Claim Your Discount Today
Get 10% off on all Statistics homework at statisticshomeworkhelp.com! Whether it’s Probability, Regression Analysis, or Hypothesis Testing, our experts are ready to help you excel. Don’t miss out—grab this offer today! Our dedicated team ensures accurate solutions and timely delivery, boosting your grades and confidence. Hurry, this limited-time discount won’t last forever!
We Accept
Sample Non-Parametric Analysis Homework Completed by Our Experts
We understand the importance of seeing examples of the work we provide. In our sample section, you can review completed Non-Parametric Analysis homework to get a sense of the quality and thoroughness of our solutions. These samples cover various topics within Non-Parametric Analysis, showcasing the structured and insightful work our experts consistently deliver. Browse our samples to see how we approach different statistical challenges and provide tailored solutions for each homework.
Statistical Tests
SPSS
Statistical Tests
Statistical Tests
Statistical Tests
Affordable Non-Parametric Analysis Homework Help Service Tailored to Your Budget
We believe that every student deserves access to high-quality Non-Parametric Analysis homework help without straining their finances. Our pricing structure is designed to offer affordability while maintaining the highest standards of service. By tailoring our rates to meet the diverse budgets of students, we ensure that you receive value for your money. Below is a sample table showcasing the price ranges for various types of Non-Parametric Analysis homework, reflecting our commitment to both affordability and excellence.
Homework Type | Basic Rate (USD) | Standard Rate (USD) | Premium Rate (USD) |
---|---|---|---|
Problem Sets | $20 - $40 | $30 - $50 | $40 - $60 |
Essay/Report Writing | $25 - $45 | $35 - $55 | $45 - $65 |
Research Papers | $30 - $50 | $40 - $60 | $50 - $70 |
Case Studies | $25 - $45 | $35 - $55 | $45 - $65 |
Exam Preparation | $35 - $55 | $45 - $65 | $55 - $75 |
- What is Non-parametric Analysis?
- Why Students Choose Our Reliable Help with Non-Parametric Analysis Homework
- How Our Seasoned Non-Parametric Analysis Homework Helpers Complete Your Project
- What Are the Qualifications of the Experts Who Will Do My Non-Parametric Analysis Homework?
What is Non-parametric Analysis?
Non-parametric analysis refers to statistical methods that do not assume a specific distribution for the data. Unlike parametric methods, which rely on assumptions about the underlying population parameters, non-parametric methods are more flexible and can be applied to data that doesn’t fit standard distribution models. These methods are particularly useful when dealing with ordinal data, small sample sizes, or data that does not meet the assumptions required for parametric tests.
At Statistics Homework Helper, the key concepts catered to by our non-parametric analysis homework help include:
- Rank-Based Methods: Non-parametric tests often use ranks rather than actual data values. For instance, the Wilcoxon signed-rank test and the Mann-Whitney U test are used to compare medians and assess differences between groups without assuming normal distribution.
- Chi-Square Tests: These tests are used to determine if there is a significant association between categorical variables. The Chi-square test for independence assesses whether two categorical variables are independent, while the Chi-square goodness-of-fit test checks if a sample data matches a population with a specific distribution.
- Kruskal-Wallis Test: This is a non-parametric alternative to one-way ANOVA. It is used when comparing more than two independent groups to determine if there are significant differences in their distributions.
- Spearman’s Rank Correlation: This method assesses the strength and direction of association between two ranked variables. Unlike Pearson’s correlation, it does not require the assumption of linear relationships or normality.
- Bootstrapping: A resampling technique that allows estimation of the sampling distribution of an estimator by repeatedly resampling with replacement from the data. It is useful for assessing the variability of statistical estimates.
Understanding these concepts allows our non-parametric analysis homework helpers at StatisticsHomeworkHelper.com to handle a variety of data types and scenarios where parametric assumptions are not appropriate, making non-parametric methods a versatile tool in statistical analysis.
Why Students Choose Our Reliable Help with Non-Parametric Analysis Homework
Completing non-parametric analysis homework can be a complex and challenging task for many students. The dedicated team at StatisticsHomeworkHelper.com is here to make the process smoother and more manageable. By choosing our professional statistics homework help, you gain access to a range of benefits designed to improve your academic performance and deepen your understanding of this statistical method.
- Expert Assistance: Our team consists of seasoned professionals with extensive knowledge in non-parametric analysis. They are equipped to handle a variety of complex topics and provide you with clear, accurate solutions. Whether you’re struggling with hypothesis testing or rank-based methods, our experts are here to assist you with precision.
- Customized Solutions: Every student’s needs are unique. That’s why we offer tailored solutions that cater specifically to your individual requirements. From personalized homework help to exam preparation, we create customized homework that align with your learning style and academic goals.
- Timely Delivery: Meeting deadlines is crucial in academic settings. Our team ensures that your non-parametric analysis homework are completed on time, allowing you to submit your work without stress. Whether you need last-minute help or ongoing support throughout the semester, we provide timely assistance to meet your needs.
- Enhanced Academic Performance: With our help, you can significantly boost your performance in non-parametric analysis. Our comprehensive approach, including detailed explanations and practice problems, helps you master the concepts and apply them effectively in your homework and exams.
- Increased Confidence: Mastering non-parametric analysis concepts can be daunting, but with our support, you'll build the confidence needed to tackle challenging problems with ease. Our expert assistance helps you approach your homework with assurance and clarity.
- Comprehensive Learning Experience: Beyond just completing your homework, we aim to enhance your overall learning experience. Our experts provide additional resources, sample problems, and insightful explanations to deepen your understanding of non-parametric analysis principles.
Contact StatisticsHomeworkHelper.com today and see how our expert non-parametric analysis homework help can transform your academic journey.
How Our Seasoned Non-Parametric Analysis Homework Helpers Complete Your Project
When it comes to non-parametric analysis, precision and expertise are crucial. Our team of dedicated non-parametric analysis homework helpers is well-versed in statistical methods that do not rely on parametric assumptions. Here’s a detailed look at how our experts approach and complete your non-parametric analysis homework to ensure you receive high-quality, accurate, and timely solutions.
- Understanding the Homework Requirements: Our non-parametric analysis homework helpers begin by thoroughly reviewing the homework instructions and requirements. This step ensures that they grasp the specific needs of your homework, including any unique guidelines or objectives outlined by your instructor.
- Research and Data Collection: Experts in non-parametric analysis then gather the necessary data and resources. This may involve collecting data sets, reviewing academic literature, or utilizing statistical software to analyze data. Accurate data collection is critical for performing valid non-parametric tests and deriving meaningful results.
- Choosing the Appropriate Non-Parametric Test: Depending on the nature of the data and the research questions, our specialists select the most suitable non-parametric test. Whether it’s the Mann-Whitney U test, Kruskal-Wallis test, or Spearman’s rank correlation, the choice of test is crucial for accurate analysis and interpretation.
- Performing the Analysis: With the test selected, our experts conduct the non-parametric analysis. This includes running statistical calculations, interpreting the results, and ensuring that the analysis aligns with the homework’s objectives. Precision in this step is essential to provide accurate and reliable findings.
- Interpreting Results and Drawing Conclusions: After performing the analysis, our experts interpret the results and draw meaningful conclusions based on the data. This step involves explaining the significance of the findings in the context of the homework’s research question or hypothesis.
- Crafting a Comprehensive Report: The final stage involves compiling a detailed report that includes the analysis process, results, interpretations, and any relevant visualizations or charts. Our experts ensure that the report is well-structured, clear, and meets academic standards.
- Revisions and Final Checks: Before submitting the completed homework, our non-parametric analysis homework helpers conduct thorough revisions and quality checks. This ensures that the homework is free from errors, adheres to all guidelines, and is of the highest quality.
By following these meticulous steps, our experts in non-parametric analysis deliver homework solutions that not only meet but exceed your academic expectations. Reach out to us to experience the precision and expertise of our non-parametric analysis homework helpers.
What Are the Qualifications of the Experts Who Will Do My Non-Parametric Analysis Homework?
When you ask us, “Please do my non-parametric analysis homework,” you’re entrusting it to a team of highly qualified professionals. Our experts are selected based on their extensive knowledge, practical experience, and academic credentials, ensuring that your homework is completed to the highest standard. Here’s a closer look at the qualifications that make our team exceptional:
- Subject-Matter Experts: Our non-parametric analysis homework helpers are subject-matter experts with in-depth understanding of statistical methods that do not rely on parametric assumptions. They possess specialized knowledge in non-parametric techniques, which allows them to tackle complex problems and deliver precise results.
- Former Professors and Lecturers: Many of our experts have a background as former professors and lecturers in statistics and related fields. Their teaching experience provides them with a deep understanding of non-parametric analysis concepts and pedagogical skills, ensuring they can explain and apply these concepts effectively in your homework.
- Industry Practitioners: Our team includes industry practitioners who apply non-parametric analysis in real-world scenarios. Their practical experience with data analysis, statistical software, and industry-specific applications enhances their ability to provide relevant and accurate solutions for your homework.
- PhD-Qualified Statisticians: We have PhD-qualified statisticians who bring advanced expertise in statistical theory and methods. Their extensive academic and research background equips them with the skills to handle complex non-parametric analysis tasks and ensure that your homework is not only correct but also academically rigorous.
By leveraging the diverse qualifications of our team at StatisticsHomeworkHelper.com, we ensure that every aspect of your non-parametric analysis homework is handled with the utmost expertise and precision. Trust us to deliver top-quality work and help you achieve academic success.
Read Our Well-Researched Blog Articles on Non-Parametric Analysis
Our blog is a rich resource for students looking to deepen their understanding of Non-Parametric Analysis. From articles on the latest developments in statistical methods to detailed explanations of concepts such as the Kruskal-Wallis test and the Mann-Whitney U test, our blog covers a wide range of topics. All content is written by our in-house experts, providing insightful and practical knowledge that can enhance your studies and application of Non-Parametric Analysis.
Over 1.2K Reviews with an Average Rating of 4.9/5
We are proud to have received glowing feedback from the students who have used our Non-Parametric Analysis homework help services. With more than 1,200 reviews and an impressive average rating of 4.9/5, our testimonials highlight the quality, reliability, and effectiveness of our services. These reviews reflect our unwavering commitment to helping students achieve their academic goals, and we continue to strive for excellence in every homework we complete.
100+ PhD Qualified Non-Parametric Analysis Homework Experts
Our team of experts comprises highly qualified statisticians and data analysis specialists, each with a deep understanding of Non-Parametric Analysis. Equipped with advanced degrees and a proven track record in academia, they bring expert-level knowledge to every homework. Our experts are dedicated to delivering top-tier solutions that meet your specific requirements, ensuring you receive homework that align with the highest academic standards. From hypothesis testing to rank-based methods, our team ensures comprehensive support for your Non-Parametric Analysis homework.
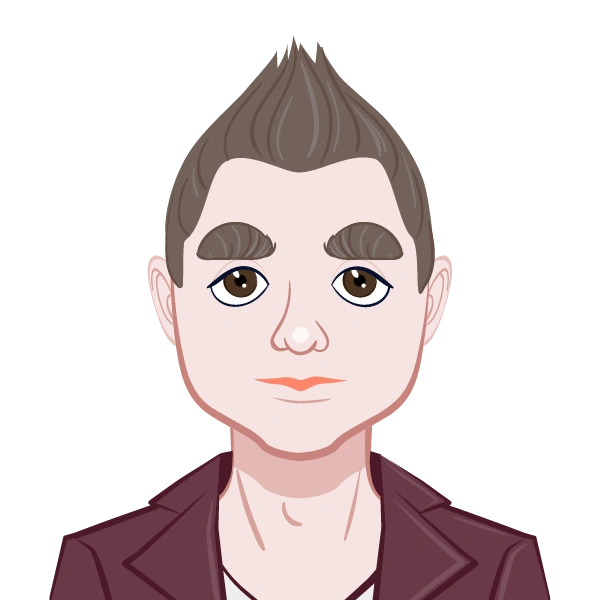
Mason Dawson
Ph.D. in Statistics
🇩🇪 Germany
Mason Dawson, a Ph.D. graduate from TU Dortmund University, offers 9 years of expertise in one-sample z-test homework. His focus on Normal Distribution Analysis and Two-Tailed Test Procedures ensures comprehensive support for students navigating their assignments.
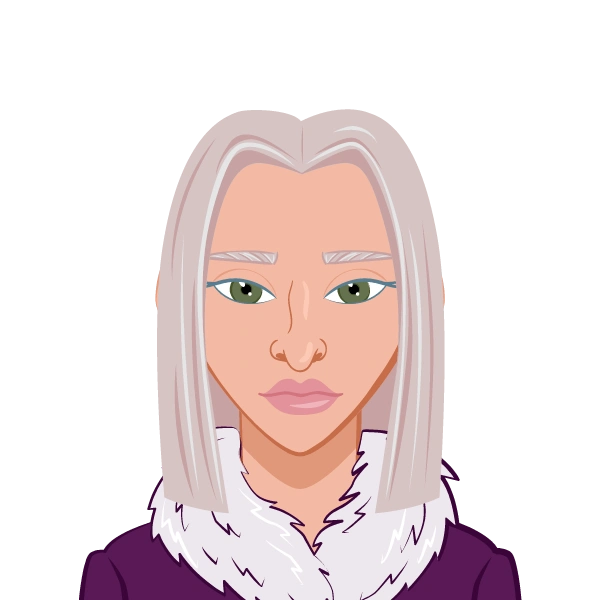
Olivia Read
Ph.D. in Statistics
🇺🇸 United States
Olivia Read, with a Ph.D. from University of Maryland, College Park, brings 15 years of experience to one-sample z-test homework. Her specialization in Standard Error Calculation and Error Margin Analysis offers students detailed support and precise solutions.
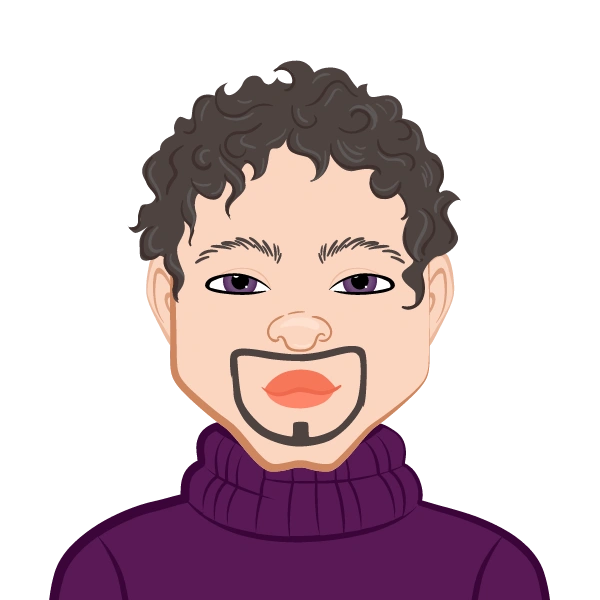
Ellis Abbott
Ph.D. in Statistics
🇩🇪 Germany
Ellis Abbott, a Ph.D. graduate from RWTH Aachen University, has 18 years of experience in one-sample z-test homework. His expertise includes Hypothesis Testing and Critical Value Analysis, ensuring students receive high-quality guidance on complex statistical topics.
.webp)
Ethan Brooks
Master's degree in Statistics
🇺🇸 United States
Ethan Brooks, a seasoned statistician with a Master’s degree from Stanford University, has completed over 950 homework related to statistical analysis. His extensive knowledge of ANOVA and other statistical methods allows him to deliver top-quality homework solutions in record time. Ethan’s work is driven by a passion for problem-solving and ensuring that each student receives solutions that not only meet their academic needs but also improve their understanding of statistical principles.
.webp)
Isla Montgomery
Master’s degree in Biostatistics
🇬🇧 United Kingdom
Isla Montgomery holds a Master’s degree in Biostatistics from Imperial College London and has successfully completed over 800 homework orders in the field of statistics. With a focus on Analysis of Variance and related statistical methods, Isla’s expertise lies in providing clear, well-structured solutions that meet academic standards. Her ability to break down complex statistical concepts and deliver them in an understandable format has made her a favorite among students seeking reliable and timely help with their ANOVA homework.
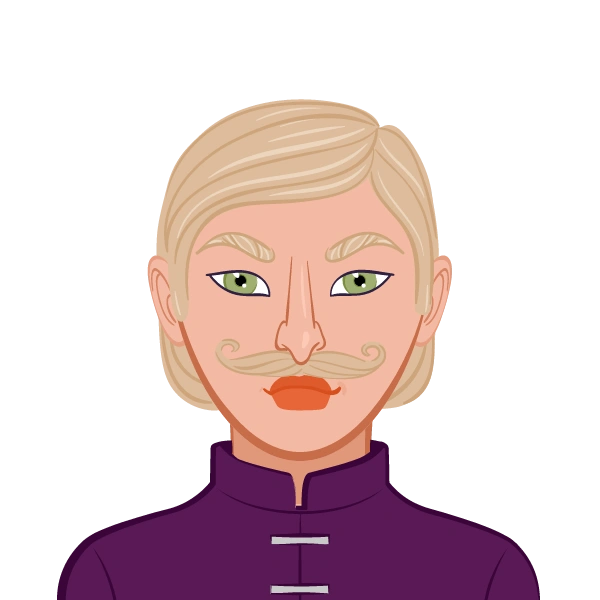
Liam Prescott
PhD holder in Applied Mathematics
🇦🇺 Australia
Liam Prescott, a PhD holder in Applied Mathematics from the University of Melbourne, has completed over 900 homework homework in statistical analysis. With more than a decade of experience, Liam specializes in advanced topics such as variance analysis and regression models. His academic background and practical experience equip him to tackle even the most complex homework. His exceptional communication skills and attention to detail ensure that students not only receive accurate solutions but also understand the underlying concepts of their homework.
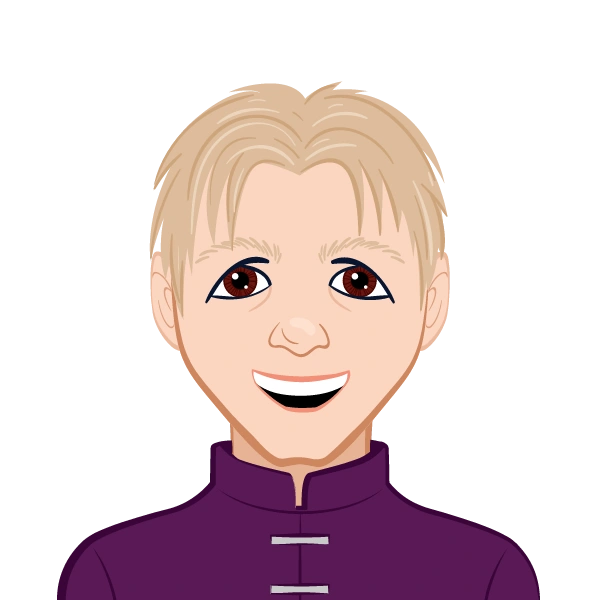
Daniela Curtis
Master's degree in Statistics
🇬🇧 United Kingdom
Daniela Curtis holds a Master's degree in Statistics from the University of Edinburgh, specializing in statistical analysis and research methods. With over 8 years of experience in academia and 750+ completed homework orders, Daniela is known for delivering precise and thorough solutions in a wide range of statistical topics. She has a deep understanding of Analysis of Variance (ANOVA) and other statistical techniques, making her a highly sought-after expert for homework that require accuracy and in-depth analysis. Her strong analytical skills and commitment to quality make her a top choice for students.
.webp)
Benjamin Turner
Master’s in Data Science
🇦🇺 Australia
Benjamin is a highly qualified statistician with a Master’s in Data Science from the University of Melbourne. He has completed over 600 homework focusing on statistical analysis, particularly in the area of Goodness of Fit tests. Benjamin's methodical approach and keen eye for detail allow him to solve even the most challenging problems quickly and accurately. His expertise in both theoretical and applied statistics ensures that students receive top-notch solutions that meet academic standards.
.webp)
Ayesha Khan
Master’s in Statistics
🇨🇦 Canada
Ayesha earned her Master’s in Statistics from the University of Toronto and has successfully completed over 700 homework for students globally. Known for her clear and concise approach to solving Goodness of Fit problems, Ayesha focuses on providing detailed explanations so students can master the concepts behind their homework. Her thorough understanding of statistical distributions and hypothesis testing has helped students achieve excellent grades.
.webp)
Natalie Carter
PhD in Data Science
🇺🇸 United States
Natalie has a PhD in Applied Statistics from the University of California, Berkeley, and has worked on more than 950 homework projects in various fields of statistics. With a passion for teaching and a talent for simplifying complicated statistical models, Natalie is known for helping students understand the intricate details of Goodness of Fit tests. Her extensive experience with statistical software and methods makes her a valuable resource for students needing help with data-driven homework.
.webp)
James Riley
Master’s degree in Statistics
🇬🇧 United Kingdom
James holds a Master’s degree in Statistics from the University of Edinburgh and has completed over 800 homework related to statistical analysis. With his deep knowledge of statistical methods, James specializes in helping students tackle complex problems involving Goodness of Fit tests. His ability to break down difficult concepts into understandable steps has made him a favorite among students. James is committed to delivering accurate, high-quality solutions within tight deadlines, ensuring students not only meet but exceed their academic goals.
.webp)
Liam Roberts
Master's degree in Statistics
🇺🇸 United States
Liam Roberts is a statistical consultant with a strong academic background, including a Master's degree in Statistics from the University of Oxford. Having successfully completed over 550 correlation analysis homework tasks, he brings a wealth of knowledge and practical insight to his tutoring. Liam’s focus on precision and his commitment to providing detailed, well-explained solutions ensure that students receive high-quality support.
.webp)
Hannah Miller
Master’s degree in Quantitative Analysis
🇦🇺 Australia
Hannah Miller is an experienced data analyst with a Master’s degree in Quantitative Analysis from the University of Sydney. With over 700 completed homework, she excels in providing thorough and clear explanations of correlation analysis concepts. Hannah’s practical approach and her ability to relate theoretical concepts to real-world data make her a valuable resource for students needing assistance with their homework.

Nathaniel Scott
Master's degree in Data Science
🇺🇸 United States
Nathaniel Scott holds a Master's degree in Statistics from Stanford University and has been providing expert help for over 600 correlation analysis homework. His background in data science and statistics, combined with his practical experience, allows him to deliver insightful and precise solutions. Nathaniel’s dedication to detail and his ability to simplify complex statistical methods have earned him a reputation for excellence among his students.
.webp)
Jessica Adams
Ph.D. in Applied Statistics
🇦🇺 Australia
Jessica Adams is a seasoned statistician with a Ph.D. in Applied Statistics from the University of Melbourne. She has completed over 800 correlation analysis homework, showcasing her expertise in both fundamental and advanced topics. Jessica’s deep understanding of statistical methods and her ability to break down complex concepts make her an exceptional tutor for students seeking clarity and accuracy. Her approach is meticulous, ensuring each homework is tailored to meet specific academic requirements.
.webp)
Lily Fernandez
Master's degree in Data Science
🇺🇸 United States
Based in the USA, Lily Fernandez holds a Master's degree in Data Science from Stanford University and has completed over 950 homework. She is renowned for her deep knowledge of Non-Parametric methods and her ability to handle complex data structures. Lily's work is known for its accuracy and insightful conclusions.
.webp)
David Thompson
PhD in Biostatistics
🇦🇺 Australia
David Thompson, an Australian expert, has a PhD in Biostatistics from the University of Sydney. With over 850 homework completed, David excels in advanced Non-Parametric Analysis techniques. His homework are detailed, focusing on both the theoretical aspects and practical implementation using statistical software.
.webp)
Samantha Chen
Master's degree in Applied Statistics
🇸🇬 Singapore
Samantha Chen, from Singapore, holds a Master's degree in Applied Statistics from the National University of Singapore. With over 700 completed homework, she specializes in applying Non-Parametric techniques to real-world data. Samantha's approach is practical, and her homework are known for their clarity and detailed explanations.
.webp)
Ethan Collins
PhD in Statistics
🇬🇧 United Kingdom
Based in the UK, Ethan Collins holds a PhD in Statistics from the University of Edinburgh. Having completed over 900 homework, Ethan is known for his expertise in Non-Parametric Analysis and statistical modeling. His analytical skills and precise interpretation of data make him a top choice for handling complex Non-Parametric homework.
.webp)
Dr. Lucas White
Ph.D. in Quantitative Methods
🇬🇧 United Kingdom
Dr. Lucas White earned his Ph.D. in Quantitative Methods from Stanford University and has completed over 850 homework assignments. With 9 years of experience in statistical research and teaching, Dr. White excels in conducting one sample T-tests with precision and clarity, ensuring students receive comprehensive and well-explained solutions.
Related Topics
Frequently Asked Questions
If you have any questions about our Non-Parametric Analysis homework help, be sure to check out our FAQ section. We cover commonly asked questions to provide clarity and transparency about our services. Should you have additional queries, our live chat support is available 24/7 to assist you and ensure a seamless experience throughout your journey with us.