to complex regression problems.
- 24/7 Support: Access round-the-clock assistance from our team, ensuring you get timely help and answers to any questions regarding your statistics homework help at any time.
- Customized Assistance: Enjoy personalized support that addresses your unique requirements and challenges in multiple linear regression equations homework, enhancing your understanding and performance.
- Confidential Service: Trust in our secure and confidential approach to providing multiple linear regression equations homework help, ensuring your personal information and academic work remain protected.
Varied Multiple Linear Regression Equations Homework Topics We Expertly Manage
At statistics homework helper, we expertly handle a wide range of multiple linear regression equations homework topics. Our experienced multiple linear regression equations homework experts provide precise help with linear regression homework, covering diverse aspects from model formulation to advanced analysis. Whether you need assistance with variable selection, data normalization, or interaction terms, our professional statistics homework help ensures comprehensive support tailored to your needs. We also offer guidance on simple linear regressions, addressing the response variable, predictor variables, test statistic, and methods that minimize the sum of the squared residuals.
Our experts are proficient in using statistical software and can handle questions about independent variables, degree of freedom, and crafting a simple linear regression model with one predictor. If you are worried about who will do my multiple linear regression equations homework? Trust us to deliver high-quality solutions that meet academic standards and help you excel in your multiple linear regression equations homework.
- Model Formulation: We provide expert assistance in designing and formulating multiple linear regression models, ensuring your equations are correctly specified and suited to your data.
- Variable Selection: Our experts help you identify and select the most relevant variables for your regression analysis, enhancing model accuracy and performance for precise results.
- Data Normalization: Receive guidance on standardizing your data to improve model reliability and interpretability, making your multiple linear regression equations more effective and robust.
- Interaction Terms: We assist in incorporating and interpreting interaction terms in your regression models, allowing you to explore and understand complex relationships between variables.
- Residual Analysis: Our service includes thorough residual analysis to check for model assumptions and identify potential issues, ensuring your regression results are valid and reliable.
- Model Validation: Get support in validating your multiple linear regression models through various techniques, ensuring that your results are accurate and meet academic standards.
Easy Steps to Reach Our Multiple Linear Regression Equations Homework Help Team
Getting in touch with our team for multiple linear regression equations homework help is simple. Start by submitting your homework details through our easy-to-use online form. Our multiple linear regression equations homework experts will review your requirements and get back to you with a personalized plan.
For additional help with multiple linear regression equations homework, you can also reach out directly through our live chat option. We offer comprehensive statistics homework help to ensure you receive accurate and timely support. If you are worried about who will do my multiple linear regression equations homework? Trust statisticshomeworkhelper.com to get the expertise you need to complete your multiple linear regression equations homework with ease.
- Submit Homework Requirements: Provide your cumulative relative frequency homework details through our website or email, including specific questions and guidelines for accurate and tailored assistance.
- Receive a Quote: After submission, receive a quote for our help with cumulative relative frequency homework based on the complexity and scope of your assignment.
- Assign an Expert: We match you with a cumulative relative frequency homework expert who specializes in your topic to ensure high-quality and precise support.
- Expert Review: The assigned expert reviews your requirements and begins working on the assignment, focusing on delivering accurate and comprehensive statistics homework help.
- Receive Completed Work: Obtain the completed cumulative relative frequency homework solutions by the agreed deadline, with all required calculations and explanations clearly provided.
- Request Revisions: If needed, request revisions from the expert to ensure the final work meets your expectations and academic requirements for cumulative relative frequency homework help.
Different Payment Methods Available for Easy Transactions
At statisticshomeworkhelper.com, we offer a variety of payment methods to ensure easy and secure transactions for our multiple linear regression equations homework help services. To make the process seamless, you can choose from several convenient options.
We accept Credit/Debit Card payments, including American Express, for quick and straightforward transactions. If you prefer, you can use PayPal for its secure and efficient service. Bank Transfer is also available for those who prefer traditional payment methods.
Additionally, Zelle and UnionPay are supported for added flexibility in managing your payments. Our goal is to make acquiring help with multiple linear regression equations homework as hassle-free as possible. With these diverse payment methods, you can easily access support from our multiple linear regression equations homework experts and benefit from our top-notch statistics homework help.
We ensure that all transactions are processed securely and efficiently, providing a smooth experience for our valued clients. Choose the payment method that best suits your needs and let us help you achieve academic success.
Our Well-Crafted Blogs on Multiple Linear Regression Equations Homework
Explore our well-crafted blogs on multiple linear regression equations homework to gain valuable insights. Our multiple linear regression equations homework helper provides expert tips and solutions, offering the best help with multiple linear regression equations homework. Discover effective strategies and techniques to complete your assignments with confidence.
Impressive Client Feedback on Our Multiple Linear Regression Equations Homework Help
Our clients rave about the exceptional multiple linear regression equations homework help they’ve received. With top-notch help with multiple linear regression equations homework from our skilled multiple linear regression equations homework helper, students achieve outstanding results. The positive feedback highlights our commitment to delivering clear, accurate, and timely assistance, ensuring top performance on every assignment.
Introducing Our Dedicated Multiple Linear Regression Equations Homework Helpers
Meet our dedicated team of experts offering top-tier multiple linear regression equations homework help. Our skilled multiple linear regression equations homework helpers provide exceptional help with multiple linear regression equations homework, ensuring precise and effective solutions for all your assignments. Trust our specialists to deliver quality assistance and help you excel in your coursework.
Dr. Chloe Evans
PhD in Statistical Analysis
🇬🇧 United Kingdom
Dr. Chloe Evans, with a PhD in Statistical Analysis from Simon Fraser University, has over 12 years of experience and has completed over 675 homework. Her profound knowledge and hands-on experience in statistical modeling and analysis make her an invaluable resource. Dr. Evans is dedicated to providing precise and effective solutions tailored to each student’s needs.
MR. Ankit Patel
Master’s in Applied Statistics
🇮🇳 India
MR. Ankit Patel earned his Master’s in Applied Statistics from the Indian Institute of Technology, Roorkee. Over the past 10 years, he has completed over 500 homework, bringing a wealth of experience to his role. MR. Patel’s expertise in practical and theoretical aspects of statistics ensures that students receive comprehensive and accurate assistance.
Professor Reuben O'Connor
PhD in Statistics
🇬🇧 United Kingdom
Professor Reuben O'Connor holds a PhD in Statistics from the University of Leicester and has a robust track record with over 400 completed homework. With 15 years in academia, his extensive knowledge covers various statistical methods and applications. Professor O'Connor is known for his meticulous approach and commitment to delivering high-quality, reliable solutions.
Dr. Yumi Nakamura
PhD in Statistical Science
🇦🇺 Australia
Dr. Yumi Nakamura, with a PhD in Statistical Science from Kyushu University, has over 15 years of experience in statistical modeling. She has completed more than 350 homework, showcasing her ability to handle a wide range of complex problems. Dr. Nakamura’s deep expertise and dedication ensure that each project is tackled with precision and insightful analysis.
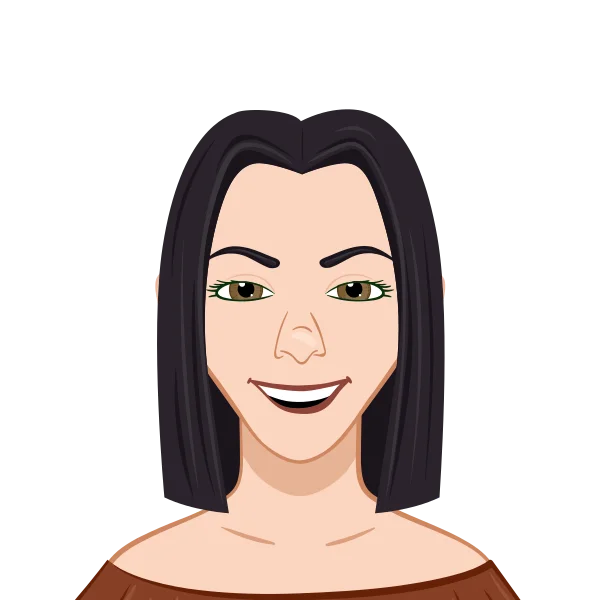
Dr. Ava Smith
master’s degree in Applied Statistics
🇺🇸 United States
Dr. Ava Smith, hailing from the USA, is an accomplished statistician with a focus on advanced regression and correlation analysis. She earned her Ph.D. in Statistics from Stanford University and has completed over 950 homework. Dr. Smith's expertise includes a range of regression techniques and correlation methods, and she is dedicated to helping students achieve academic success through thorough, high-quality assistance.
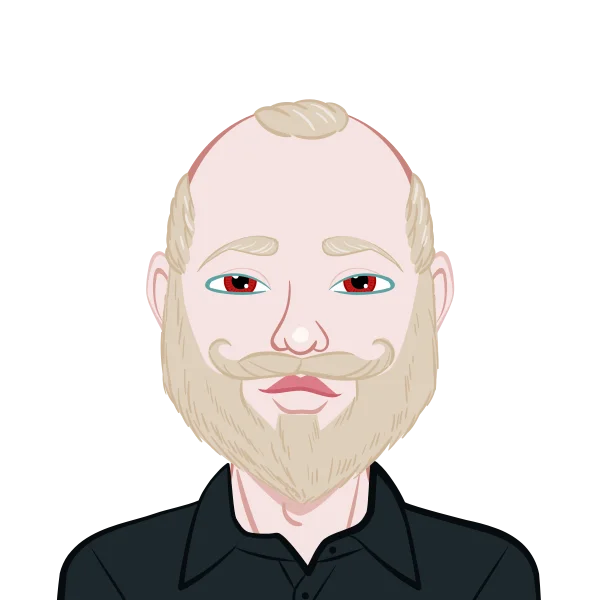
Professor Daniel Clarke
Ph.D. in Statistics
🇦🇺 Australia
From Australia, Professor Daniel Clarke brings a wealth of knowledge to our team with his background in statistics and data analysis. With a Ph.D. in Statistics from the Australian National University, he has handled over 800 homework. His expertise lies in providing comprehensive solutions and explanations for various regression models and correlation techniques, ensuring clarity and precision.
.webp)
Dr. Jessica Martin
Master’s degree in Applied Statistics
🇨🇦 Canada
Dr. Jessica Martin is a leading statistician from Canada with extensive experience in handling Regression and Correlation homework. She holds a Master’s degree in Applied Statistics from the University of Toronto and has successfully completed more than 600 homework. Dr. Martin is known for her meticulous approach and ability to simplify complex statistical concepts, making them accessible to students at all levels.
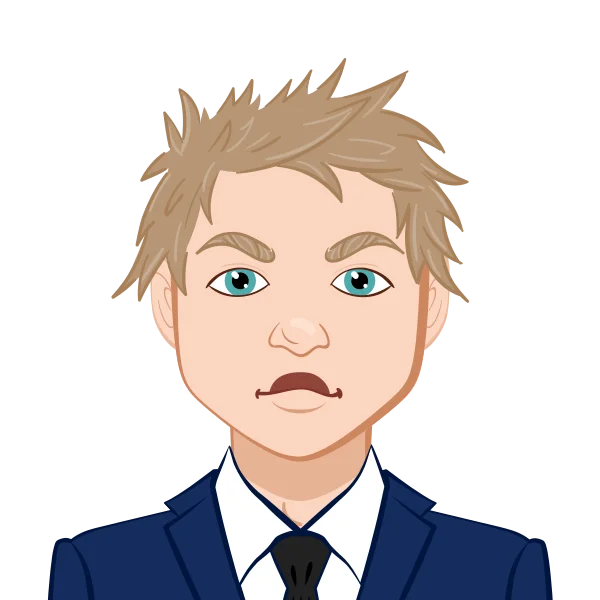
Dr. Liam Williams
Ph.D. in Statistics
🇬🇧 United Kingdom
Based in the UK, Dr. Liam Williams is a seasoned expert in statistics with a focus on Regression and Correlation analysis. He holds a Ph.D. in Statistics from the University of Cambridge and has completed over 750 homework. Dr. Williams combines his academic knowledge with practical experience to provide detailed and insightful solutions for complex statistical problems. His approach ensures that students not only get accurate results but also gain a deeper understanding of the concepts involved.
.webp)
Dr. Ramon McDonell
Ph.D. in Statistics
🇦🇺 Australia
Dr. Ramon McDonell is an experienced statistician with a PhD from the University of Sydney. With over 500 completed homework in Generalized Linear Modeling, he excels in both theoretical and applied statistics. Dr. O'Connor’s expertise extends to complex modeling techniques and software applications, providing comprehensive support for students’ academic needs.
.webp)
Dr. Aisha Ibrahim
Ph.D. in Statistics
🇬🇧 United Kingdom
Dr. Aisha Ibrahim holds a PhD in Statistics from the University of Malaya and has extensive experience in teaching and research. She has completed around 700 Generalized Linear Modeling homework, focusing on ensuring clarity and precision in statistical analysis. Her approach combines rigorous methodology with practical insights to help students excel in their coursework.
.webp)
Dr. James Bennett
PhD in Applied Mathematics
🇨🇦 Canada
Dr. James Bennett is a data scientist with a PhD in Applied Mathematics from Cape Breton. With over 600 completed Generalized Linear Modeling homework, he specializes in applying advanced statistical techniques to real-world problems. His background in both academia and industry allows him to provide thorough and practical solutions tailored to students' needs.
.webp)
Dr. Jeanine Woods
Ph.D. in Statistics
🇺🇸 United States
Dr. Jeanine Woods holds a PhD in Statistics from the University of Western Illinois and has over a decade of experience in data analysis and statistical modeling. She has completed over 800 Generalized Linear Modeling homework, offering expertise in both theoretical and practical aspects of the subject. Dr. Clarke is well-versed in various statistical software and methodologies, ensuring accurate and insightful results for complex homework.
.webp)
Dr. Oliver James
PhD in Statistics
🇬🇧 United Kingdom
Dr. Oliver James earned his BSc in Mathematics from the University of Warwick. With over 1,600 completed homework and 10 years of experience, he excels in delivering insightful and practical solutions in predictive regression modeling.
.webp)
Ms. Priya Patel
Master’s degree in Applied Statistics
🇬🇧 United Kingdom
Ms. Priya Patel holds a Master’s degree in Applied Statistics from the University of Warwick. With more than 850 completed homework and 9 years of teaching experience, she focuses on making complex regression models accessible and understandable.
.webp)
Professor Mark Thompson
MSc in Statistics
🇨🇦 Canada
Professor Mark Thompson earned his MSc in Statistics from the University of Toronto. He has completed over 1,200 homework and has been teaching for 14 years. His expertise lies in bridging theoretical concepts with practical applications to enhance student understanding.
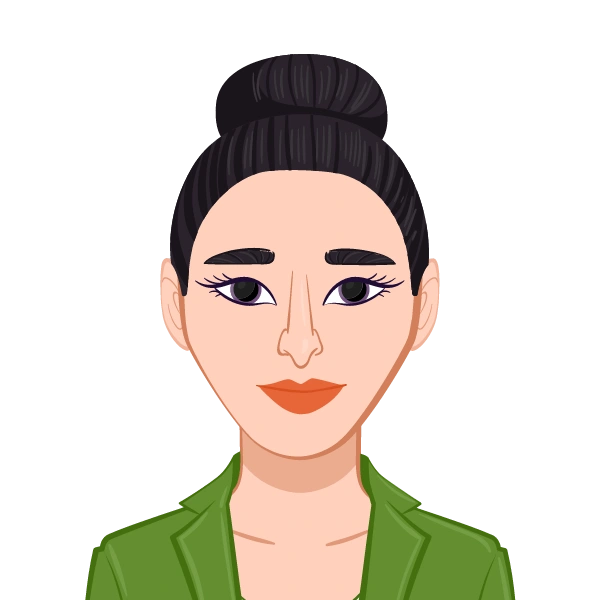
Dr. Lisa Bennett
PhD in Data Science
🇺🇸 United States
Dr. Lisa Bennett holds a PhD in Data Science from Stanford University. With over 900 completed homework, she has more than 11 years of experience in academia and research. Her specialty includes complex predictive modeling techniques and providing clear, actionable insights for students.
.webp)
Mr. Rajesh Iyer
Master’s in Statistics
🇬🇧 United Kingdom
Mr. Iyer holds a Master’s in Statistics from University of St Mark & St John. He has successfully completed over 320 homework tasks. With 7 years of experience, his practical knowledge of real-world applications of regression makes him a favorite among students seeking clear, concise solutions.
.webp)
Dr. Mei-Ling Zhang
Ph.D. in Mathematics
🇨🇳 China
Dr. Zhang earned her Ph.D. in Mathematics from Xi’an Jiaotong University and has over 12 years of experience in statistical research and homework assistance. With more than 380 completed homework, Dr. Zhang is adept at simplifying complex concepts and delivering top-quality work on tight deadlines.
.webp)
Professor Daniel Ainsley
Ph.D. in Applied Statistics
🇬🇧 United Kingdom
A distinguished professor with a Ph.D. in Applied Statistics from University of St Mark & St John, Professor Ainsley has guided over 520 students to success. He specializes in crafting accurate regression models and providing step-by-step solutions for students tackling challenging homework.
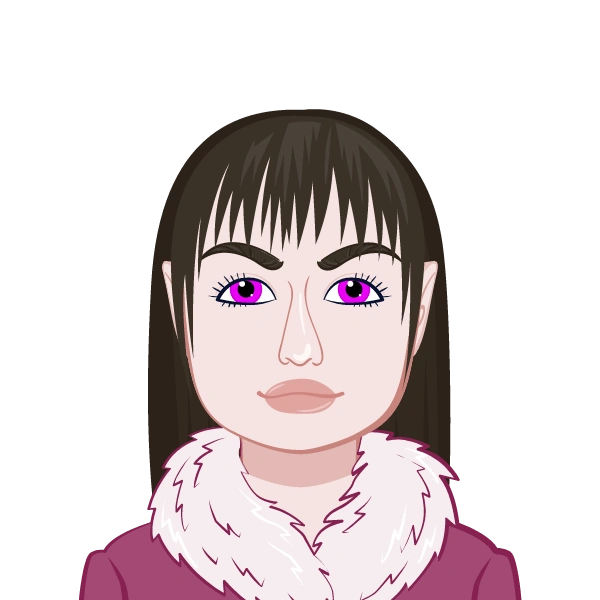
Dr. Evelyn Harper
Ph.D. in Statistics
🇺🇸 United States
With a Ph.D. in Statistics from The College of Wooster, Dr. Harper has over 10 years of experience in regression analysis. She has completed more than 450 homework with precision and depth, helping students excel academically. Dr. Harper’s expertise spans various fields, particularly in linear and multiple regression topics.
Our Popular Services
Frequently Asked Questions
Check out our Frequently Asked Questions (FAQs) for answers about our multiple linear regression equations homework help. Learn more about how our multiple linear regression equations homework helpers can provide the help with multiple linear regression equations homework you need. Our FAQs cover everything from the scope of assistance to how you can benefit from our expert services.